CoT-MAE v2: Contextual Masked Auto-Encoder with Multi-view Modeling for Passage Retrieval
CoRR(2023)
摘要
Growing techniques have been emerging to improve the performance of passage retrieval. As an effective representation bottleneck pretraining technique, the contextual masked auto-encoder utilizes contextual embedding to assist in the reconstruction of passages. However, it only uses a single auto-encoding pre-task for dense representation pre-training. This study brings multi-view modeling to the contextual masked auto-encoder. Firstly, multi-view representation utilizes both dense and sparse vectors as multi-view representations, aiming to capture sentence semantics from different aspects. Moreover, multiview decoding paradigm utilizes both autoencoding and auto-regressive decoders in representation bottleneck pre-training, aiming to provide both reconstructive and generative signals for better contextual representation pretraining. We refer to this multi-view pretraining method as CoT-MAE v2. Through extensive experiments, we show that CoT-MAE v2 is effective and robust on large-scale passage retrieval benchmarks and out-of-domain zero-shot benchmarks.
更多查看译文
关键词
cot-mae,auto-encoder,multi-view
AI 理解论文
溯源树
样例
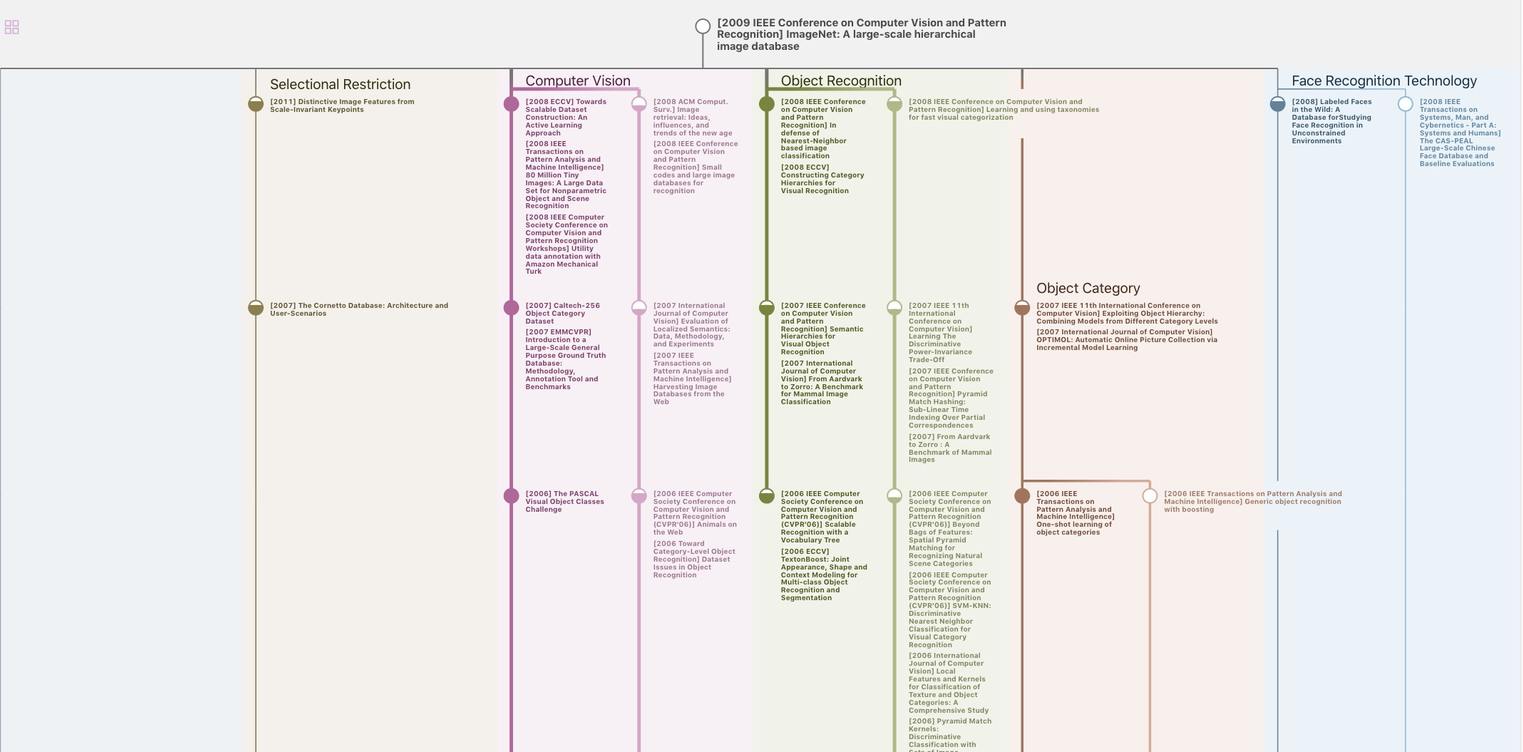
生成溯源树,研究论文发展脉络
Chat Paper
正在生成论文摘要