RsALUNet: A reinforcement supervision U-Net-based framework for multi-ROI segmentation of medical images
BIOMEDICAL SIGNAL PROCESSING AND CONTROL(2023)
摘要
A new multiple region of interest (multi-ROI) segmentation framework, RsALUNet, is proposed in this paper, whose backbone was U-Net. Rs represented the reinforcement-supervision strategy by utilizing adversarial learning (AL) between U-Net's decoders and an additional discriminator, which was based on the differences among the segmentation results (diffSegs) and labels of multi-ROI (diffLabels). As the AL progressed, diffSegs was increasingly similar to diffLabels, and this further contributed to a more accurate segmentation of each member in the multi-ROI. In addition to the Rs strategy, three blocks were proposed to enhance RsALUNet, namely, a dilated convolution chain providing diverse and large receptive fields to accommodate different target sizes, a fusion block integrating features of large targets to small ones to optimize the segmentation of the latter, and a location-encoder block extracting multi-scale positional information to enhance the model's attention to the ROI. RsA-LUNet was evaluated through three multi-ROI segmentation tasks using different imaging modalities, including X-ray, ultrasound, and magnetic-resonance (MR) imaging. The mean Dice coefficient (Dice) increased from 1.1% to 8.5% compared to the other frameworks. The results demonstrate the promising adaptability and extendibility of our strategy and RsALUNet for multi-ROI segmentation in X-ray, ultrasound, and MR images.
更多查看译文
关键词
Multi-ROI segmentation framework,Difference,Reinforcement supervision,Adversarial learning
AI 理解论文
溯源树
样例
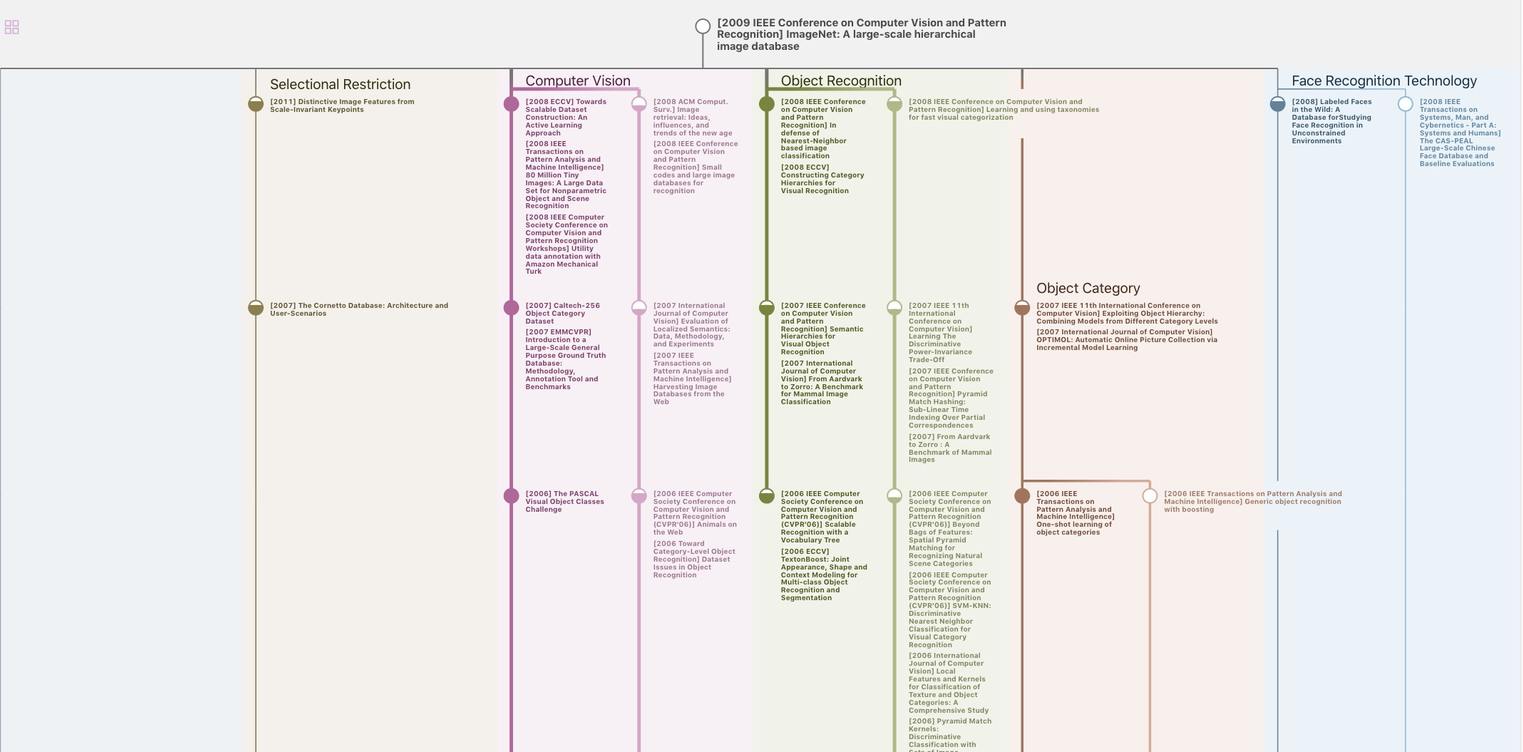
生成溯源树,研究论文发展脉络
Chat Paper
正在生成论文摘要