Improving performance of decision threshold moving-based strategies by integrating density-based clustering technique
ELECTRONIC RESEARCH ARCHIVE(2023)
摘要
Class imbalance learning (CIL), which aims to addressing the performance degradation problem of traditional supervised learning algorithms in the scenarios of skewed data distribution, has become one of research hotspots in fields of machine learning, data mining, and artificial intelligence. As a postprocessing CIL technique, the decision threshold moving (DTM) has been verified to be an effective strategy to address class imbalance problem. However, no matter adopting random or optimal threshold designation ways, the classification hyperplane could be only moved parallelly, but fails to vary its orientation, thus its performance is restricted, especially on some complex and density variable data. To further improve the performance of the existing DTM strategies, we propose an improved algorithm called CDTM by dividing majority training instances into multiple different density regions, and further conducting DTM procedure on each region independently. Specifically, we adopt the well-known DBSCAN clustering algorithm to split training set as it could adapt density variation well. In context of support vector machine (SVM) and extreme learning machine (ELM), we respectively verified the effectiveness and superiority of the proposed CDTM algorithm. The experimental results on 40 benchmark class imbalance datasets indicate that the proposed CDTM algorithm is superior to several other state-of-the-art DTM algorithms in term of G-mean performance metric.
更多查看译文
关键词
class imbalance learning,decision threshold moving,clustering,DBSCAN
AI 理解论文
溯源树
样例
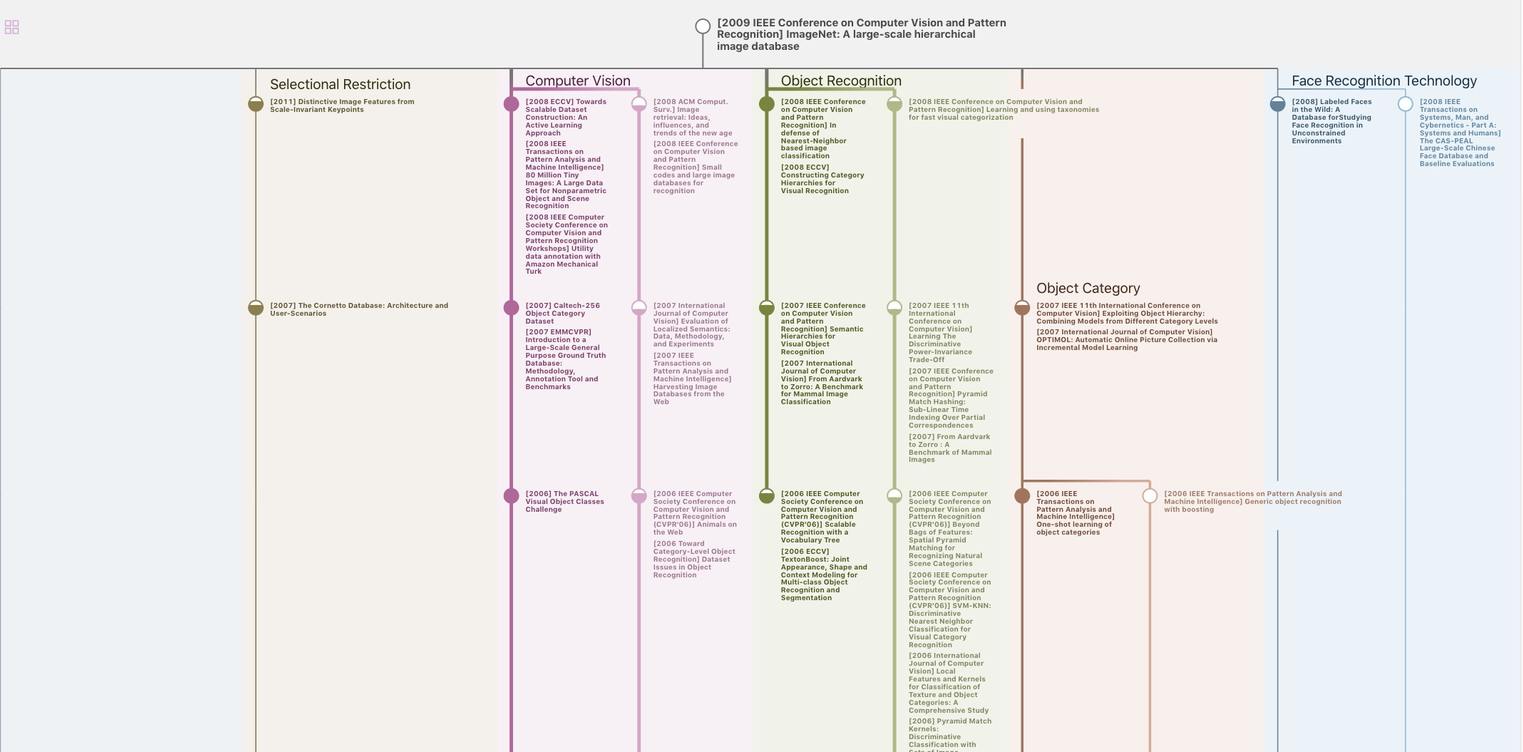
生成溯源树,研究论文发展脉络
Chat Paper
正在生成论文摘要