Temporal pattern mining of urban traffic volume data: a pairwise hybrid clustering method
TRANSPORTMETRICA B-TRANSPORT DYNAMICS(2023)
摘要
Multiple pattern analyses of traffic data have been conducted previously; however, it has yet to be explored with an awareness of temporal factors in big real-world traffic data. In this paper, we introduce a hybrid method to measure the intensity of differences among various temporal factors' data. The proposed method can efficiently process the historical data given temporal factors and provide insightful information about the intensity of variations. After data denoising with basis splines, we reshape the time series into a 2-D latent space using Principal Component Analysis (PCA) according to the type of analysis. Pairwise K-means clustering is then applied after anomaly elimination with DBSCAN to derive Adjusted Rand Index (ARI) matrices. Finally, these matrices are then systematically used to find similar patterns of different temporal perspectives. Multiple analyses are carried out with real data from Melbourne, Australia. Dissimilarities with intensities of up to 80% are detected that are not detectable with general clustering approaches.
更多查看译文
关键词
Temporal pattern mining,traffic volume data,pairwise clustering
AI 理解论文
溯源树
样例
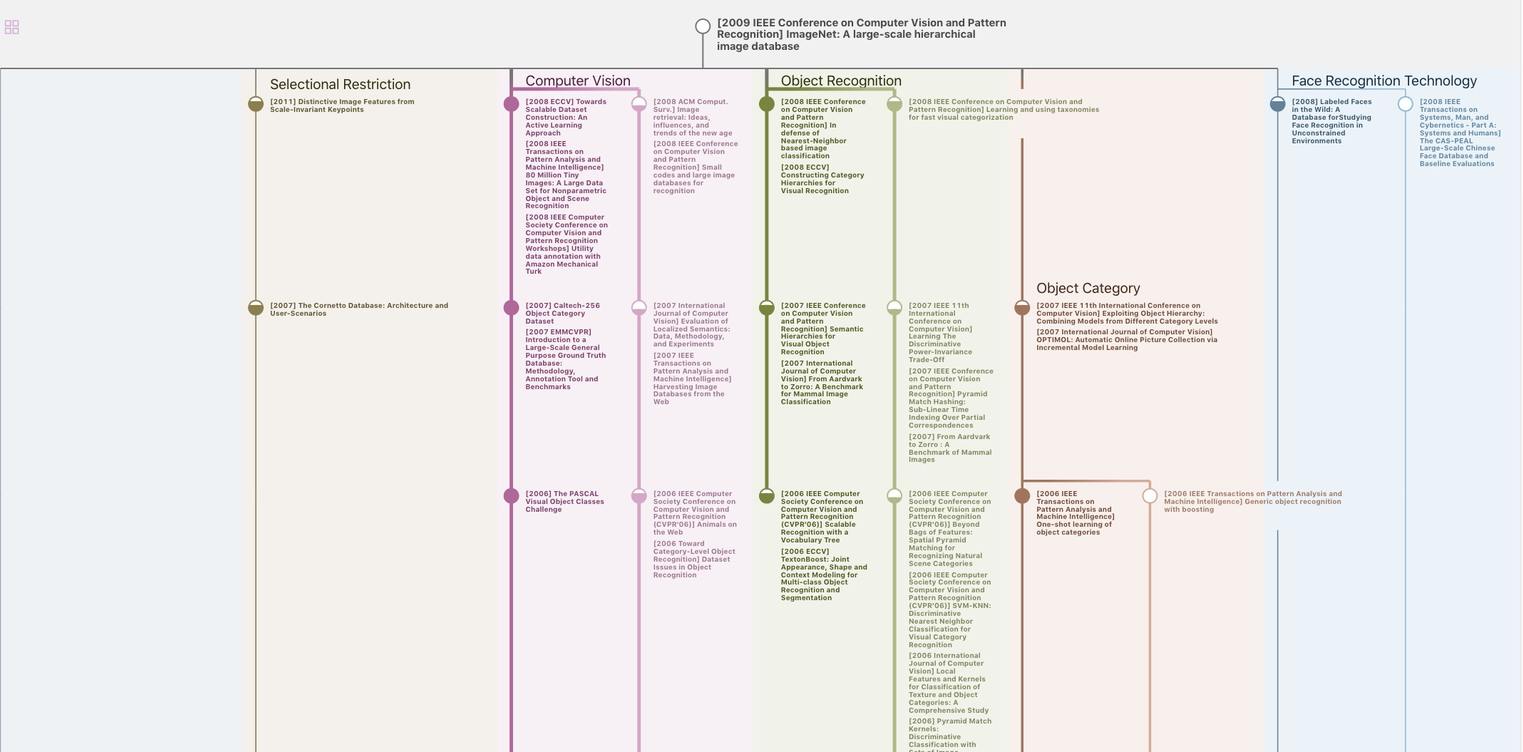
生成溯源树,研究论文发展脉络
Chat Paper
正在生成论文摘要