Deep learning enhanced NIR-II volumetric imaging of whole mice vasculature
user-61447a76e55422cecdaf7d19(2023)
摘要
Fluorescence imaging through the second near-infrared window (NIR-II,1000-1700 nm) allows in-depth imaging. However, current imaging systems use wide-field illumination and can only provide low-contrast 2D information, without depth resolution. Here, we systematically apply a light-sheet illumination, a time-gated detection, and a deep-learning al-gorithm to yield high-contrast high-resolution volumetric images. To achieve a large FoV (field of view) and minimize the scattering effect, we generate a light sheet as thin as 100.5 mu m with a Rayleigh length of 8 mm to yield an axial resolu-tion of 220 mu m. To further suppress the background, we time-gate to only detect long lifetime luminescence achieving a high contrast of up to 0.45 /contrast. To enhance the resolution, we develop an algorithm based on profile protrusions de-tection and a deep neural network and distinguish vasculature from a low-contrast area of 0.07 /contrast to resolve the 100 mu m small vessels. The system can rapidly scan a volume of view of 75 x 55 x 20 mm3 and collect 750 images within 6 mins. By adding a scattering-based modality to acquire the 3D surface profile of the mice skin, we reveal the whole volu-metric vasculature network with clear depth resolution within more than 1 mm from the skin. High-contrast large-scale 3D animal imaging helps us expand a new dimension in NIR-II imaging.
更多查看译文
关键词
NIR-II fluorescence,time-gated,light sheet illumination,deep learning,vessel enhancement,3D imaging
AI 理解论文
溯源树
样例
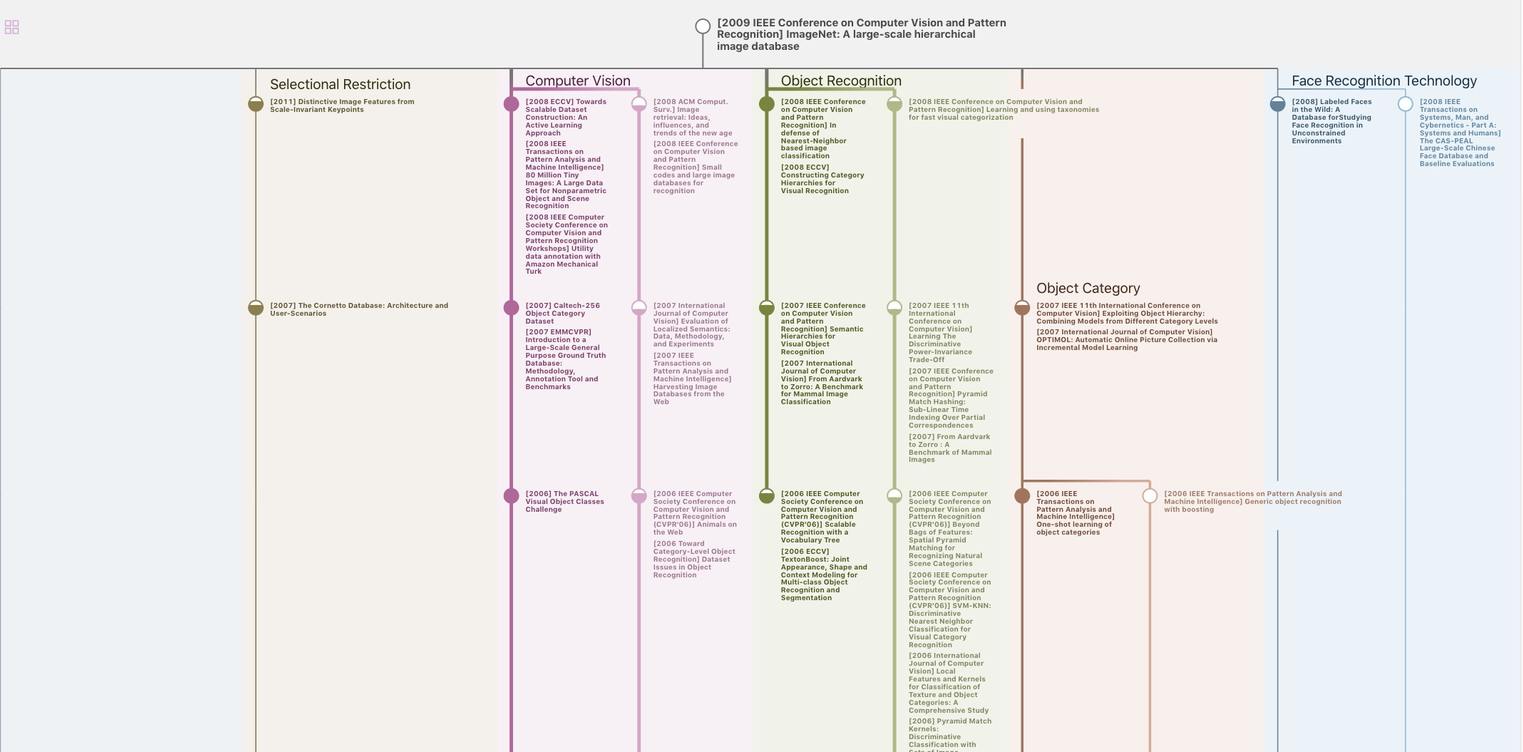
生成溯源树,研究论文发展脉络
Chat Paper
正在生成论文摘要