Statistical constraints on climate model parameters using a scalable cloud-based inference framework
Environmental Data Science(2023)
摘要
Atmospheric aerosols influence the Earth's climate, primarily by affecting cloud formation and scattering visible radiation. However, aerosol-related physical processes in climate simulations are highly uncertain. Constraining these processes could help improve model-based climate predictions. We propose a scalable statistical framework for constraining parameters in expensive climate models by comparing model outputs with observations. Using the C3.ai Suite, a cloud computing platform, we use a perturbed parameter ensemble of the UKESM1 climate model to efficiently train a surrogate model. A method for estimating a data-driven model discrepancy term is described. The strict bounds method is applied to quantify parametric uncertainty in a principled way. We demonstrate the scalability of this framework with two weeks' worth of simulated aerosol optical depth data over the South Atlantic and Central African region, written from the model every three hours and matched in time to twice-daily MODIS satellite observations. When constraining the model using real satellite observations, we establish constraints on combinations of two model parameters using much higher time-resolution outputs from the climate model than previous studies. This result suggests that, within the limits imposed by an imperfect climate model, potentially very powerful constraints may be achieved when our framework is scaled to the analysis of more observations and for longer time periods.
更多查看译文
关键词
climate model parameters,statistical constraints,cloud-based
AI 理解论文
溯源树
样例
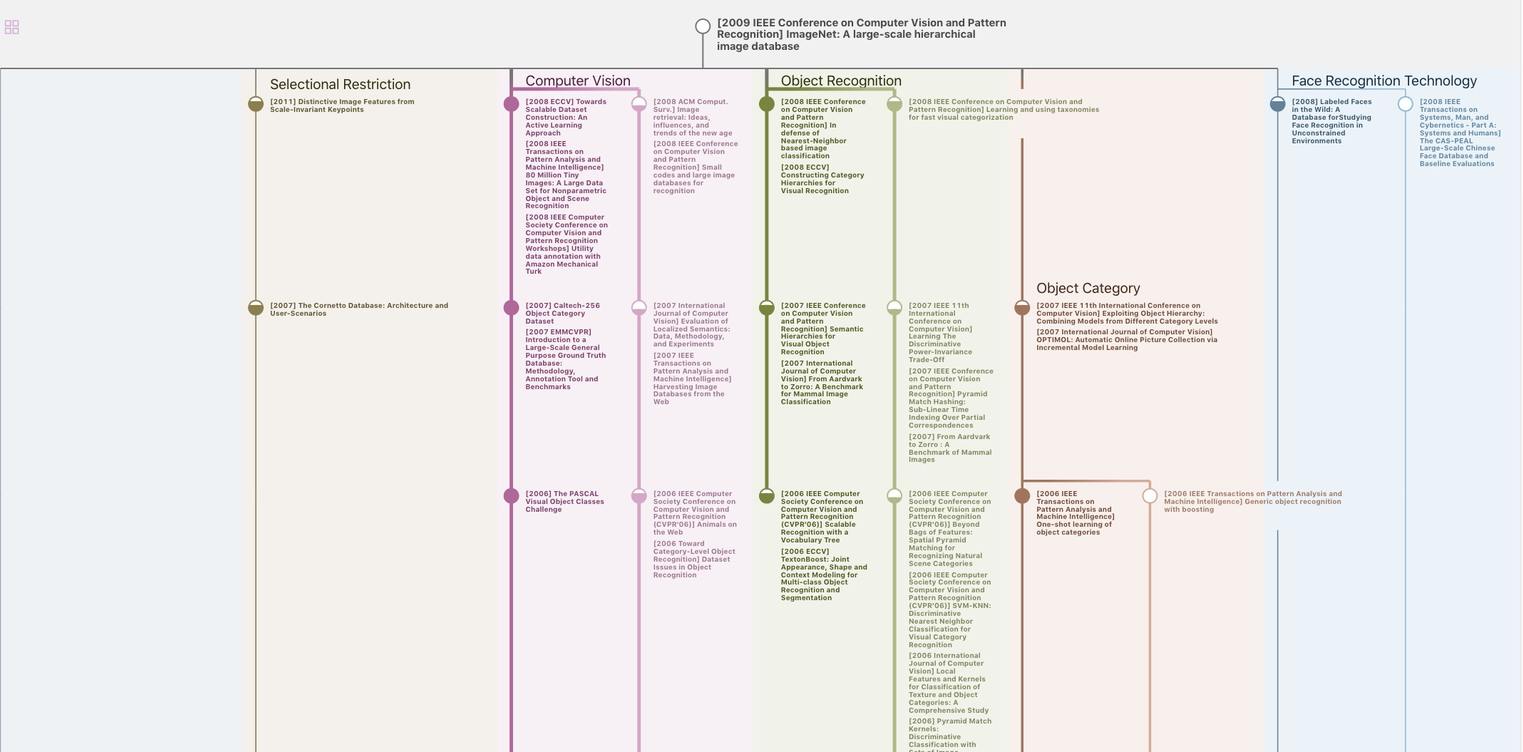
生成溯源树,研究论文发展脉络
Chat Paper
正在生成论文摘要