Machine Learning Based Approach for EVAP System Early Anomaly Detection Using Connected Vehicle Data
INTERNATIONAL JOURNAL OF PROGNOSTICS AND HEALTH MANAGEMENT(2023)
摘要
From automobile manufacturers perspective, reduction of warranty cost leads to less expenditures, which then yields higher profits. Thus, it is crucial to leverage the available methods and tools to achieve such outcome. Connected vehicle data is one critical resource that can be a gamechanger, reducing the associated costs and improving the business profitability. This project uses Mode06 connected vehicle data along with contextual data to early detect EVAP and purge monitors anomalies, yielding proactive fix of the detected issue through software (SW) and/or hardware (HW) upgrades (preventive maintenance and system quality improvement). Root cause analysis, which can be developed based on the anomaly detection outcomes and which is not within the scope of this paper, allows diagnostics of HW and/or SW related issues in a timely manner and eventually be prepared ahead of time for system failures. In this paper, statistics-based early anomaly detection models, based on vehicle data and fleet data, are developed. The proposed solution is a generic tool that doesn't make assumptions on data distribution and can be adapted to other systems by tweaking mainly the data cleaning process. It also incorporates specific system definitions of abnormal behavior, which makes it more accurate compared to conventional anomaly detection tools, which are mainly affected by the imbalanced data and the EVAP and purge definition of an anomaly. When deployed with field data, the algorithm showed higher performance, compared to popular anomaly detection techniques, and proved that failures can be prevented through detection of the anomalies several weeks/miles before the actual fail.
更多查看译文
关键词
machine learning,vehicle
AI 理解论文
溯源树
样例
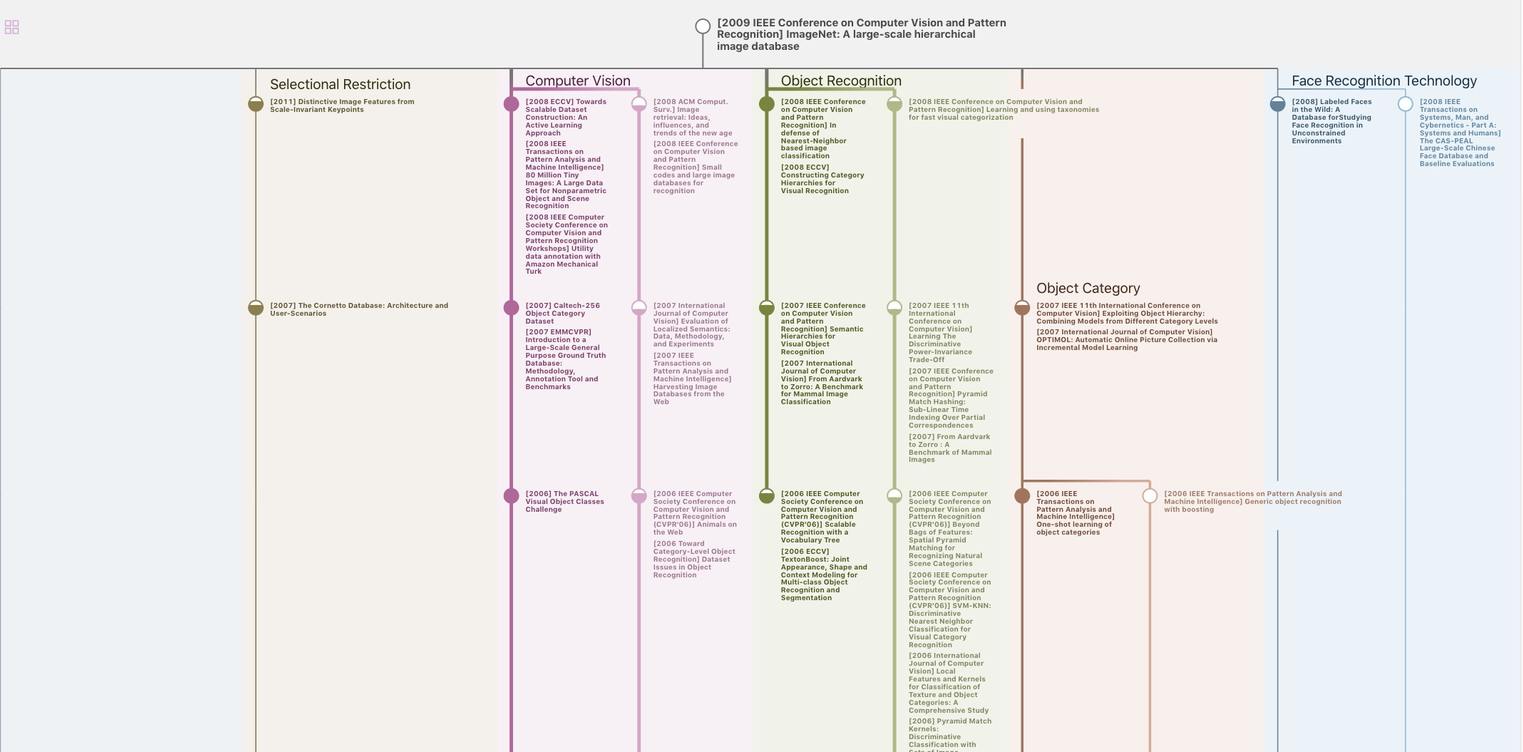
生成溯源树,研究论文发展脉络
Chat Paper
正在生成论文摘要