Severity-Based Hierarchical ECG Classification Using Neural Networks.
IEEE transactions on biomedical circuits and systems(2023)
摘要
Timely detection of cardiac arrhythmia characterized by abnormal heartbeats can help in the early diagnosis and treatment of cardiovascular diseases. Wearable healthcare devices typically use neural networks to provide the most convenient way of continuously monitoring heart activity for arrhythmia detection. However, it is challenging to achieve high accuracy and energy efficiency in these smart wearable healthcare devices. In this work, we provide architecture-level solutions to deploy neural networks for cardiac arrhythmia classification. We have created a hierarchical structure after analyzing various neural network topologies where only required network components are activated to improve energy efficiency while maintaining high accuracy. In our proposed architecture, we introduce a severity-based classification approach to directly help the users of the wearable healthcare device as well as the medical professionals. Additionally, we have employed computation-in-memory based hardware to improve energy efficiency and area consumption by leveraging in-situ data processing and scalability of emerging memory technologies such as resistive random access memory (RRAM). Simulation experiments conducted using the MIT-BIH arrhythmia dataset show that the proposed architecture provides high accuracy while consuming average energy of 0.11 μJ per heartbeat classification and 0.11 mm area, thereby achieving 25× improvement in average energy consumption and 12× improvement in area compared to the state-of-the-art.
更多查看译文
关键词
Humans,Electrocardiography,Neural Networks, Computer,Arrhythmias, Cardiac,Heart Rate,Wearable Electronic Devices,Signal Processing, Computer-Assisted,Algorithms
AI 理解论文
溯源树
样例
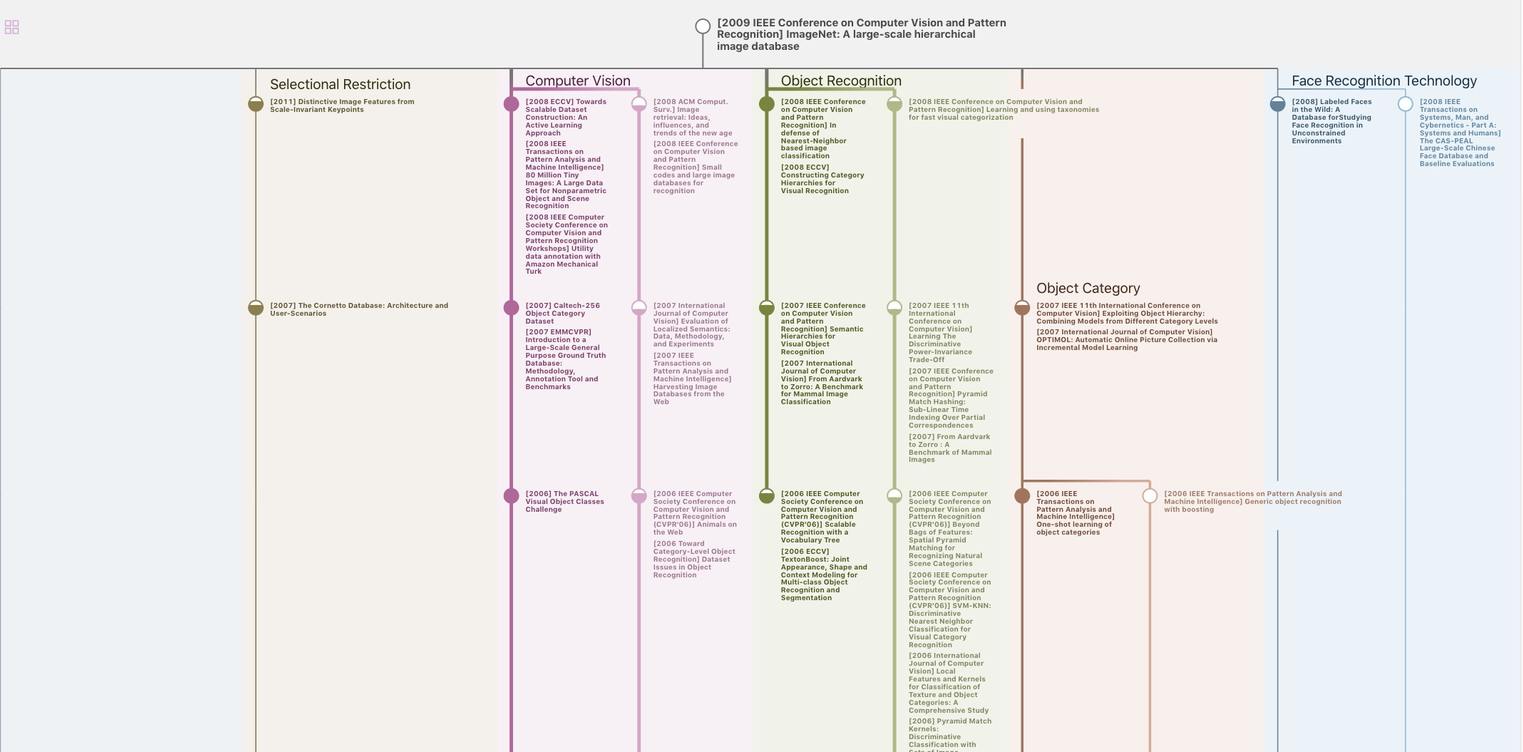
生成溯源树,研究论文发展脉络
Chat Paper
正在生成论文摘要