Handling categorical features with many levels using a product partition model
ANNALS OF APPLIED STATISTICS(2023)
摘要
A common difficulty in data analysis is how to handle categorical predictors with a large number of levels or categories. Few proposals have been developed to tackle this important and frequent problem. We introduce a generative model that simultaneously carries out the model fitting and the aggregation of the categorical levels into larger groups. We represent the categorical predictor by a graph where the nodes are the categories and establish a probability distribution over meaningful partitions of this graph. Conditionally on the observed data, we obtain a posterior distribution for the levels aggregation, allowing the inference about the most probable clustering for the categories. Simultaneously, we extract inference about all the other regression model parameters. We compare our and state-of-art methods showing that it has equally good predictive performance and more interpretable results. Our approach balances out accuracy vs. interpretability, a current important concern in statistics and machine learning.
更多查看译文
关键词
 ,Categorical predictors,clustering effects,random partition,dimension reduction,linear regression
AI 理解论文
溯源树
样例
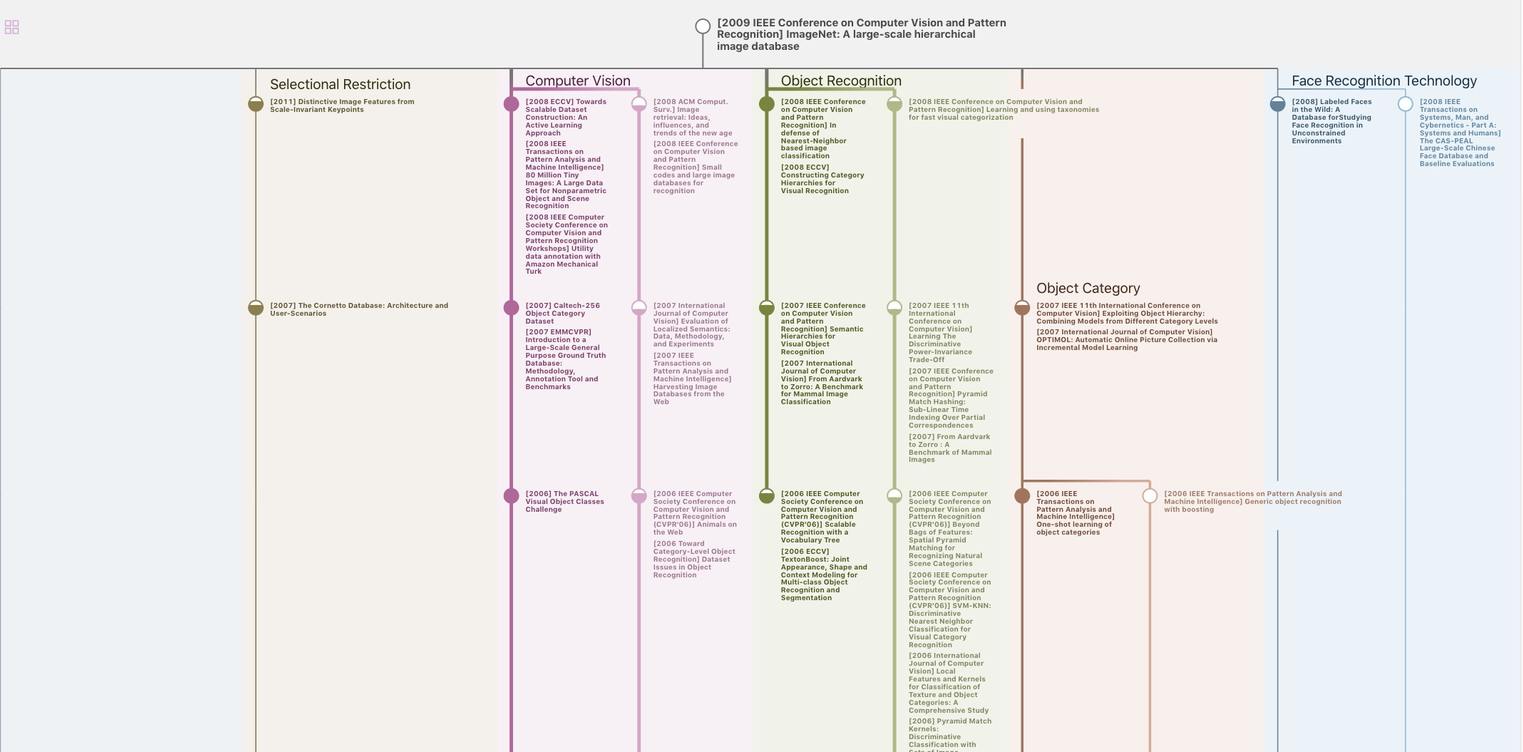
生成溯源树,研究论文发展脉络
Chat Paper
正在生成论文摘要