Audio-visual keyword transformer for unconstrained sentence-level keyword spotting
CAAI TRANSACTIONS ON INTELLIGENCE TECHNOLOGY(2024)
摘要
As one of the most effective methods to improve the accuracy and robustness of speech tasks, the audio-visual fusion approach has recently been introduced into the field of Keyword Spotting (KWS). However, existing audio-visual keyword spotting models are limited to detecting isolated words, while keyword spotting for unconstrained speech is still a challenging problem. To this end, an Audio-Visual Keyword Transformer (AVKT) network is proposed to spot keywords in unconstrained video clips. The authors present a transformer classifier with learnable CLS tokens to extract distinctive keyword features from the variable-length audio and visual inputs. The outputs of audio and visual branches are combined in a decision fusion module. As humans can easily notice whether a keyword appears in a sentence or not, our AVKT network can detect whether a video clip with a spoken sentence contains a pre-specified keyword. Moreover, the position of the keyword is localised in the attention map without additional position labels. Experimental results on the LRS2-KWS dataset and our newly collected PKU-KWS dataset show that the accuracy of AVKT exceeded 99% in clean scenes and 85% in extremely noisy conditions. The code is available at .
更多查看译文
关键词
artificial intelligence,multimodal approaches,natural language processing,neural network,speech processing
AI 理解论文
溯源树
样例
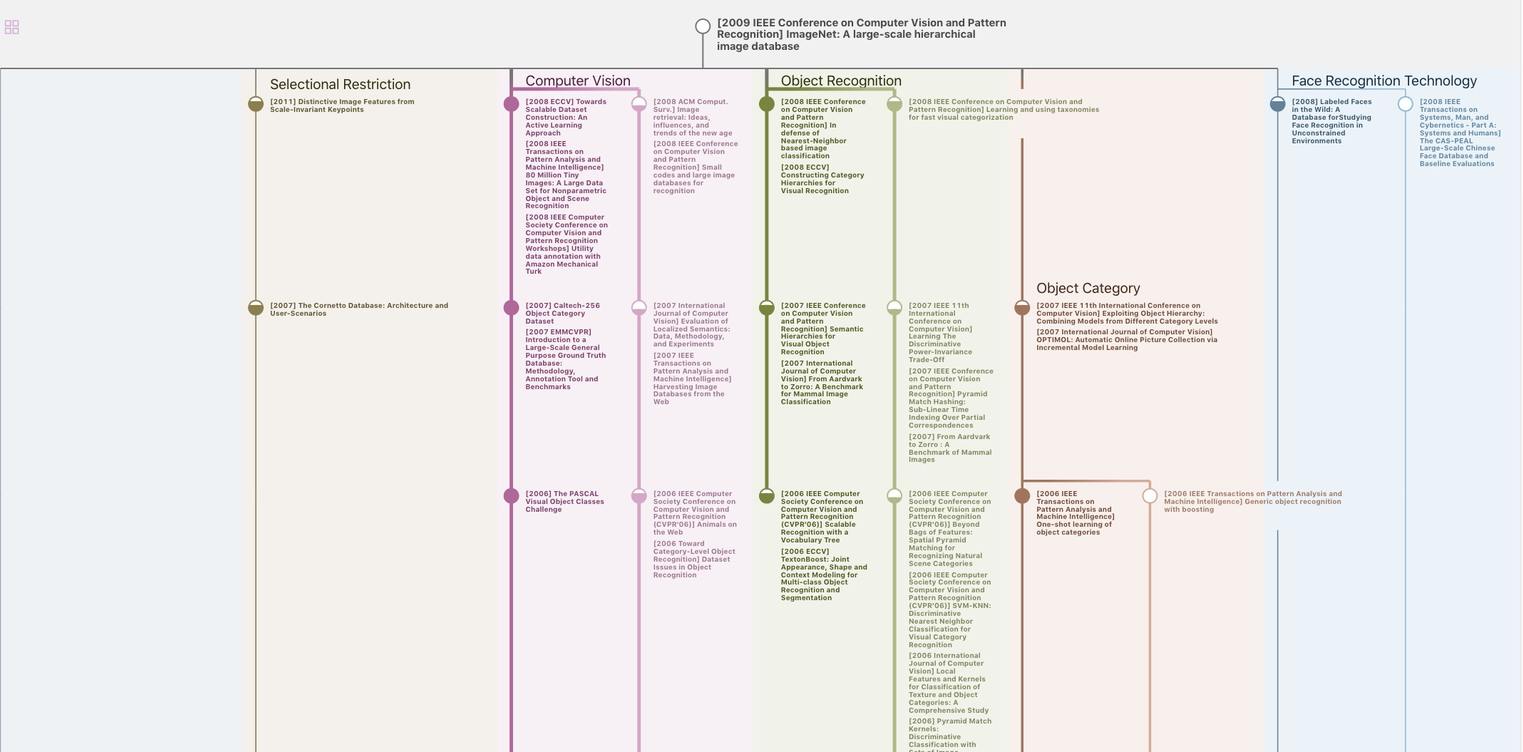
生成溯源树,研究论文发展脉络
Chat Paper
正在生成论文摘要