Self-Supervised Representation Learning for Geographical Data-A Systematic Literature Review
ISPRS INTERNATIONAL JOURNAL OF GEO-INFORMATION(2023)
摘要
Self-supervised representation learning (SSRL) concerns the problem of learning a useful data representation without the requirement for labelled or annotated data. This representation can, in turn, be used to support solutions to downstream machine learning problems. SSRL has been demonstrated to be a useful tool in the field of geographical information science (GIS). In this article, we systematically review the existing research literature in this space to answer the following five research questions. What types of representations were learnt? What SSRL models were used? What downstream problems were the representations used to solve? What machine learning models were used to solve these problems? Finally, does using a learnt representation improve the overall performance?
更多查看译文
关键词
geographical data,self-supervised representation learning,systematic literature review
AI 理解论文
溯源树
样例
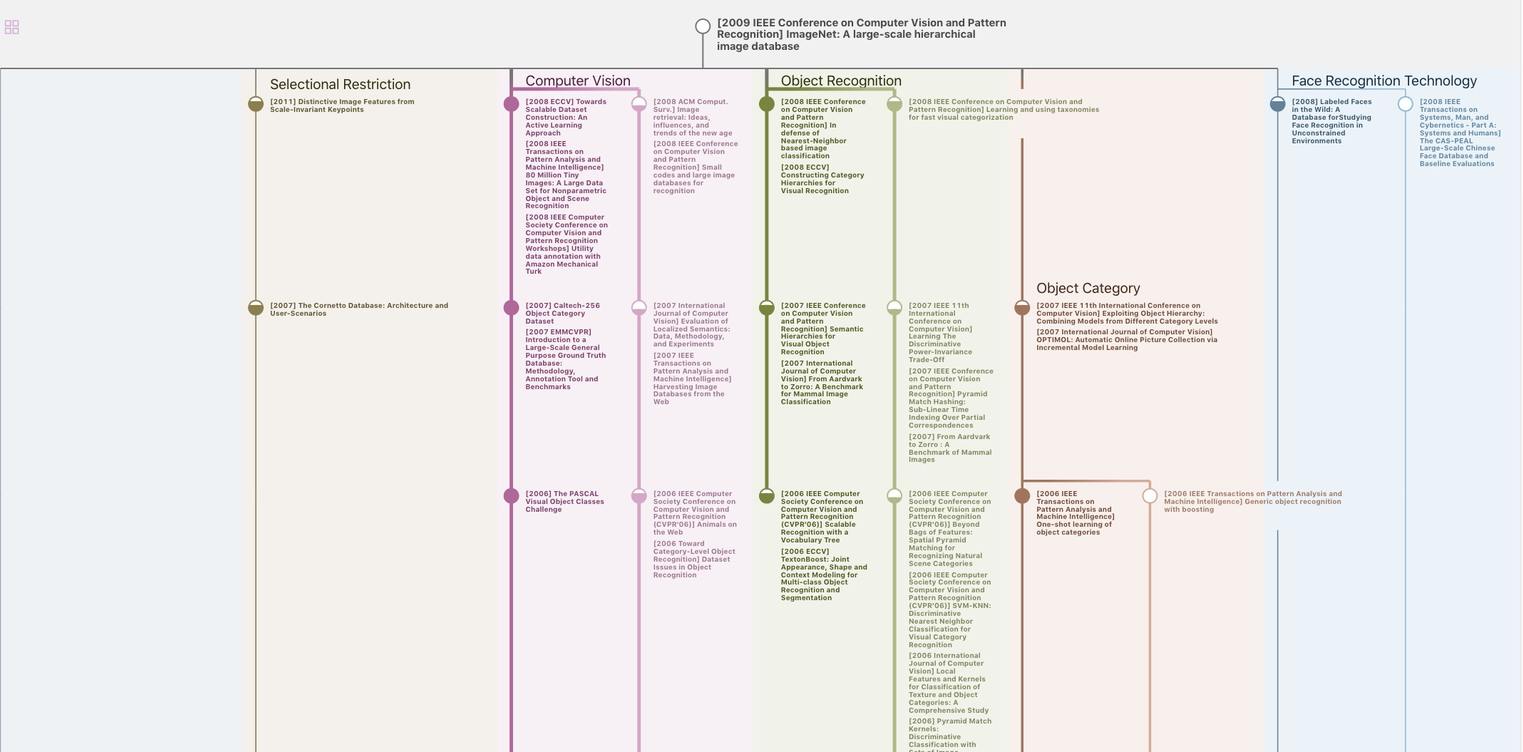
生成溯源树,研究论文发展脉络
Chat Paper
正在生成论文摘要