Using Machine Learning to Expedite the Screening of Environmental Factors Associated with the Risk of Spontaneous Preterm Birth: From Exposure Mixtures to Key Molecular Events
ENVIRONMENTAL SCIENCE & TECHNOLOGY LETTERS(2023)
摘要
Spontaneous preterm birth (SPB) is affected by various environmental exposures. However, there is still an urgent need to efficiently integrate exposomic information to build its prediction model and unveil the potential toxic pathways. Here, we conducted a nested case-control study by recruiting 30 women with SPB delivery (cases) and 30 women without (controls) at their early pregnancy. We analyzed various biomarkers of external chemical exposure, lipidomics, and immunity, resulting in 1081 exposure features. A logistic regression model (LR) was used to screen potential risk factors, and four statistical learners were used to establish SPB prediction models. Overall, the serum lipid concentration in cases was higher than in controls, while this was not the case for chemical and immune biomarkers. Random forest (RF) and extreme gradient boosting (XGboost) models had a relatively higher prediction accuracy of > 90%. Glycerophospholipids (GP) were the most abundant lipidomic features screened by LR, RF, and XGboost models, followed by glycerolipids and sphingolipids, shown as well as by enrichment analysis. Moreover, FA(21:0) had the largest contribution to the prediction performance. Maternal exposure to various elements can contribute to SPB risk due to their interaction with GP metabolism. Therefore, it is promising to use exposomic data to predict SPB risk and screen key molecular events.
更多查看译文
关键词
exposome,preterm birth,risk factor,lipidomics,machine learning
AI 理解论文
溯源树
样例
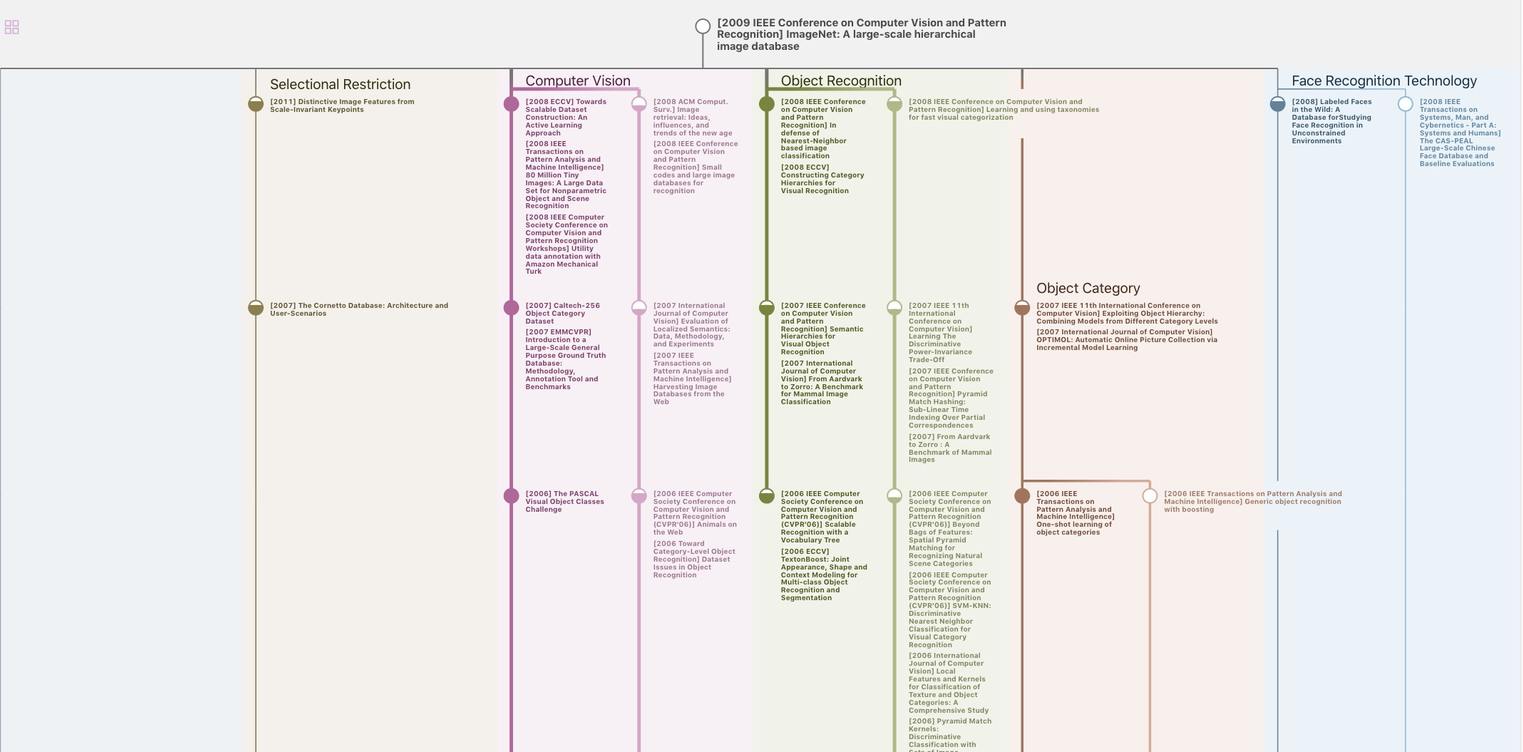
生成溯源树,研究论文发展脉络
Chat Paper
正在生成论文摘要