A reliable and fair federated learning mechanism for mobile edge computing.
Comput. Networks(2023)
摘要
Federated learning-enabled mobile edge computing implements privacy-preserving collaborative machine learning of complex models. However, mobile end devices have high mobility and are vulnerable to be corrupted due to exposuring to an open network environment, which not only affect the performance of federated learning models but also bring serious security issues. To solve the above problem, we propose a reliable and fair federated learning mechanism for mobile edge computing. To begin with, in order to select end nodes as reliable as possible, a reputation-based end nodes selection scheme is proposed, which includes the construction of the reputation model and the concealment of selected end nodes. Then, corresponding counter strategies are proposed for the possible attack behaviors launched by corrupted end nodes during the training process. To avoid the impact of poisoning attacks on the model performance, a new global model aggregation strategy is proposed to maintain the model performance by meritocratic campaigning. To solve the fairness problem caused by end nodes' escape, a reward-penalty scheme using blockchain is proposed. Finally, the numerical results clearly show that the proposed mechanism is effective for reliable and fair federated learning for mobile edge computing, maintaining high accuracy under attacks from malicious end nodes while penalizing them to ensure fairness.
更多查看译文
关键词
Federated learning,Mobile edge computing,Fairness,Reliable
AI 理解论文
溯源树
样例
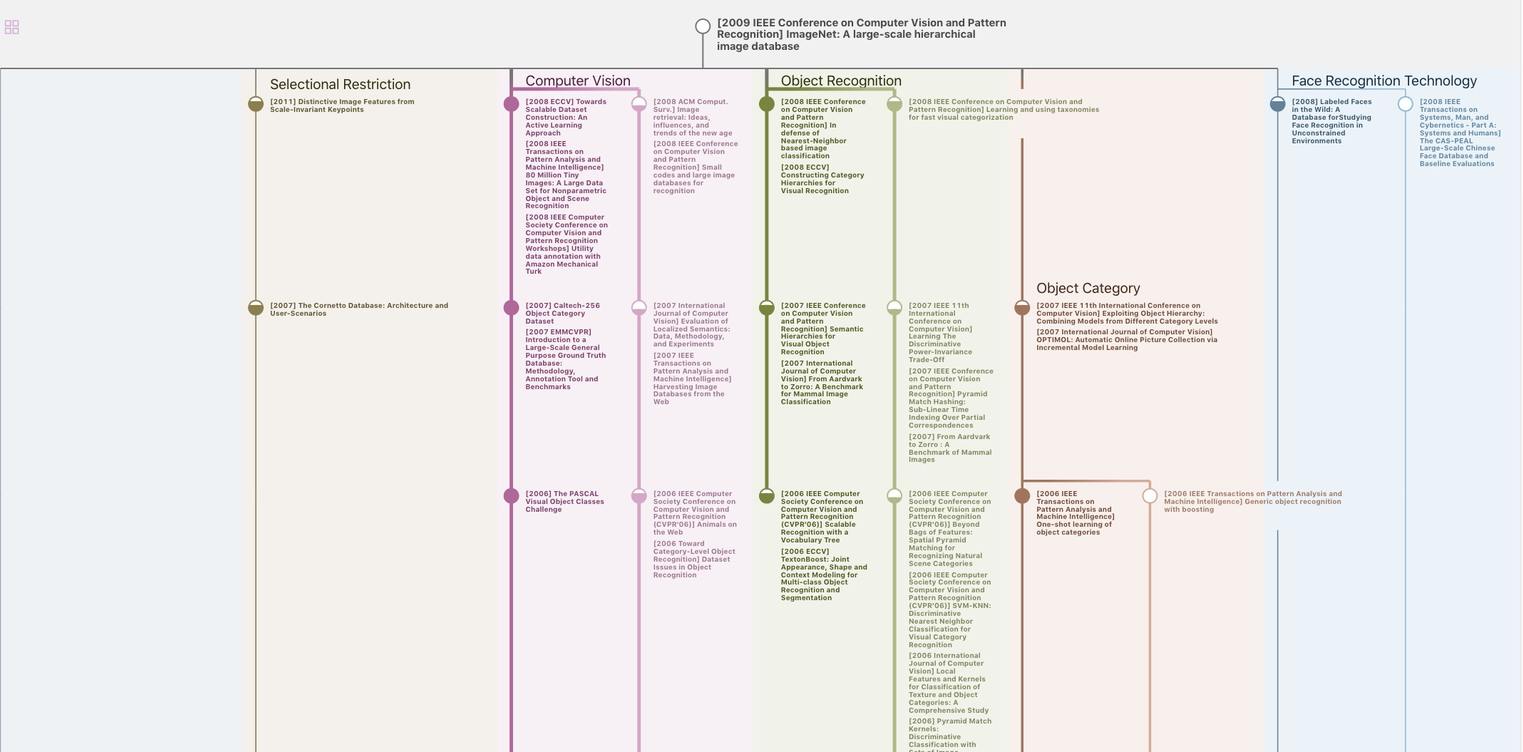
生成溯源树,研究论文发展脉络
Chat Paper
正在生成论文摘要