Patch-wise Features for Blur Image Classification
CoRR(2023)
摘要
Images captured through smartphone cameras often suffer from degradation, blur being one of the major ones, posing a challenge in processing these images for downstream tasks. In this paper we propose low-compute lightweight patch-wise features for image quality assessment. Using our method we can discriminate between blur vs sharp image degradation. To this end, we train a decision-tree-based XGBoost model on various intuitive image features like gray level variance, first and second order gradients, texture features like local binary patterns. Experiments conducted on an open dataset show that the proposed low compute method results in 90.1% mean accuracy on the validation set, which is comparable to the accuracy of a compute-intensive VGG16 network with 94% mean accuracy fine-tuned to this task. To demonstrate the generalizability of our proposed features and model we test the model on BHBID dataset and an internal dataset where we attain accuracy of 98% and 91%, respectively. The proposed method is 10x faster than the VGG16 based model on CPU and scales linearly to the input image size making it suitable to be implemented on low compute edge devices.
更多查看译文
关键词
classification,features,image,patch-wise
AI 理解论文
溯源树
样例
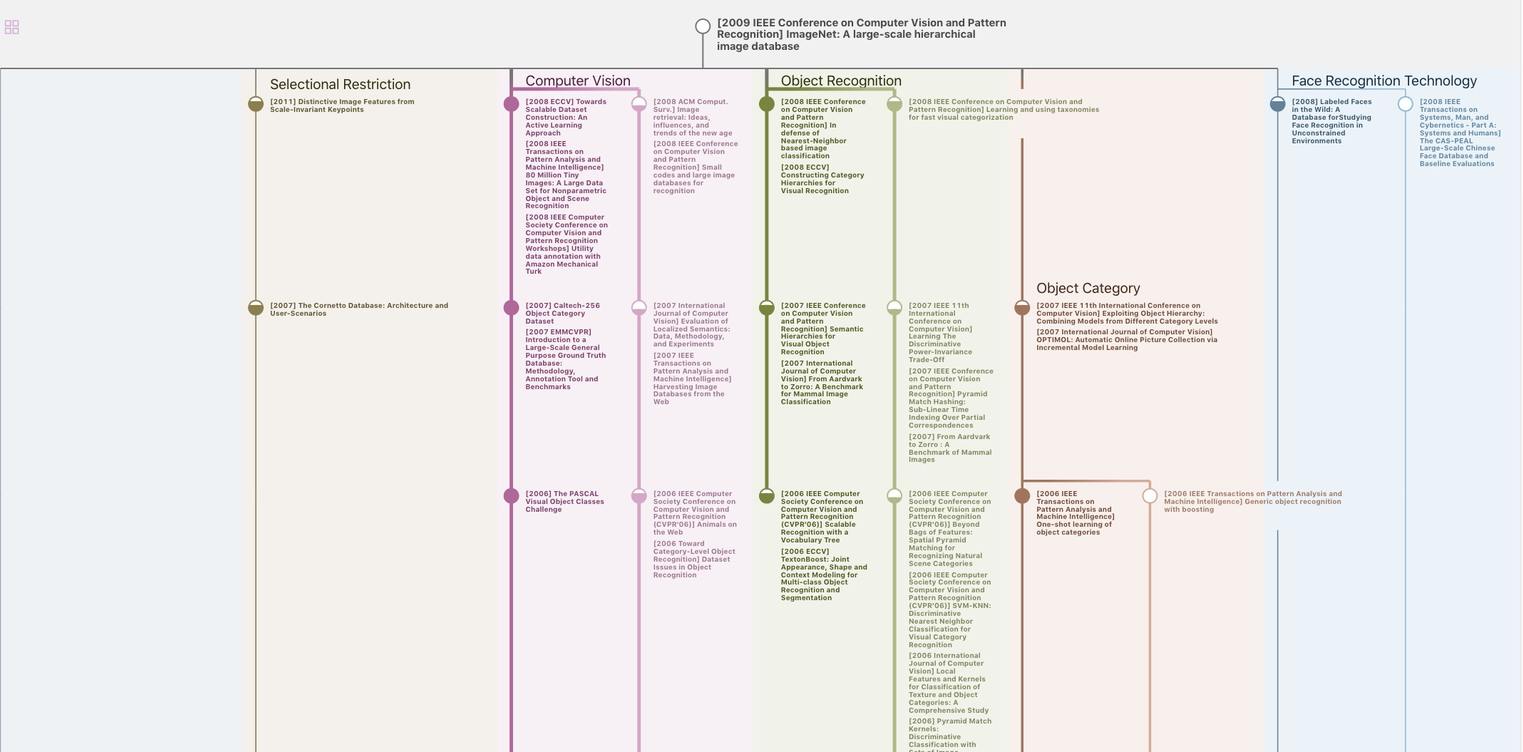
生成溯源树,研究论文发展脉络
Chat Paper
正在生成论文摘要