Fast Accurate Fish Recognition with Deep Learning Based on a Domain-Specific Large-Scale Fish Dataset.
MMM (1)(2023)
摘要
Fish species recognition is an integral part of sustainable marine biodiversity and aquaculture. The rapid emergence of deep learning methods has shown great potential on classification and recognition tasks when trained on a large scale dataset. Nevertheless, some practical challenges remain for automating the task, e.g., the lack of appropriate methods applied to a complicated fish habitat. In addition, most publicly accessible fish datasets have small-scale and low resolution, imbalanced data distributions, or limited labels and annotations, etc. In this work, we aim to overcome the aforementioned challenges. First, we construct the OceanFish database with higher image quality and resolution that covers a large scale and diversity of marine-domain fish species in East China sea. The current version covers 63, 622 pictures of 136 fine-grained fish species. Accompanying the dataset, we propose a fish recognition test-bed by incorporating two widely applied deep neural network based object detection models to exploit the facility of the enlarged dataset, which achieves a convincing performance in detection precision and speed. The scale and hierarchy of OceanFish can be further enlarged by enrolling new fish species and annotations. Interested readers may ask for access and re-use this benchmark datasets for their own classification tasks upon inquiries. We hope that the OceanFish database and the fish recognition testbed can serve as a generalized benchmark that motivates further development in related research communities.
更多查看译文
关键词
Fish recognition, Deep learning, Data augmentation, Convolutional neural networks
AI 理解论文
溯源树
样例
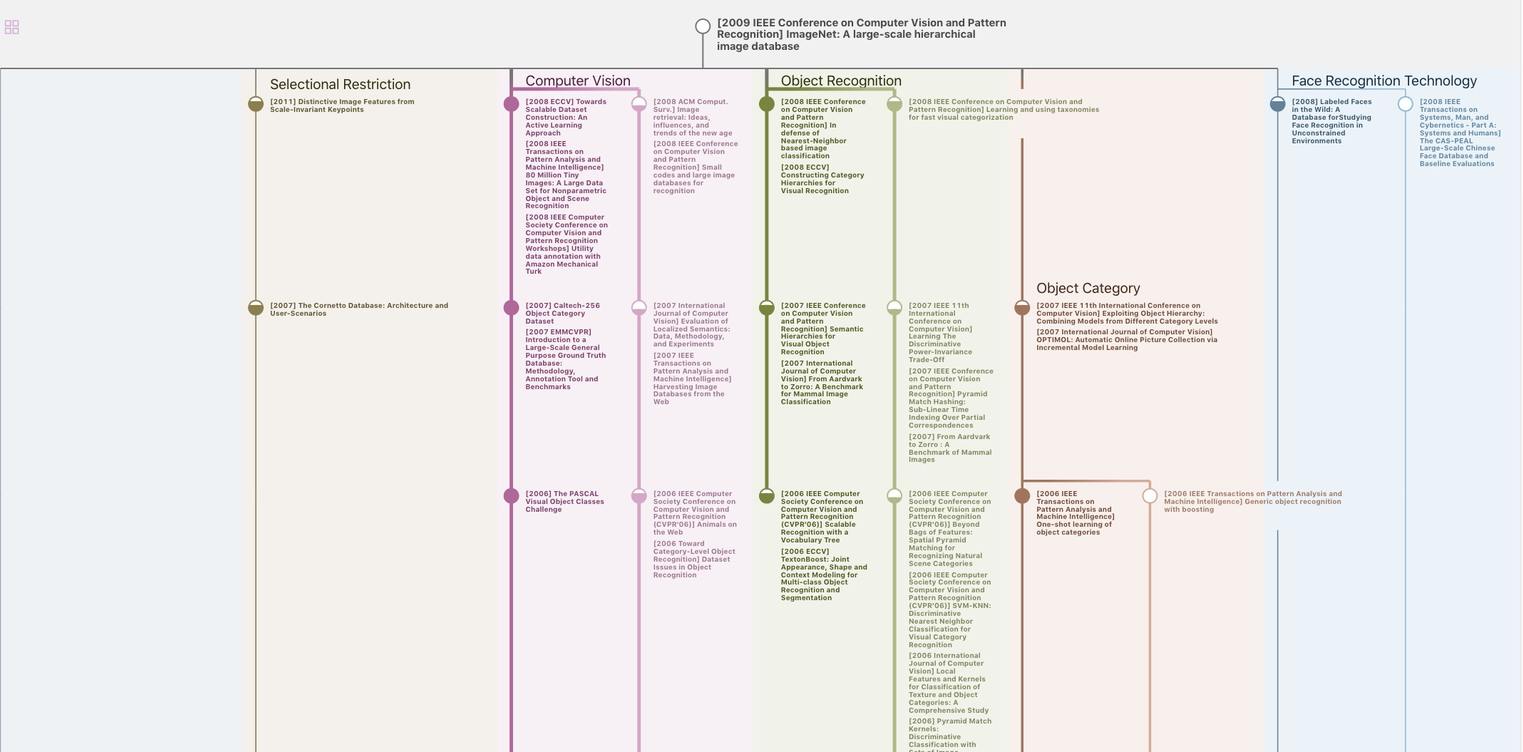
生成溯源树,研究论文发展脉络
Chat Paper
正在生成论文摘要