Automatic gross tumor segmentation of canine head and neck cancer using deep learning and cross-species transfer learning
FRONTIERS IN VETERINARY SCIENCE(2023)
摘要
BackgroundRadiotherapy (RT) is increasingly being used on dogs with spontaneous head and neck cancer (HNC), which account for a large percentage of veterinary patients treated with RT. Accurate definition of the gross tumor volume (GTV) is a vital part of RT planning, ensuring adequate dose coverage of the tumor while limiting the radiation dose to surrounding tissues. Currently the GTV is contoured manually in medical images, which is a time-consuming and challenging task. PurposeThe purpose of this study was to evaluate the applicability of deep learning-based automatic segmentation of the GTV in canine patients with HNC. Materials and methodsContrast-enhanced computed tomography (CT) images and corresponding manual GTV contours of 36 canine HNC patients and 197 human HNC patients were included. A 3D U-Net convolutional neural network (CNN) was trained to automatically segment the GTV in canine patients using two main approaches: (i) training models from scratch based solely on canine CT images, and (ii) using cross-species transfer learning where models were pretrained on CT images of human patients and then fine-tuned on CT images of canine patients. For the canine patients, automatic segmentations were assessed using the Dice similarity coefficient (Dice), the positive predictive value, the true positive rate, and surface distance metrics, calculated from a four-fold cross-validation strategy where each fold was used as a validation set and test set once in independent model runs. ResultsCNN models trained from scratch on canine data or by using transfer learning obtained mean test set Dice scores of 0.55 and 0.52, respectively, indicating acceptable auto-segmentations, similar to the mean Dice performances reported for CT-based automatic segmentation in human HNC studies. Automatic segmentation of nasal cavity tumors appeared particularly promising, resulting in mean test set Dice scores of 0.69 for both approaches. ConclusionIn conclusion, deep learning-based automatic segmentation of the GTV using CNN models based on canine data only or a cross-species transfer learning approach shows promise for future application in RT of canine HNC patients.
更多查看译文
关键词
canine head and neck cancer,dogs,radiotherapy,automatic target volume segmentation,gross tumor volume,artificial intelligence,deep learning,cross-species transfer learning
AI 理解论文
溯源树
样例
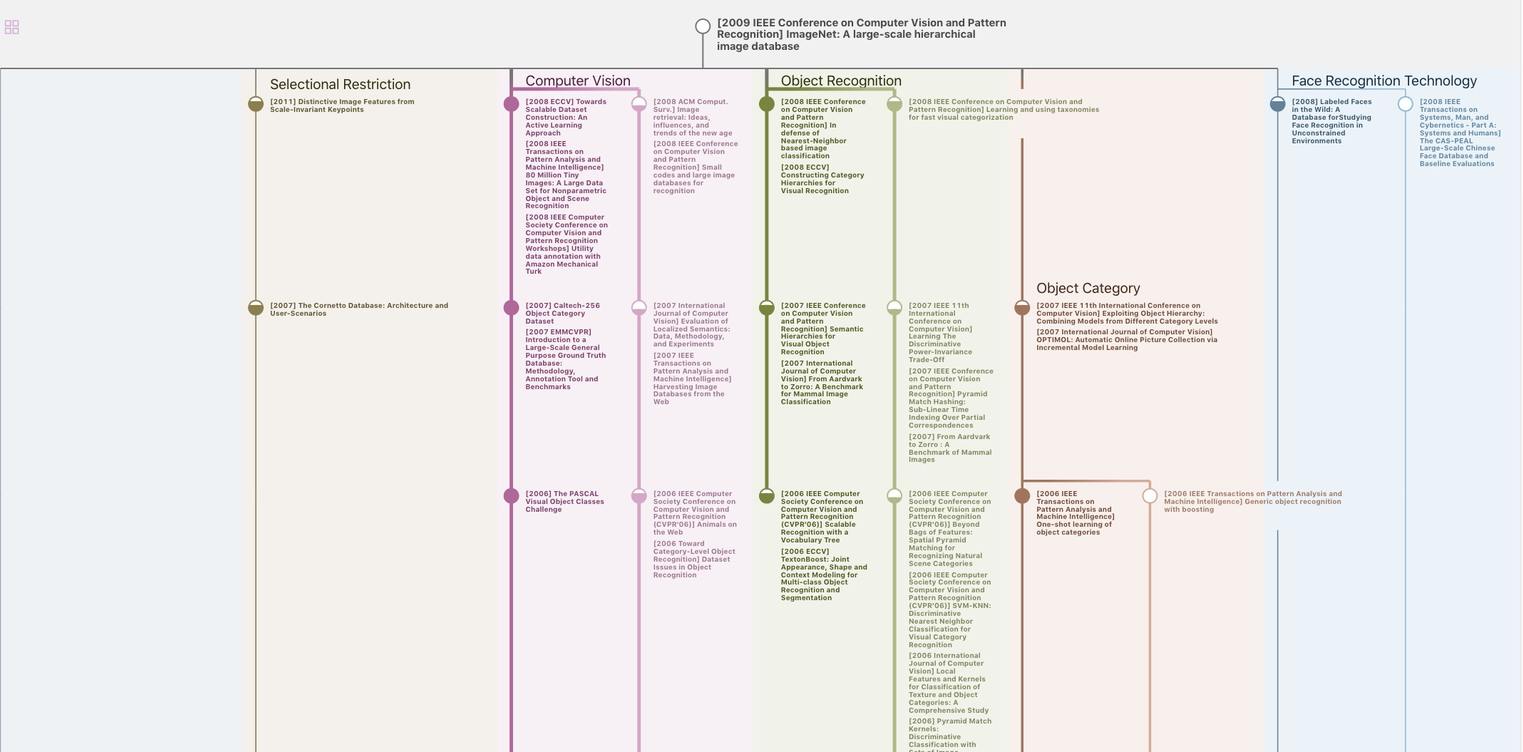
生成溯源树,研究论文发展脉络
Chat Paper
正在生成论文摘要