A convergence algorithm for graph co-regularized transfer learning
SCIENCE CHINA-INFORMATION SCIENCES(2023)
摘要
Transfer learning is an important technology in addressing the problem that labeled data in a target domain are difficult to collect using extensive labeled data from the source domain. Recently, an algorithm named graph co-regularized transfer learning (GTL) has shown a competitive performance in transfer learning. However, its convergence is affected by the used approximate scheme, degenerating learned results. In this paper, after analyzing convergence conditions, we propose a novel update rule using the multiplicative update rule and develop a new algorithm named improved GTL (IGTL) with a strict convergence guarantee. Moreover, to prove the convergence of our method, we design a special auxiliary function whose value is intimately related to that of the objective function. Finally, the experimental results on the synthetic dataset and two real-world datasets confirm that the proposed IGTL is convergent and performs better than the compared methods.
更多查看译文
关键词
transfer learning,convergence analysis,non-negative matrix factorization,multiplicative update algorithm,optimization
AI 理解论文
溯源树
样例
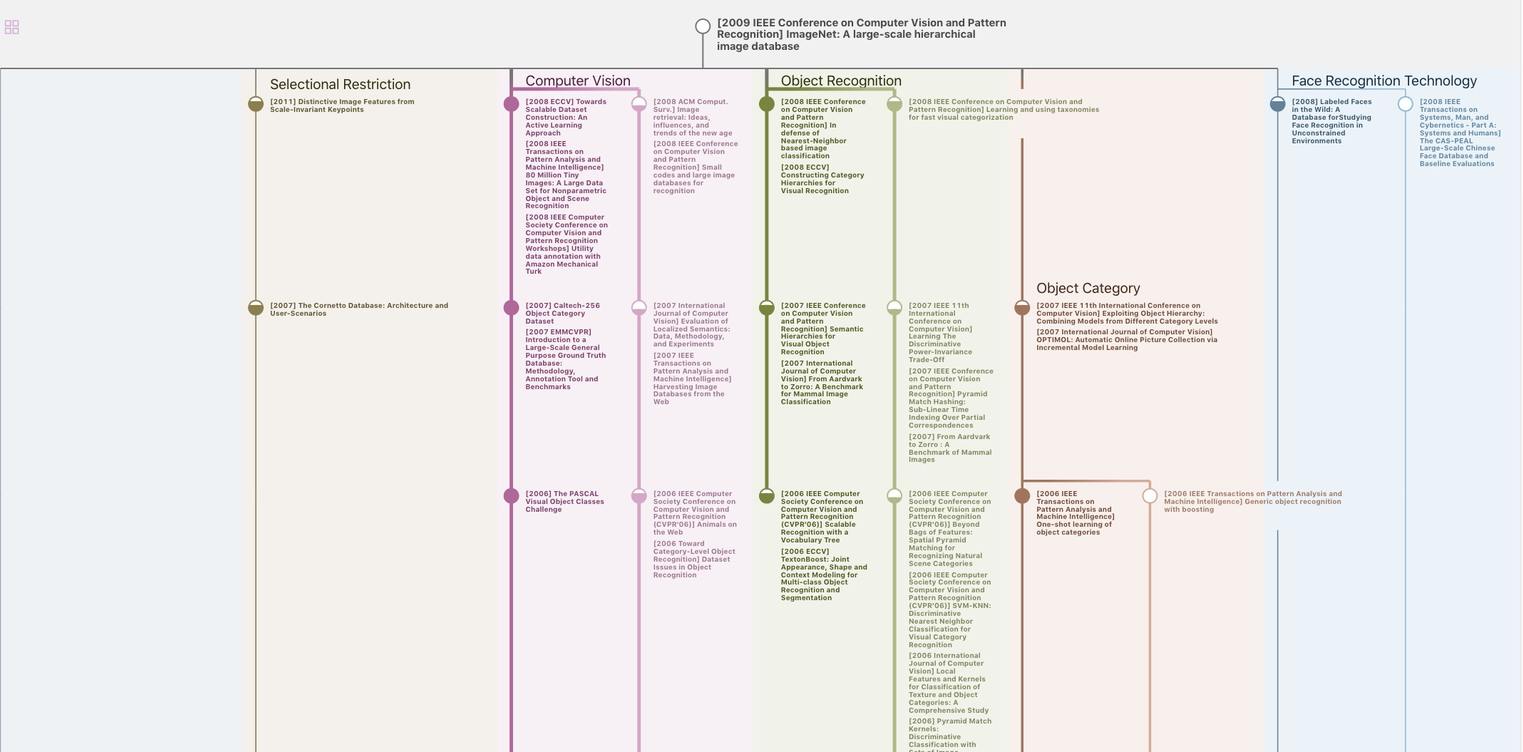
生成溯源树,研究论文发展脉络
Chat Paper
正在生成论文摘要