A Framework of Deep Optimal Features Selection for Apple Leaf Diseases Recognition
CMC-COMPUTERS MATERIALS & CONTINUA(2023)
摘要
Identifying fruit disease manually is time-consuming, expert -required, and expensive; thus, a computer-based automated system is widely required. Fruit diseases affect not only the quality but also the quantity. As a result, it is possible to detect the disease early on and cure the fruits using computer-based techniques. However, computer-based methods face several challenges, including low contrast, a lack of dataset for training a model, and inappropriate feature extraction for final classification. In this paper, we proposed an automated framework for detecting apple fruit leaf diseases using CNN and a hybrid optimization algorithm. Data augmentation is performed initially to balance the selected apple dataset. After that, two pre-trained deep models are fine-tuning and trained using transfer learning. Then, a fusion technique is proposed named Parallel Correlation Threshold (PCT). The fused feature vector is optimized in the next step using a hybrid optimization algorithm. The selected features are finally classified using machine learning algorithms. Four different experiments have been carried out on the augmented Plant Village dataset and yielded the best accuracy of 99.8%. The accuracy of the proposed framework is also compared to that of several neural nets, and it outperforms them all.
更多查看译文
关键词
Convolutional neural networks,deep learning,features fusion,features optimization,classification
AI 理解论文
溯源树
样例
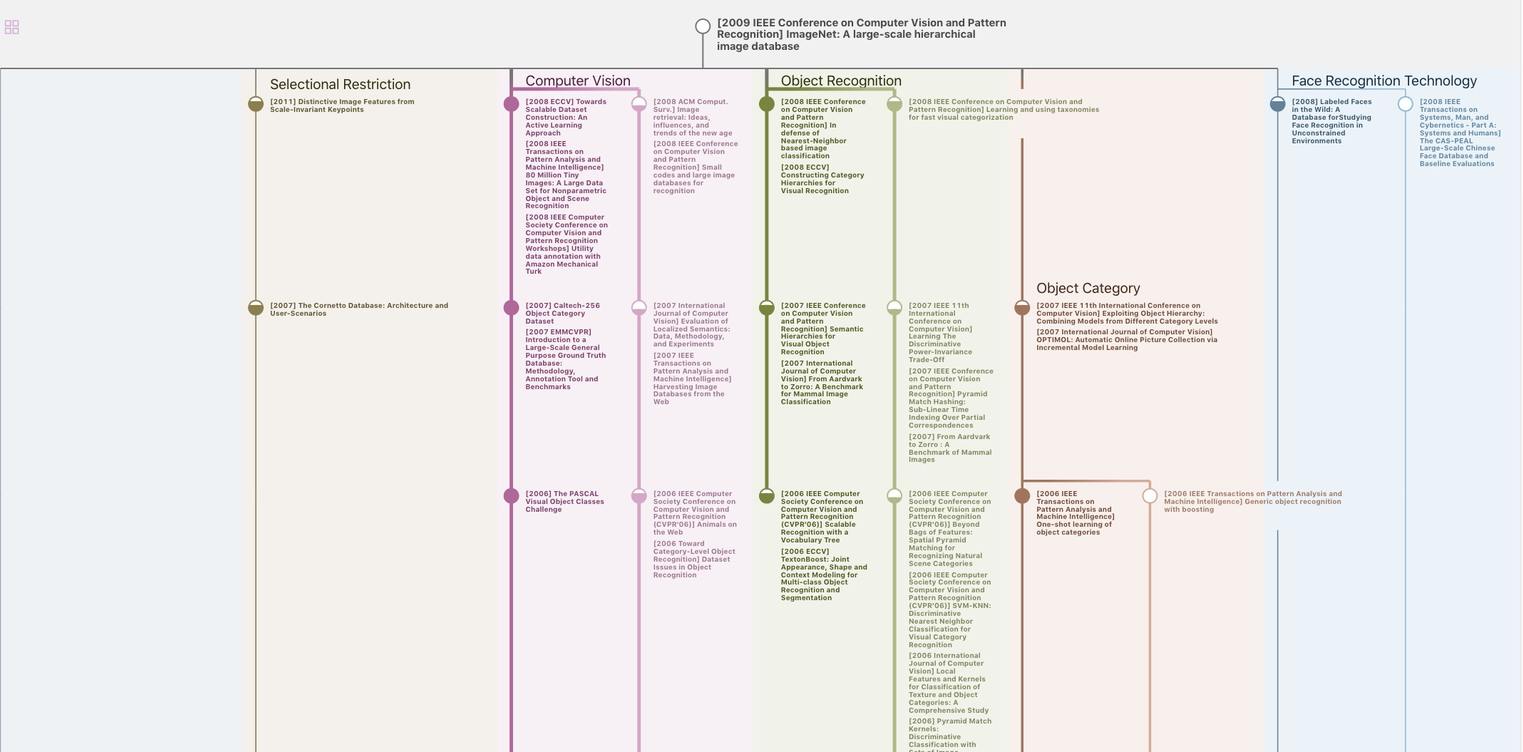
生成溯源树,研究论文发展脉络
Chat Paper
正在生成论文摘要