Outlier-resistant variance-constrained 𝐻_∞ state estimation for time-varying recurrent neural networks with randomly occurring deception attacks
NEURAL COMPUTING & APPLICATIONS(2023)
摘要
This paper investigates the outlier-resistant variance-constrained H_∞ state estimation problem for a class of discrete time-varying recurrent neural networks with randomly occurring deception attacks. The randomly occurring deception attacks are modeled by a series of random variables satisfying the Bernoulli distribution with known probability. In addition, the saturation function is introduced to reduce the negative impact from the measurement outliers onto the estimation performance. The objective of this paper is to propose an outlier-resistant finite-horizon state estimation scheme without utilizing the augmentation method such that, in the presence of measurement outliers and randomly occurring deception attacks, some sufficient criteria are obtained ensuring both the desired H_∞ performance index and the error variance boundedness. Finally, a numerical example is used to illustrate the feasibility of the presented outlier-resistant variance-constrained H_∞ state estimation algorithm.
更多查看译文
关键词
\documentclass[12pt]{minimal}
\usepackage{amsmath}
\usepackage{wasysym}
\usepackage{amsfonts}
\usepackage{amssymb}
\usepackage{amsbsy}
\usepackage{mathrsfs}
\usepackage{upgreek}
\setlength{\oddsidemargin}{-69pt}
\begin{document}$$H_{\infty }$$\end{document} performance
AI 理解论文
溯源树
样例
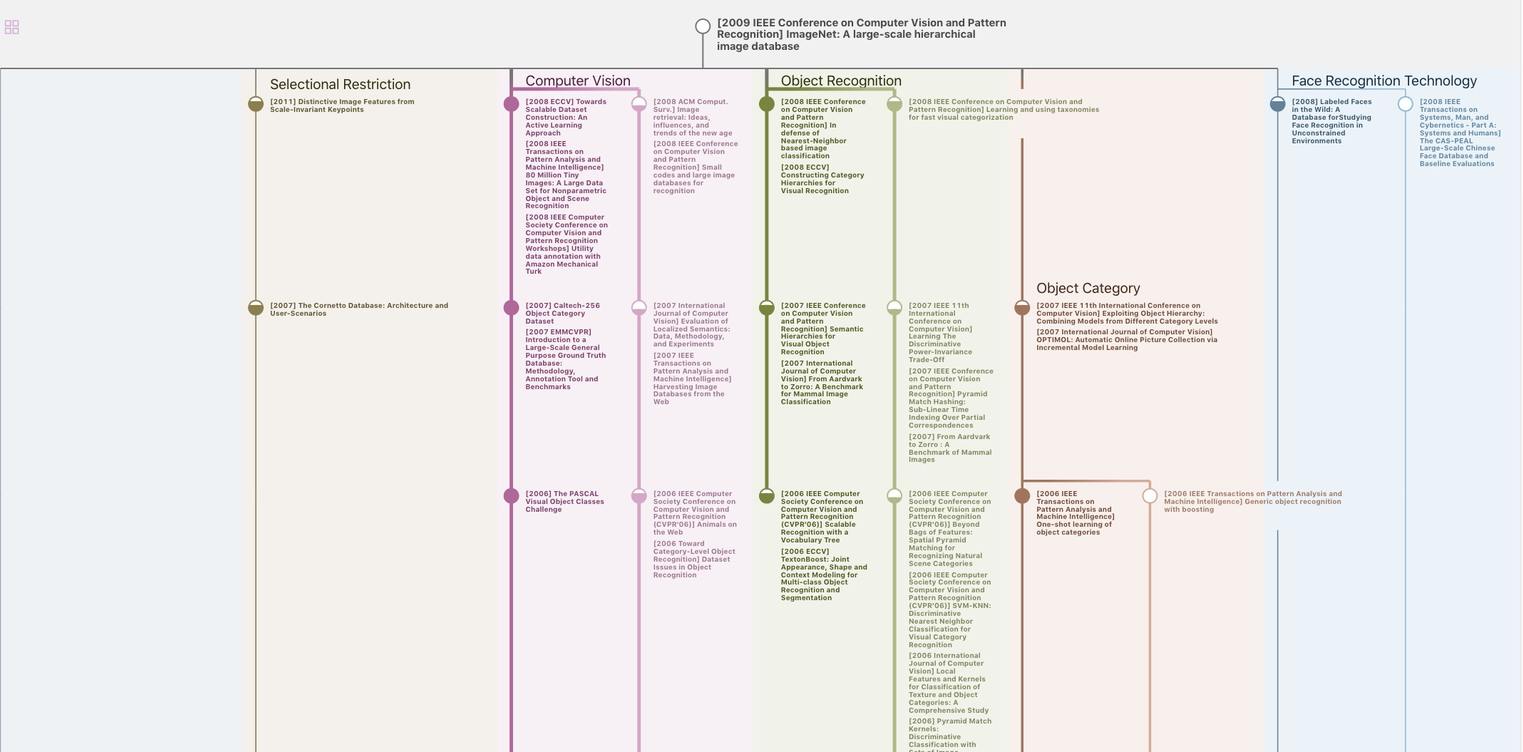
生成溯源树,研究论文发展脉络
Chat Paper
正在生成论文摘要