Meta-learning how to forecast time series
JOURNAL OF FORECASTING(2023)
摘要
Features of time series are useful in identifying suitable models for forecasting. We present a general framework, labelled Feature-based FORecast Model Selection (FFORMS), which selects forecast models based on features calculated from each time series. The FFORMS framework builds a mapping that relates the features of a time series to the "best" forecast model using a classification algorithm such as a random forest. The framework is evaluated using time series from the M-forecasting competitions and is shown to yield forecasts that are almost as accurate as state-of-the-art methods but are much faster to compute. We use model-agnostic machine learning interpretability methods to explore the results and to study what types of time series are best suited to each forecasting model.
更多查看译文
关键词
algorithm selection problem,black-box models,machine learning interpretability,random forest,visualization
AI 理解论文
溯源树
样例
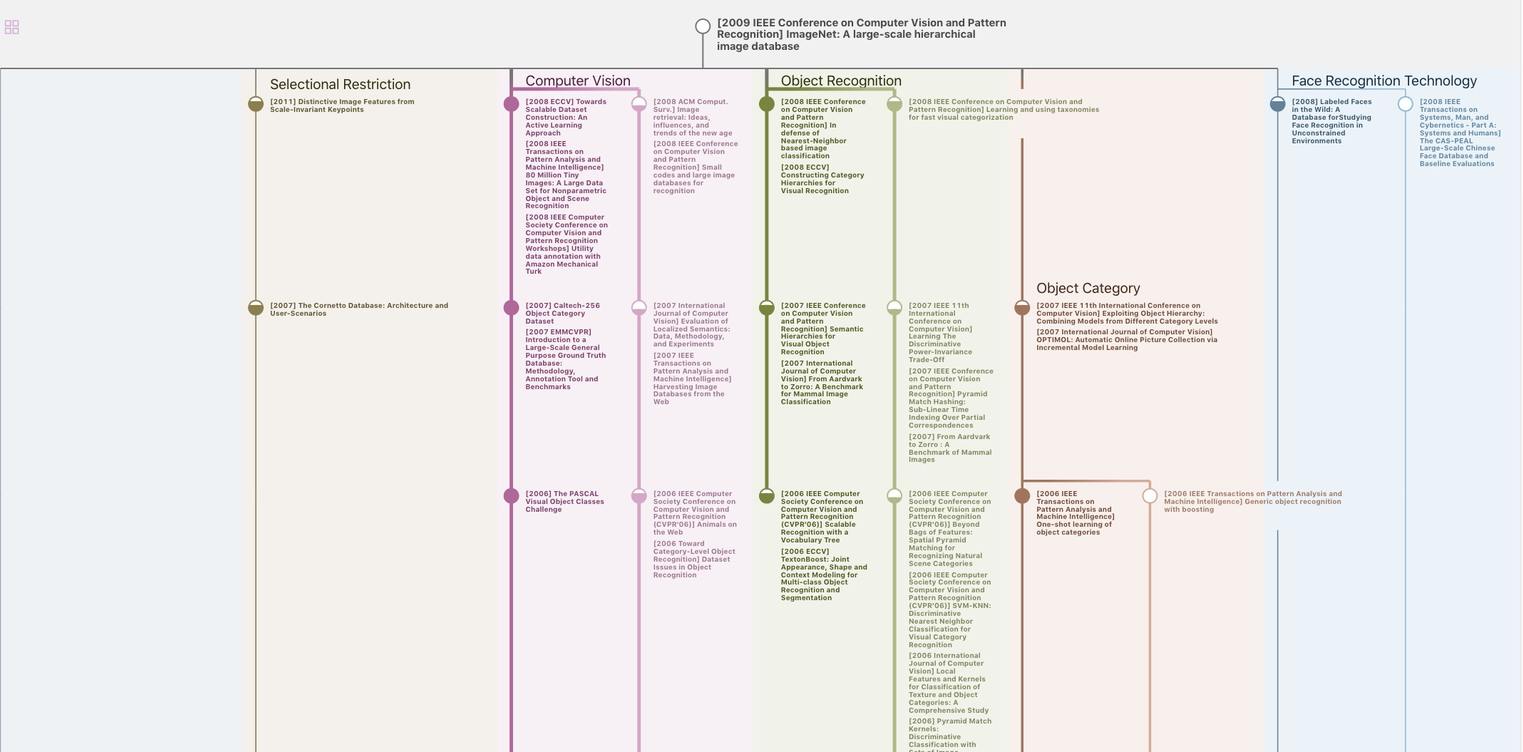
生成溯源树,研究论文发展脉络
Chat Paper
正在生成论文摘要