Quantitative Reconstruction of Dielectric Properties Based on Deep-Learning-Enabled Microwave-Induced Thermoacoustic Tomography
IEEE TRANSACTIONS ON MICROWAVE THEORY AND TECHNIQUES(2023)
摘要
Quantitative reconstruction of dielectric properties has enabled a wealth of biomedical applications. Although traditional microwave imaging and microwave-induced thermoacoustic tomography (MITAT) techniques have been widely explored for quantitative reconstruction, it is still highly challenging for them to deal with biological samples with high permittivity and conductivity. This work leverages deep-learning-enabled MITAT (DL-MITAT) approach to quantitatively reconstruct dielectric properties of biological samples with high quality. We construct a new network structure to separately reconstruct the permittivity and conductivity. By simulation and experimental testing, we demonstrate that the DL-MITAT technique is able to reliably reconstruct inhomogeneous biological samples with tumor, muscle, and fat. The experimental reconstruction error is only 5%. The network exhibits excellent generalization capability in terms of sample's geometry. This work provides a useful paradigm and alternative way for quantitative reconstruction of dielectric properties and paves the way toward practical applications.
更多查看译文
关键词
Image reconstruction,Microwave theory and techniques,Microwave imaging,Dielectrics,Acoustics,Permittivity,Conductivity,Deep learning (DL),dielectric property reconstruction,microwave imaging,microwave-induced thermoacoustic tomography (MITAT),quantitative reconstruction
AI 理解论文
溯源树
样例
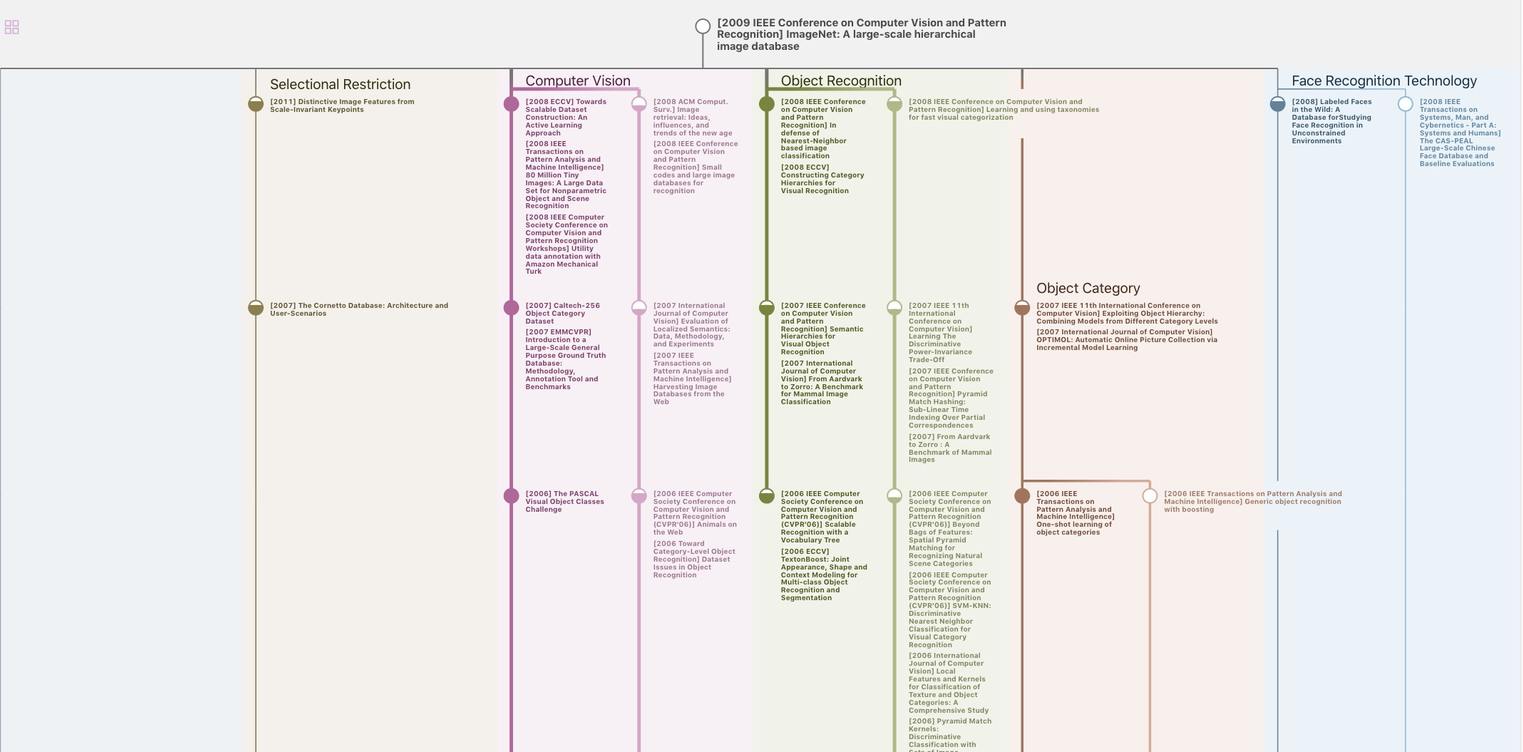
生成溯源树,研究论文发展脉络
Chat Paper
正在生成论文摘要