Toxicity Prediction in Pelvic Radiotherapy Using Multiple Instance Learning and Cascaded Attention Layers
IEEE Journal of Biomedical and Health Informatics(2023)
摘要
Modern radiotherapy delivers treatment plans optimised on an individual patient level, using CT-based 3D models of patient anatomy. This optimisation is fundamentally based on simple assumptions about the relationship between radiation dose delivered to the cancer (increased dose will increase cancer control) and normal tissue (increased dose will increase rate of side effects). The details of these relationships are still not well understood, especially for radiation-induced toxicity. We propose a convolutional neural network based on multiple instance learning to analyse toxicity relationships for patients receiving pelvic radiotherapy. A dataset comprising of 315 patients were included in this study; with 3D dose distributions, pre-treatment CT scans with annotated abdominal structures, and patient-reported toxicity scores provided for each participant. In addition, we propose a novel mechanism for segregating the attentions over space and dose/imaging features independently for a better understanding of the anatomical distribution of toxicity. Quantitative and qualitative experiments were performed to evaluate the network performance. The proposed network could predict toxicity with 80% accuracy. Attention analysis over space demonstrated that there was a significant association between radiation dose to the anterior and right iliac of the abdomen and patient-reported toxicity. Experimental results showed that the proposed network had outstanding performance for toxicity prediction, localisation and explanation with the ability of generalisation for an unseen dataset.
更多查看译文
关键词
Deep learning,multiple instance learning,radiotherapy,outcome prediction,toxicity map
AI 理解论文
溯源树
样例
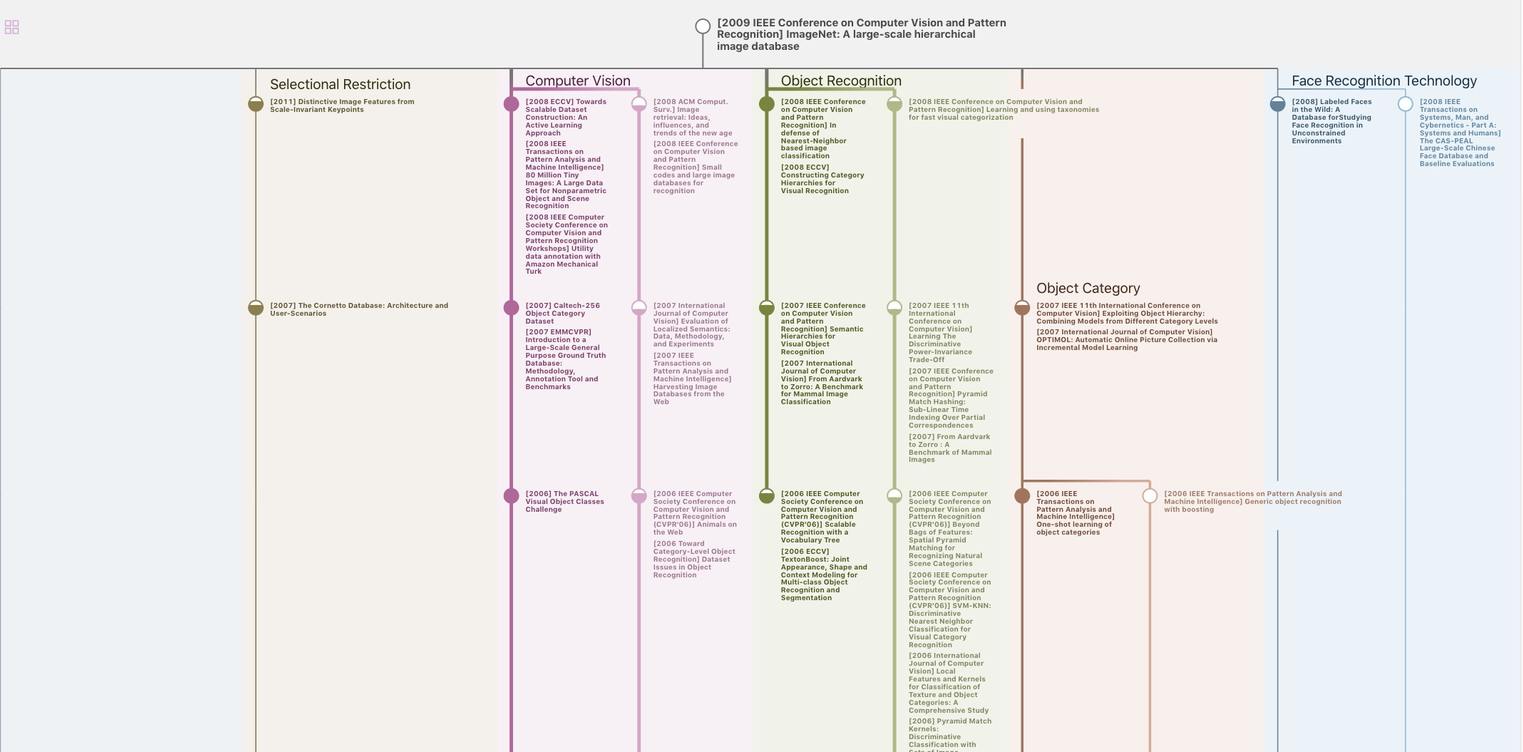
生成溯源树,研究论文发展脉络
Chat Paper
正在生成论文摘要