PopulAtion Parameter Averaging (PAPA)
CoRR(2023)
摘要
Ensemble methods combine the predictions of multiple models to improve performance, but they require significantly higher computation costs at inference time. To avoid these costs, multiple neural networks can be combined into one by averaging their weights (model soups). However, this usually performs significantly worse than ensembling. Weight averaging is only beneficial when weights are similar enough (in weight or feature space) to average well but different enough to benefit from combining them. Based on this idea, we propose PopulAtion Parameter Averaging (PAPA): a method that combines the generality of ensembling with the efficiency of weight averaging. PAPA leverages a population of diverse models (trained on different data orders, augmentations, and regularizations) while occasionally (not too often, not too rarely) replacing the weights of the networks with the population average of the weights. PAPA reduces the performance gap between averaging and ensembling, increasing the average accuracy of a population of models by up to 1.1% on CIFAR-10, 2.4% on CIFAR-100, and 1.9% on ImageNet when compared to training independent (non-averaged) models.
更多查看译文
关键词
averaging,population,papa
AI 理解论文
溯源树
样例
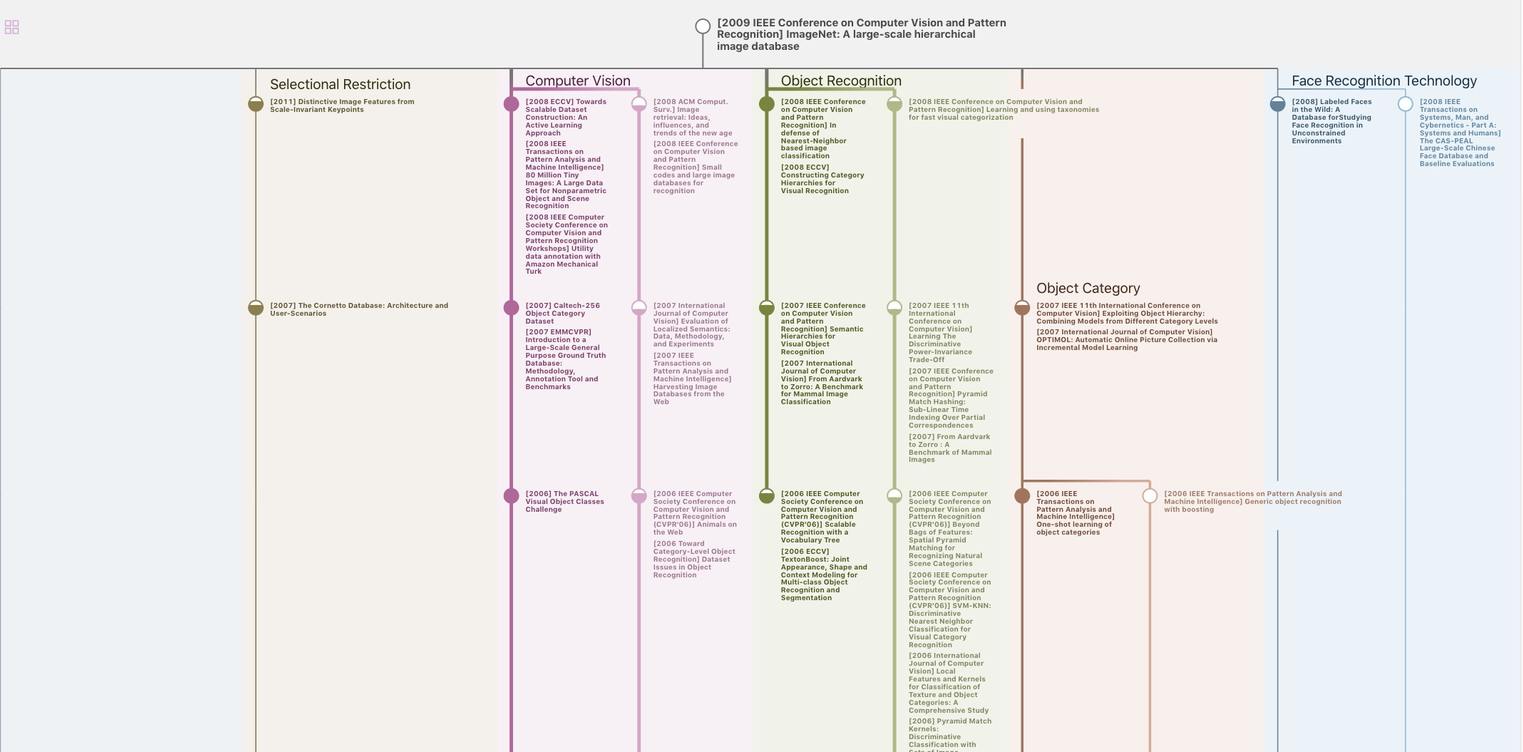
生成溯源树,研究论文发展脉络
Chat Paper
正在生成论文摘要