Secure Multi-Party Computation for Personalized Human Activity Recognition
NEURAL PROCESSING LETTERS(2023)
摘要
Calibrating Human Activity Recognition (HAR) models to end-users with Transfer Learning (TL) often yields significant accuracy improvements. Such TL is by design done based on very personal data collected by sensors worn close to the human body. To protect the users’ privacy, we therefore introduce Secure Multi-Party Computation (MPC) protocols for personalization of HAR models, and for secure activity recognition with the personalized models. Our MPC protocols do not require the end-users to reveal their sensitive data in an unencrypted manner, nor do they require the application developer to disclose their trained model parameters or any other sensitive or proprietary information with anyone in plaintext. Through experiments on HAR benchmark datasets, we demonstrate that our privacy-preserving solution yields the same accuracy gains as TL in-the-clear, i.e. when no measures to protect privacy are in place, and that our approach is fast enough for use in practice.
更多查看译文
关键词
Transfer learning,Human activity recognition,Convolutional neural network,Secure multi-party computation,Cryptography,Privacy
AI 理解论文
溯源树
样例
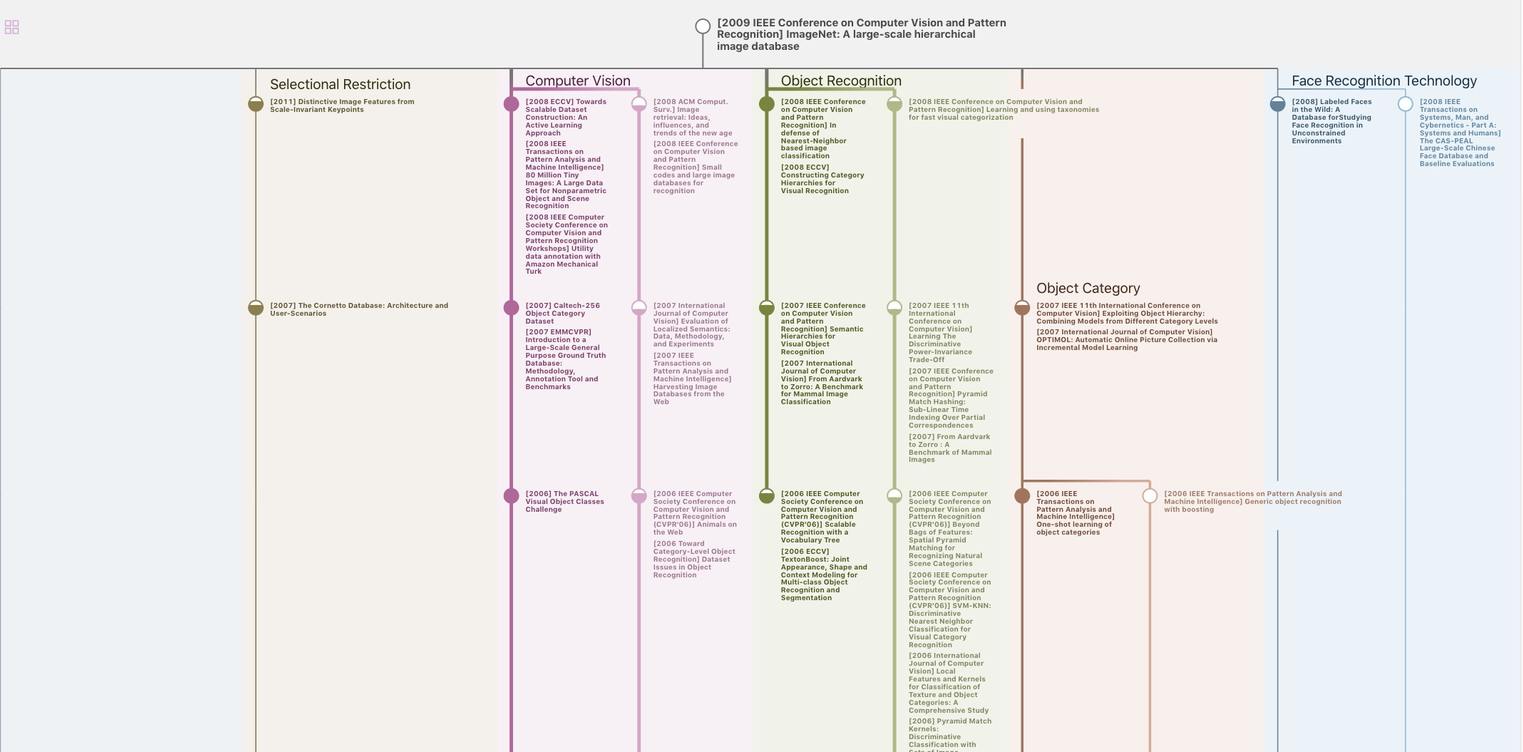
生成溯源树,研究论文发展脉络
Chat Paper
正在生成论文摘要