Implicit Anatomical Rendering for Medical Image Segmentation with Stochastic Experts
MEDICAL IMAGE COMPUTING AND COMPUTER ASSISTED INTERVENTION, MICCAI 2023, PT III(2023)
摘要
Integrating high-level semantically correlated contents and low-level anatomical features is of central importance in medical image segmentation. Towards this end, recent deep learning-based medical segmentation methods have shown great promise in better modeling such information. However, convolution operators for medical segmentation typically operate on regular grids, which inherently blur the high-frequency regions, i.e., boundary regions. In this work, we propose MORSE, a generic implicit neural rendering framework designed at an anatomical level to assist learning in medical image segmentation. Our method is motivated by the fact that implicit neural representation has been shown to be more effective in fitting complex signals and solving computer graphics problems than discrete grid-based representation. The core of our approach is to formulate medical image segmentation as a rendering problem in an end-to-end manner. Specifically, we continuously align the coarse segmentation prediction with the ambiguous coordinate-based point representations and aggregate these features to adaptively refine the boundary region. To parallelly optimize multi-scale pixel-level features, we leverage the idea from Mixture-of-Expert (MoE) to design and train our MORSE with a stochastic gating mechanism. Our experiments demonstrate that MORSE can work well with different medical segmentation backbones, consistently achieving competitive performance improvements in both 2D and 3D supervised medical segmentation methods. We also theoretically analyze the superiority of MORSE.
更多查看译文
关键词
Medical Image Segmentation,Implicit Neural Representation,Stochastic Mixture-of-Experts
AI 理解论文
溯源树
样例
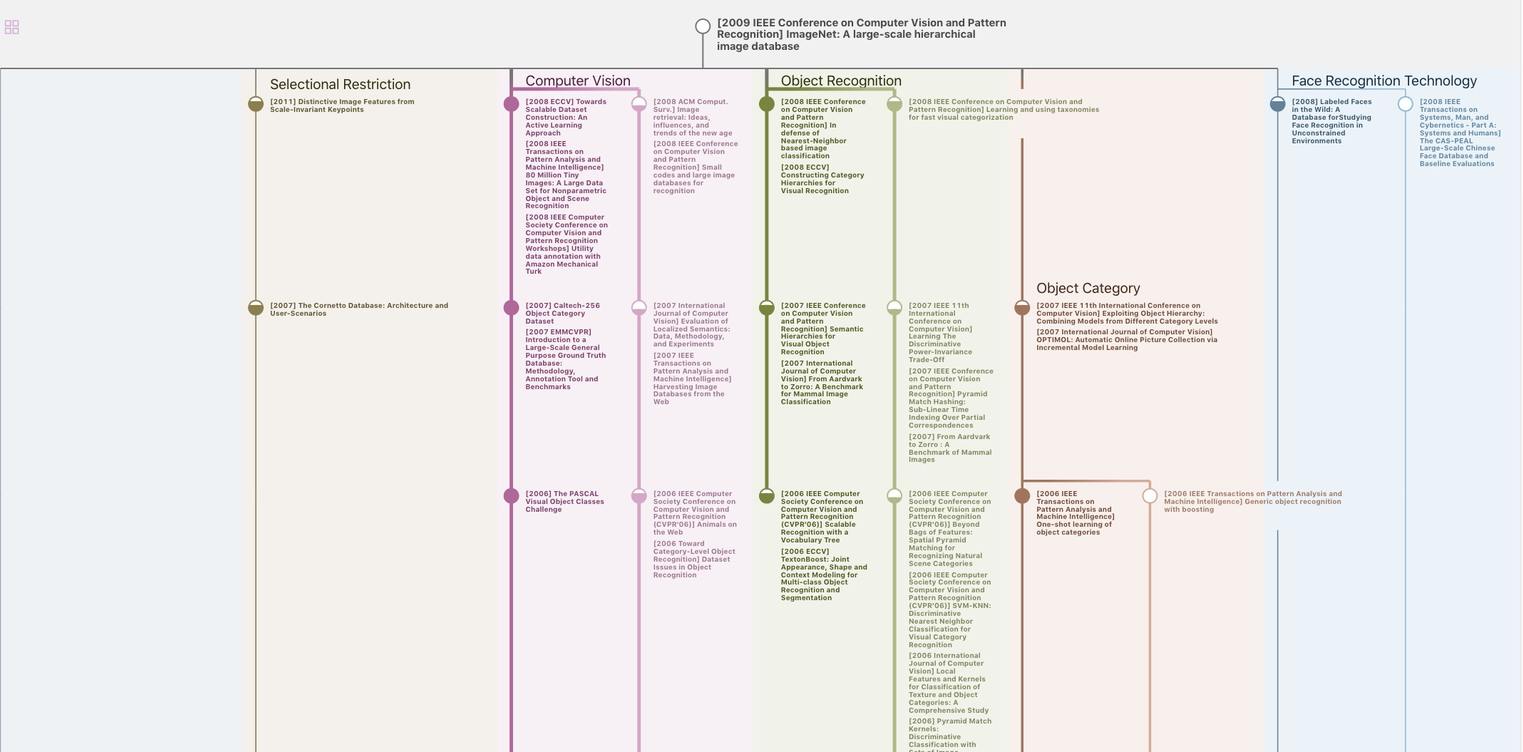
生成溯源树,研究论文发展脉络
Chat Paper
正在生成论文摘要