Segmentation of Pericardial Adipose Tissue in CMR Images: A Benchmark Dataset MRPEAT and a Triple-Stage Network 3SUnet
IEEE transactions on medical imaging(2023)
摘要
Increased pericardial adipose tissue (PEAT) is associated with a series of cardiovascular diseases (CVDs) and metabolic syndromes. Quantitative analysis of PEAT by means of image segmentation is of great significance. Although cardiovascular magnetic resonance (CMR) has been utilized as a routine method for non-invasive and non-radioactive CVD diagnosis, segmentation of PEAT in CMR images is challenging and laborious. In practice, no public CMR datasets are available for validating PEAT automatic segmentation. Therefore, we first release a benchmark CMR dataset, MRPEAT, which consists of cardiac short axis (SA) CMR images from 50 hypertrophic cardiomyopathy (HCM), 50 acute myocardial infarction (AMI), and 50 normal control (NC) subjects. We then propose a deep learning model, named as 3SUnet, to segment PEAT on MRPEAT to tackle the challenges that PEAT is relatively small and diverse and its intensities are hard to distinguish from the background. The 3SUnet is a triple-stage network, of which the backbones are all Unet. One Unet is used to extract a region of interest (ROI) for any given image with ventricles and PEAT being contained completely using a multi-task continual learning strategy. Another Unet is adopted to segment PEAT in ROI-cropped images. The third Unet is utilized to refine PEAT segmentation accuracy guided by an image adaptive probability map. The proposed model is qualitatively and quantitatively compared with the state-of-the-art models on the dataset. We obtain the PEAT segmentation results through 3SUnet, assess the robustness of 3SUnet under different pathological conditions, and identify the imaging indications of PEAT in CVDs.
The dataset and all source codes are available at https://dflag-neu.github.io/member/csz/research/
.
更多查看译文
关键词
pericardial adipose tissue,benchmark dataset mrpeat,cmr images,adipose tissue,triple-stage
AI 理解论文
溯源树
样例
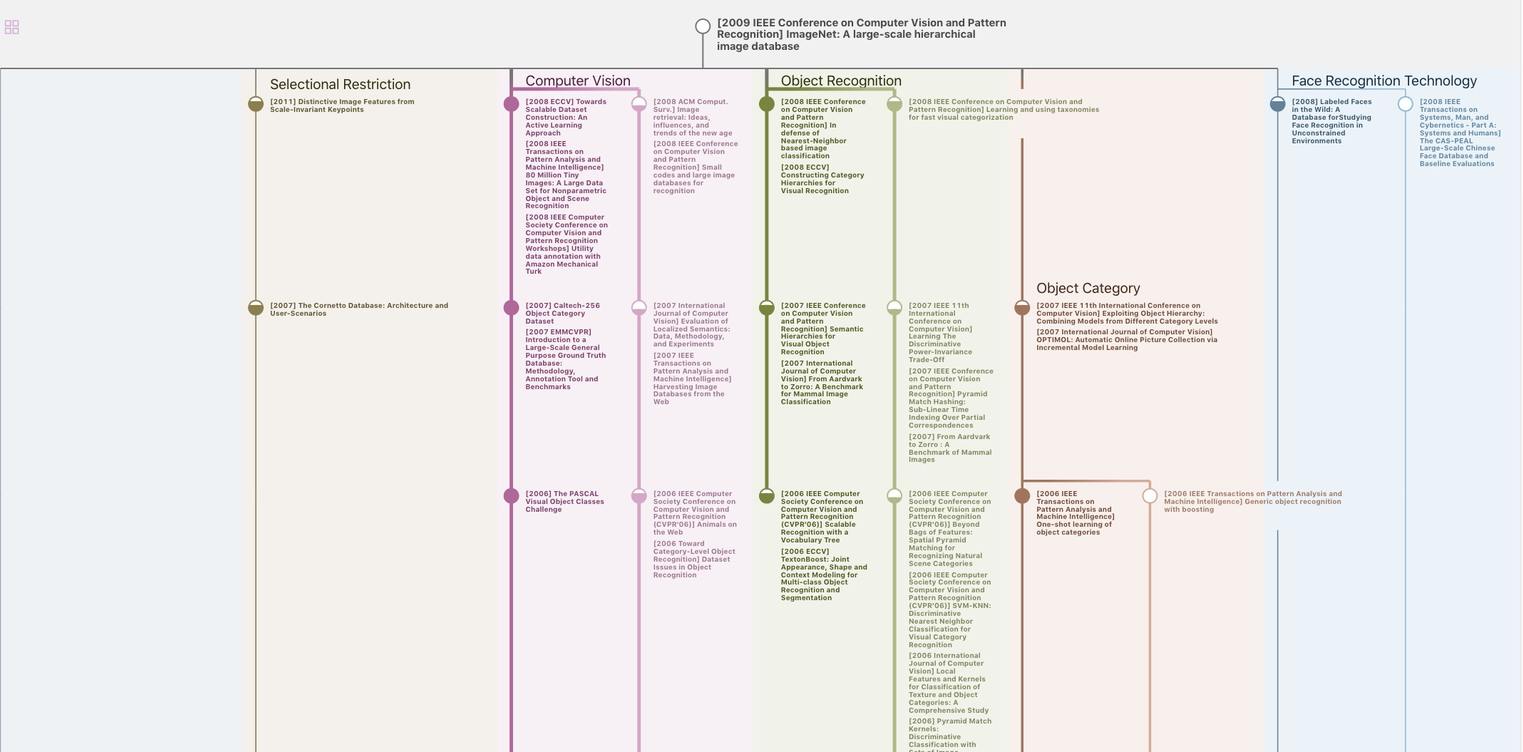
生成溯源树,研究论文发展脉络
Chat Paper
正在生成论文摘要