The Choice of Machine Learning Algorithms Impacts the Association between Brain-Predicted Age Difference and Cognitive Function
MATHEMATICS(2023)
摘要
Machine learning has been increasingly applied to neuroimaging data to compute personalized estimates of the biological age of an individual's brain (brain age). The difference between an individual's brain-predicted age and their chronological age (brainPAD) is used as a biomarker of brain aging and disease, but the potential contribution of different machine learning algorithms used for brain age prediction to the association between brainPAD and cognitive function has not been investigated yet. Here, we applied seven commonly used algorithms to the same multimodal brain imaging data (structural and diffusion MRI) from 601 healthy participants aged 18-88 years in the Cambridge Centre for Ageing and Neuroscience to assess variations in brain-predicted age. The inter-algorithm similarity in brain-predicted age and brain regional regression weights was examined using the Pearson's correlation analyses and hierarchical clustering. We then assessed to what extent machine learning algorithms impact the association between brainPAD and seven cognitive variables. The regression models achieved mean absolute errors of 5.46-7.72 years and Pearson's correlation coefficients of 0.86-0.92 between predicted brain age and chronological age. Furthermore, we identified a substantial difference in linking brainPAD to cognitive measures, indicating that the choice of algorithm could be an important source of variability that confounds the relationship between brainPAD and cognition.
更多查看译文
关键词
magnetic resonance imaging,diffusion magnetic resonance imaging,machine learning,brain age prediction,cognition
AI 理解论文
溯源树
样例
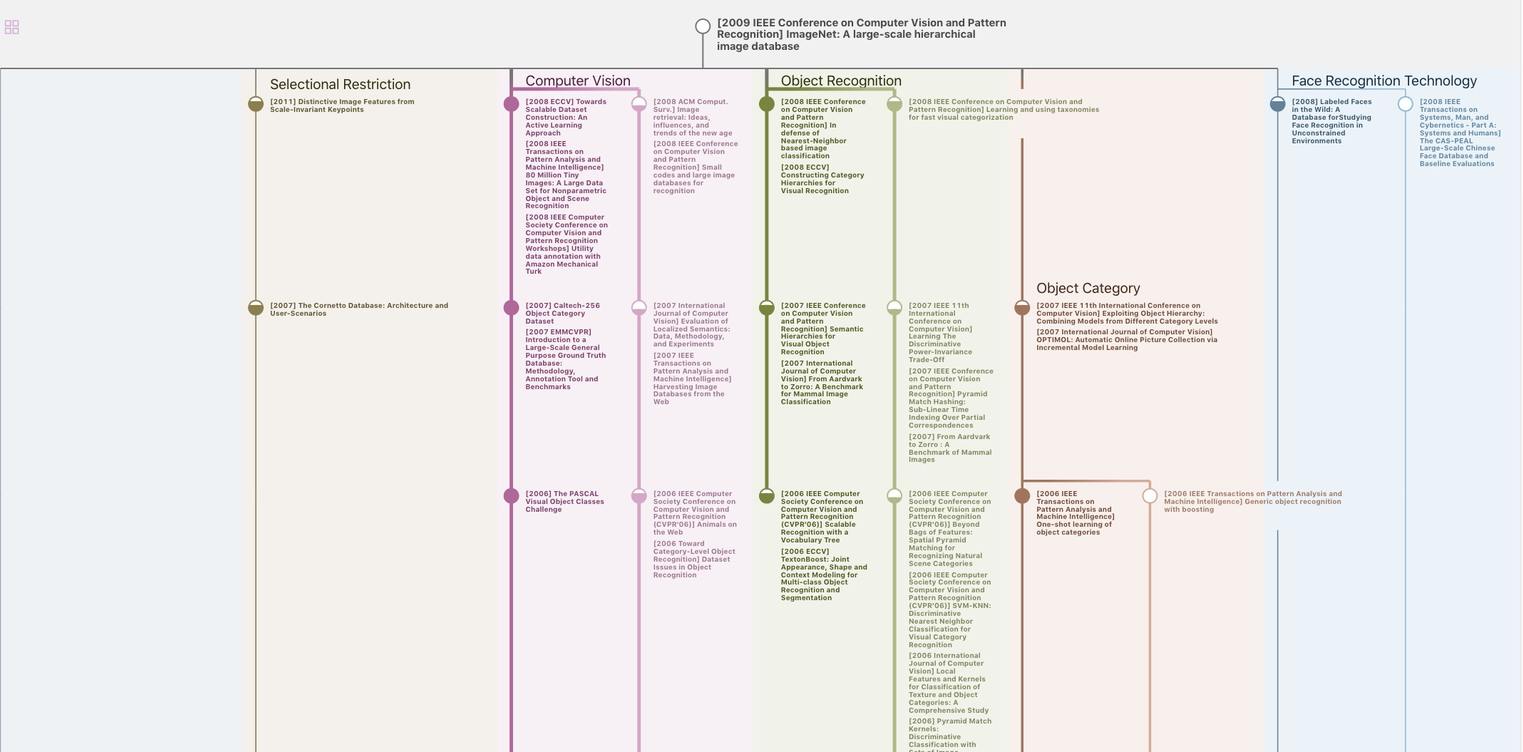
生成溯源树,研究论文发展脉络
Chat Paper
正在生成论文摘要