Adaptive Batch Size CGP: Improving Accuracy and Runtime for CGP Logic Optimization Flow.
EuroGP(2023)
摘要
With the recent advances in the Machine Learning field, alongside digital circuits becoming more complex each day, machine learning based methods are being used in error-tolerant applications to solve the challenges imposed by large integrated circuits, where the designer can obtain a better overall circuit while relaxing its accuracy requirement. One of these methods is the Cartesian Genetic Programming (CGP), a subclass of Evolutionary Algorithms that uses concepts from biological evolution applied in electronic design automation. CGP-based approaches show advantages in the logic learning and logic optimization processes. However, the main challenge of CGP-based flows is the extensive runtime compared to other logic synthesis strategies. We propose a new strategy to tackle this challenge, called Adaptive Batch Size (ABS) CGP, in which the CGP algorithm incrementally improves the fitness estimation of the candidate solutions by using more terms of the truth table for evaluating them along the evolutionary process. The proposed approach was evaluated in nine exemplars from the IWLS 2020 contest, in which 3 exemplars are from the arithmetic domain, and six are from image recognition domain, specifically three from the CIFAR-10 dataset and three from the MNIST dataset. The results show that ABS presented an accuracy increase of up to 8.19% and decreased the number of candidate solutions evaluations required by up to 84.56%, in which directly affects the runtime of the algorithm. Furthermore, for all circuits, no significant accuracy reduction was observed while a significant reduction in the number of evaluations was achieved.
更多查看译文
关键词
optimization,runtime
AI 理解论文
溯源树
样例
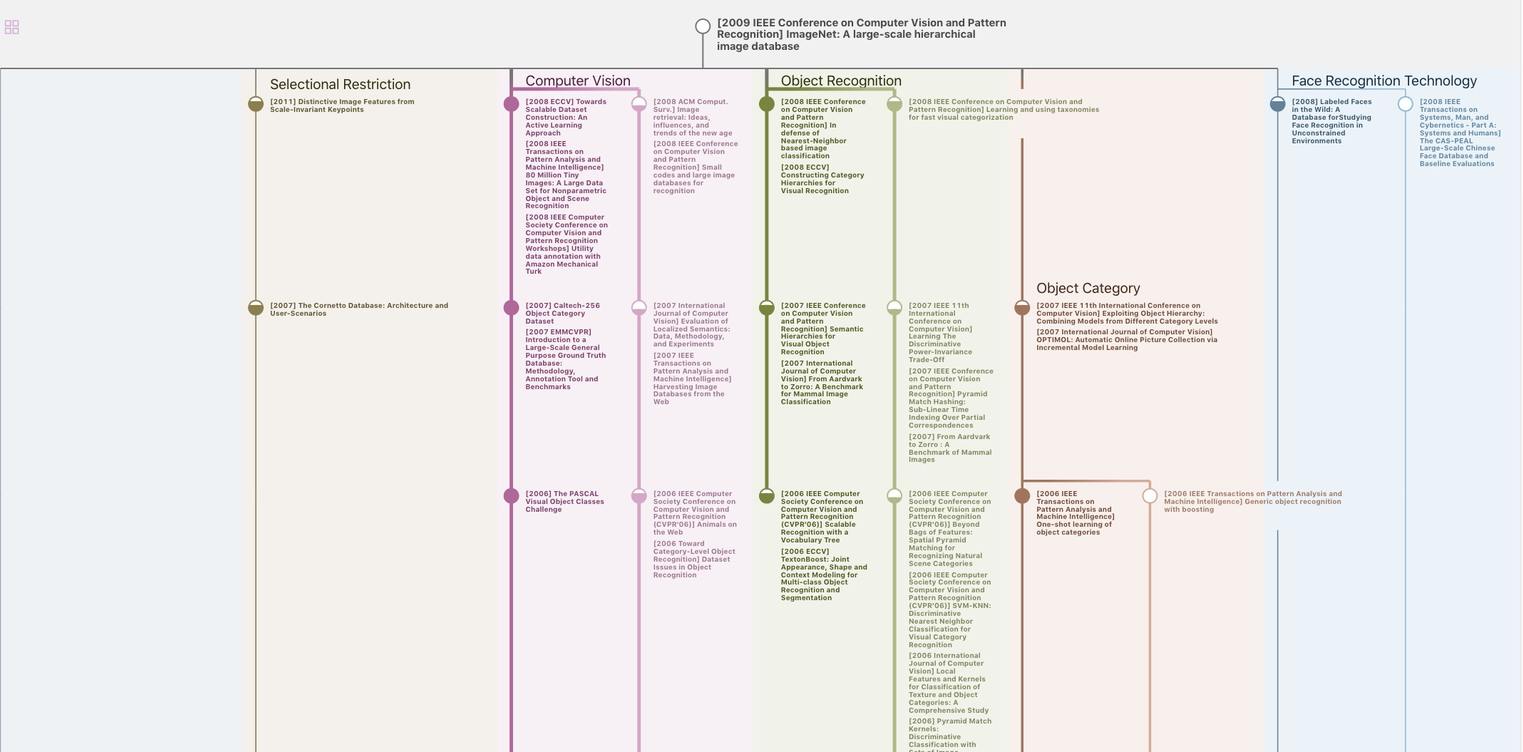
生成溯源树,研究论文发展脉络
Chat Paper
正在生成论文摘要