Toward More Accurate Heterogeneous Iris Recognition with Transformers and Capsules.
MMM (1)(2023)
摘要
As diverse iris capture devices have been deployed, the performance of iris recognition under heterogeneous conditions, e.g., cross-spectral matching and cross-sensor matching, has drastically degraded. Nevertheless, the performance of existing manual descriptor-based methods and CNN-based methods is limited due to the enormous domain gap under heterogeneous acquisition. To tackle this problem, we propose a model with transformers and capsules to extract and match the domain-invariant feature effectively and efficiently. First, we represent the features from shallow convolution as vision tokens by spatial attention. Then we model the high-level semantic information and fine-grained discriminative features in the token-based space by a transformer encoder. Next, a Siamese transformer decoder exploits the relationship between pixel-space and token-based space to enhance the domain-invariant and discriminative properties of convolution features. Finally, a 3D capsule network not only efficiently considers part-whole relationships but also increases intra-class compactness and inter-class separability. Therefore, it improves the robustness of heterogeneous recognition. Experimental validation results on two common datasets show that our method significantly outperforms the state-of-the-art methods.
更多查看译文
关键词
recognition,transformers
AI 理解论文
溯源树
样例
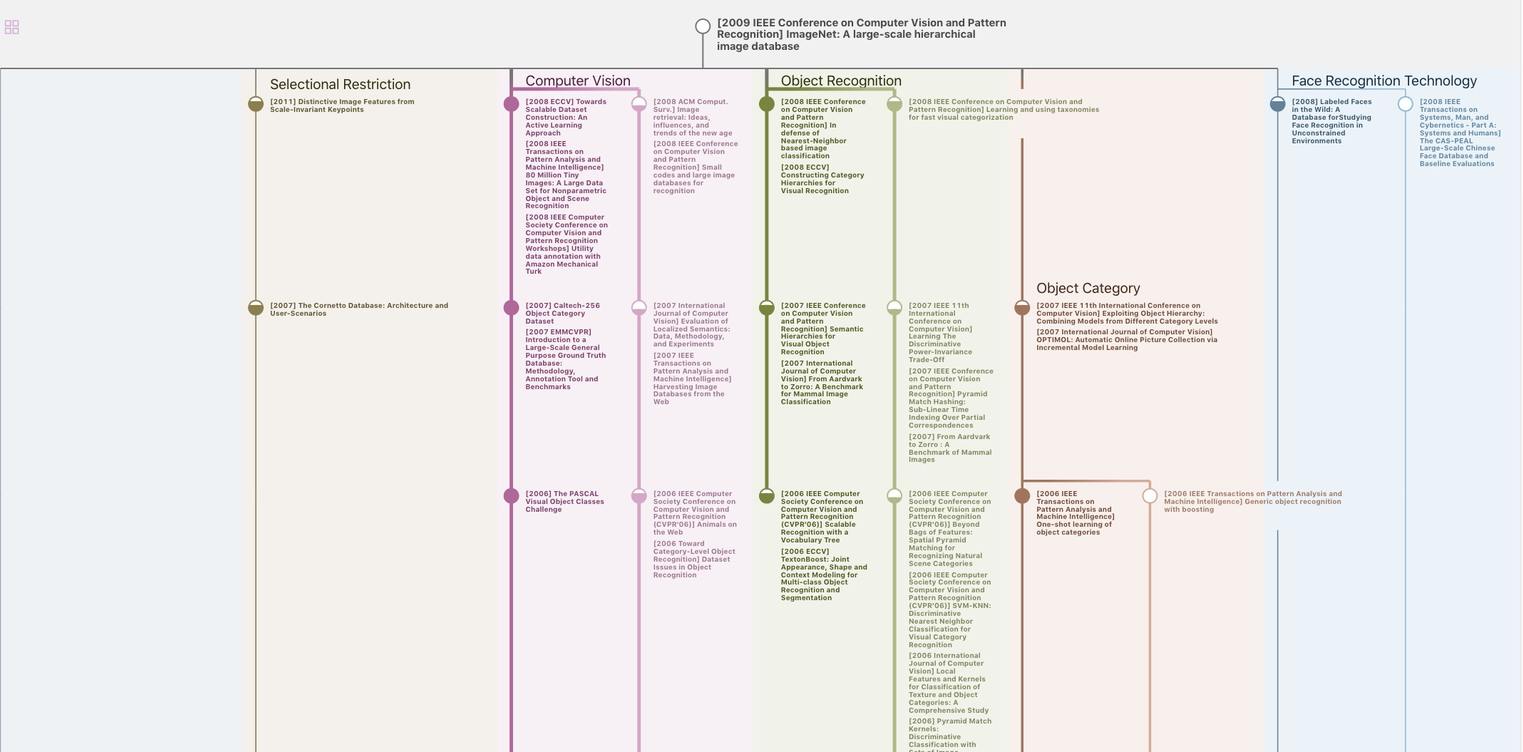
生成溯源树,研究论文发展脉络
Chat Paper
正在生成论文摘要