Investigating paired comparisons after principal component analysis
FOOD QUALITY AND PREFERENCE(2023)
摘要
Principal component analysis (PCA) is often used to explore sensory and consumer test data about products on multicollinear sensory attributes. In this paper, we propose an approach for investigating paired comparisons between products and their uncertainties in the principal components. We use the truncated total bootstrap (TTB) procedure to simulate virtual panels from the original data set. The virtual-panel results are then used to investigate the uncertainty of the paired comparisons. To justify the proposal, we provide an appendix that contains theoretical contributions. We introduce a new ("crossdiff") matrix operator for obtaining paired com-parisons and a procedure called crossdiff-unfolding. The notation and procedure facilitate proofs that help to justify investigating paired comparisons in the same space as the products. Specifically, we show that the principal components that optimally extract variance from the objects (e.g. products in the original coordinate system of sensory variables) also optimally extract variance from all paired comparisons. Also, we demonstrate that the same Procrustes rotation matrix that optimally superimposes a score matrix onto a target score matrix also optimally superimposes a matrix of crossdiff-unfolded paired difference scores onto a target matrix of crossdiff-unfolded paired difference scores. We account for mutual dependencies in TTB-derived paired differ-ence scores, then visualize these results along with contours of their kernel-estimated densities. We apply the proposed methods to two data sets, one from quantitative sensory descriptive analysis from a trained panel and one from check-all-that-apply (CATA) data from a consumer cluster. Practical advantages of the methods for practitioners are described. PCA is used widely in sensory and consumer science to communicate results, and the proposed tools can strengthen interpretability.
更多查看译文
关键词
Principal component analysis (PCA),Paired comparison,Procrustes rotation,Sensory evaluation,Sensory profiling,Bootstrap
AI 理解论文
溯源树
样例
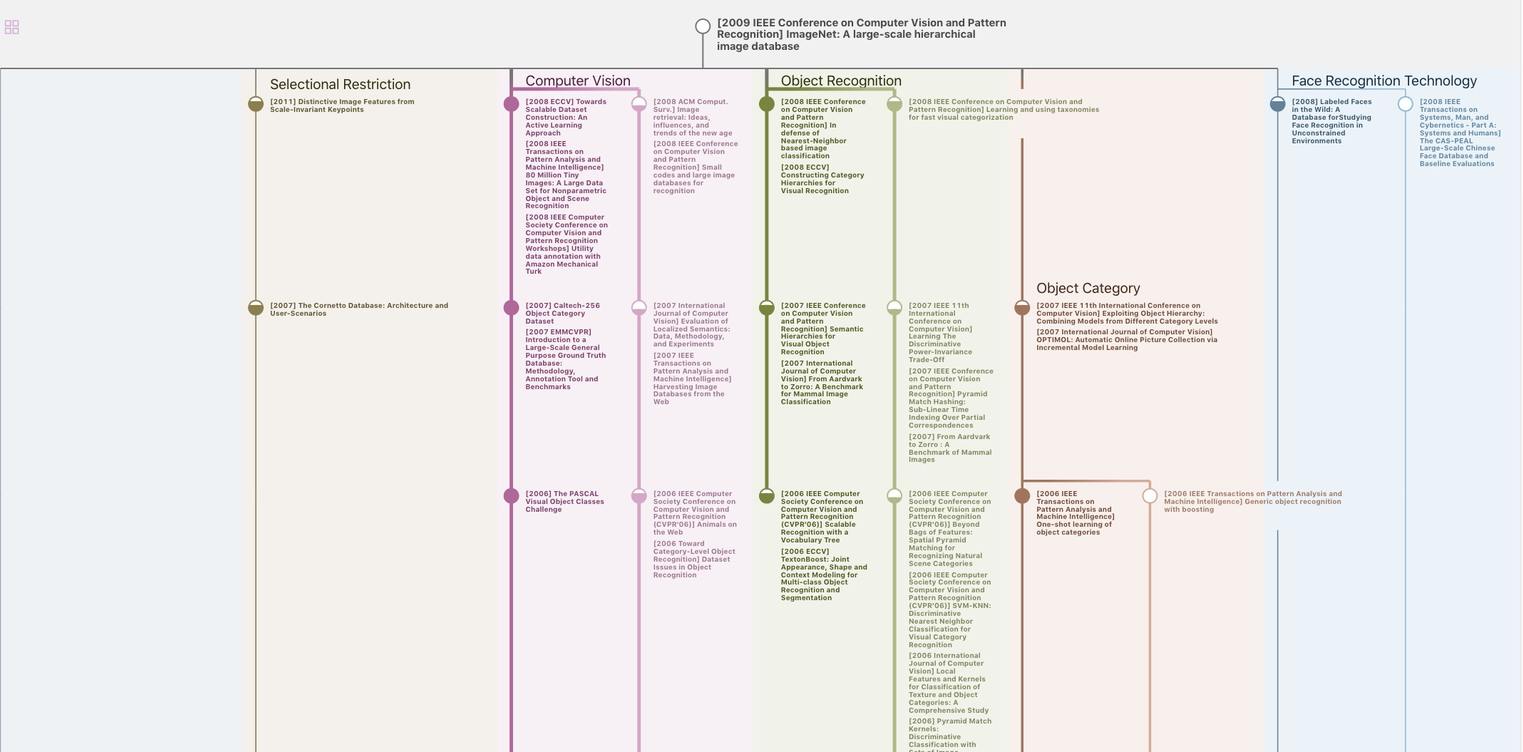
生成溯源树,研究论文发展脉络
Chat Paper
正在生成论文摘要