Contributing factors on the level of delay caused by crashes: a hybrid method of latent class analysis and XGBoost based SHAP algorithm
JOURNAL OF TRANSPORTATION SAFETY & SECURITY(2024)
摘要
Road crashes cause significant traffic delay and bring unnecessary financial losses. This study investigates the impact of contributing factors on the level of delay caused by crashes (LDC) using Texas crash data. To capture the unobserved heterogeneity, a latent class analysis (LCA) was first used to segment the whole dataset into several homogeneous clusters. Then, XGBoost based SHAP was developed on each cluster to identify the main contributing factors hidden in the latent classes. The interaction effects between the contributing factors were subsequently analyzed, including the effects between high importance features and between high and low importance features. The LCA results indicate that season is the main factor producing heterogeneity, hence the data were divided into four clusters. The main contributing factors and the interaction effects are different among the four clusters, as shown by the XGBoost based SHAP algorithm. For example, Sunrise_Sunset, Peak_hours and Crossing are the main contributing factors in Fall and Winter crash, whereas Traffic_Signal, Workday and Junction are the main contributing factors in Summer and Spring crash. The interaction effects of Highway and Zone are different in Fall and Winter crash. This study can provide insightful information for regulators to develop targeted policies in different seasons.
更多查看译文
关键词
Road crash,The level of delay caused by crashes (LDC),Unobserved heterogeneity,Latent class analysis,XGBoost based SHAP
AI 理解论文
溯源树
样例
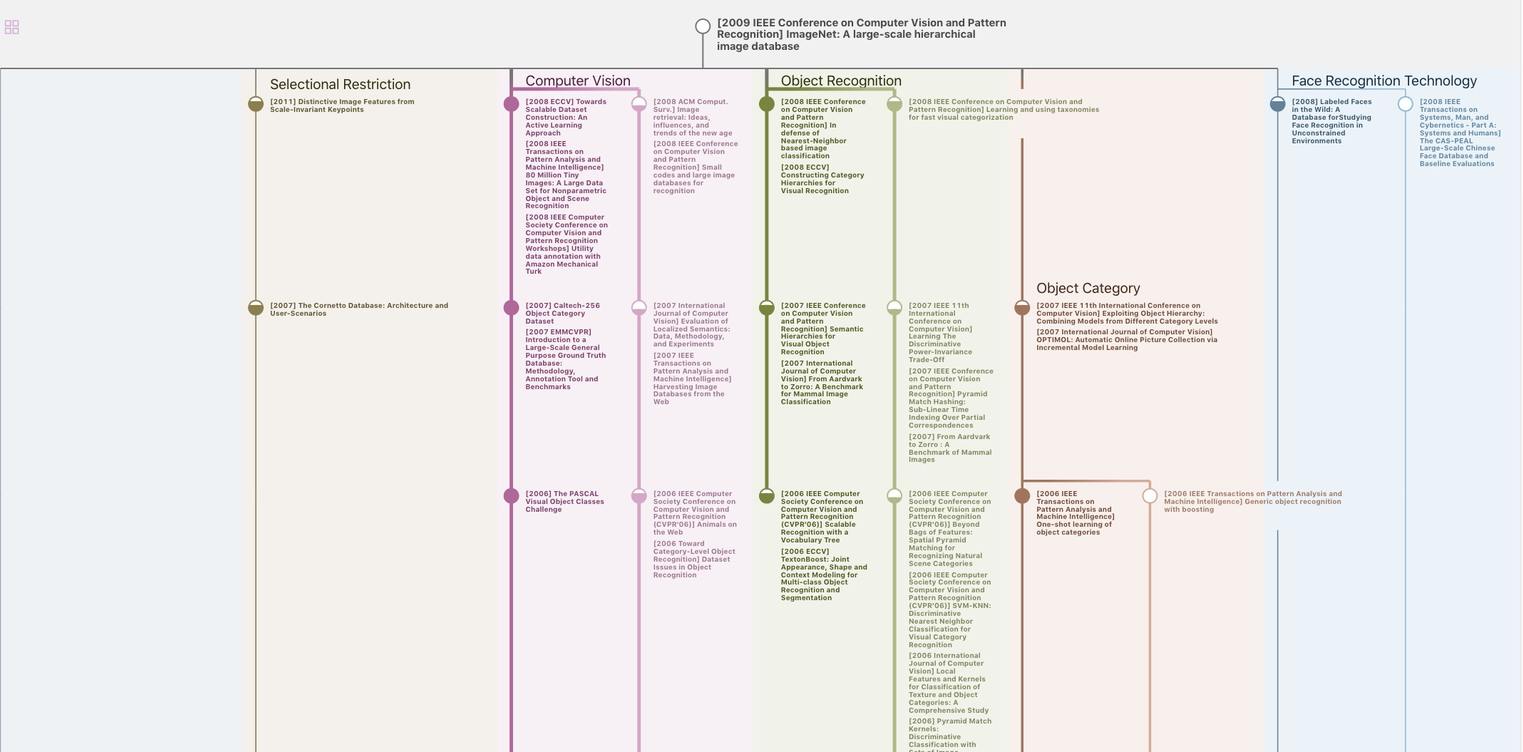
生成溯源树,研究论文发展脉络
Chat Paper
正在生成论文摘要