Synthesizing 3D Gait Data with Personalized Walking Style and Appearance
APPLIED SCIENCES-BASEL(2023)
摘要
Extracting gait biometrics from videos has been receiving rocketing attention given its applications, such as person re-identification. Although deep learning arises as a promising solution to improve the accuracy of most gait recognition algorithms, the lack of enough training data becomes a bottleneck. One of the solutions to address data deficiency is to generate synthetic data. However, gait data synthesis is particularly challenging as the inter-subject and intra-subject variations of walking style need to be carefully balanced. In this paper, we propose a complete 3D framework to synthesize unlimited, realistic, and diverse motion data. In addition to walking speed and lighting conditions, we emphasize two key factors: 3D gait motion style and character appearance. Benefiting from its 3D nature, our system can provide various gait-related data, such as accelerometer data and depth map, not limited to silhouettes. We conducted various experiments using the off-the-shelf gait recognition algorithm and draw the following conclusions: (1) the real-to-virtual gap can be closed when adding a small portion of real-world data to a synthetically trained recognizer; (2) the amount of real training data needed to train competitive gait recognition systems can be reduced significantly; (3) the rich variations in gait data are helpful for investigating algorithm performance under different conditions. The synthetic data generator, as well as all experiments, will be made publicly available.
更多查看译文
关键词
3D gait data,human motion data,neural network,gait recognition,gait synthesis
AI 理解论文
溯源树
样例
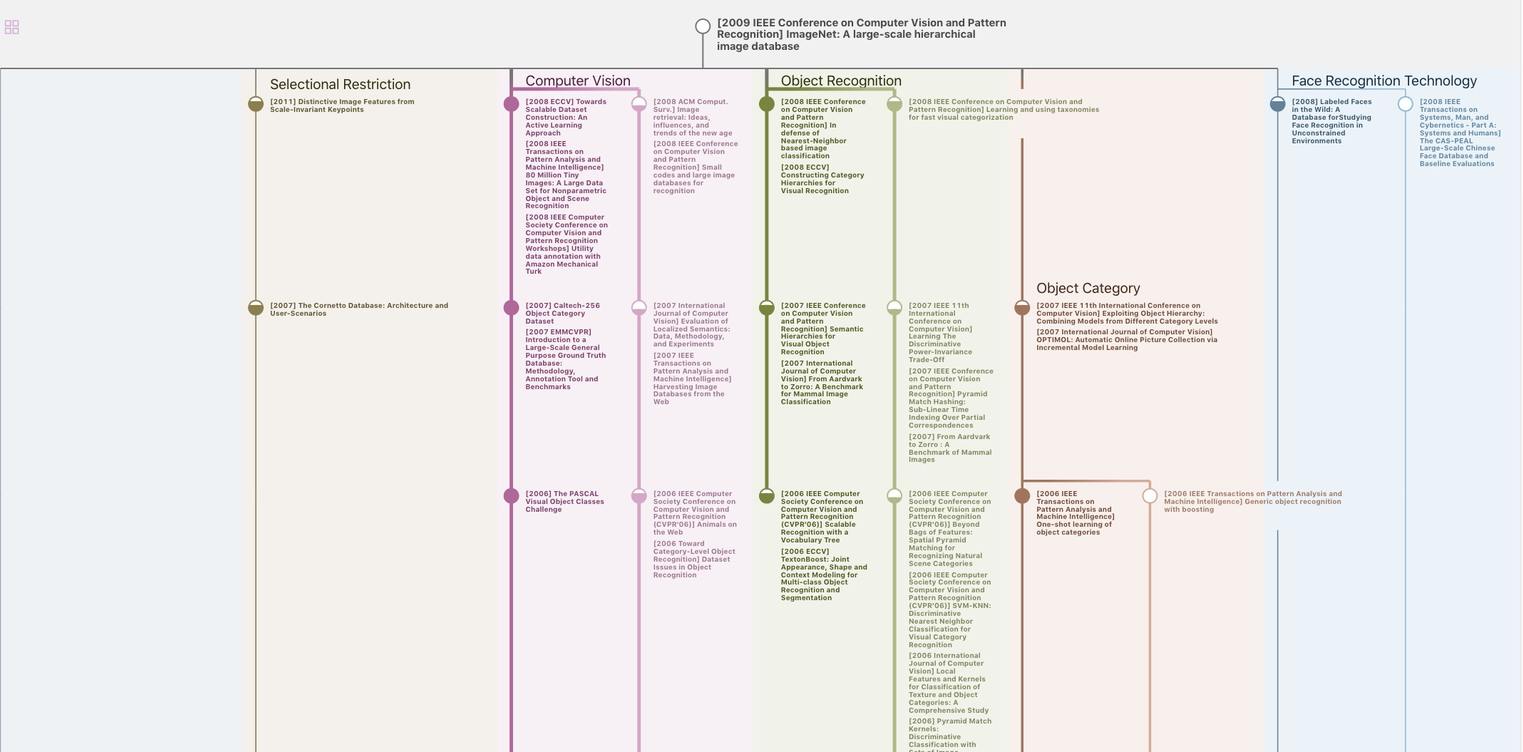
生成溯源树,研究论文发展脉络
Chat Paper
正在生成论文摘要