A Fixed-Time Noise-Tolerance ZNN Model for Time-Variant Inequality-Constrained Quaternion Matrix Least-Squares Problem
IEEE TRANSACTIONS ON NEURAL NETWORKS AND LEARNING SYSTEMS(2023)
摘要
Presently, numerical algorithms for solving quaternion least-squares problems have been intensively studied and utilized in various disciplines. However, they are unsuitable for solving the corresponding time-variant problems, and thus few studies have explored the solution to the time-variant inequality-constrained quaternion matrix least-squares problem (TVIQLS). To do so, this article designs a fixed-time noise-tolerance zeroing neural network (FTNTZNN) model to determine the solution of the TVIQLS in a complex environment by exploiting the integral structure and the improved activation function (AF). The FTNTZNN model is immune to the effects of initial values and external noise, which is much superior to the conventional zeroing neural network (CZNN) models. Besides, detailed theoretical derivations about the global stability, the fixed-time (FXT) convergence, and the robustness of the FTNTZNN model are provided. Simulation results indicate that the FTNTZNN model has a shorter convergence time and superior robustness compared to other zeroing neural network (ZNN) models activated by ordinary AFs. At last, the construction method of the FTNTZNN model is successfully applied to the synchronization of Lorenz chaotic systems (LCSs), which shows the practical application value of the FTNTZNN model.
更多查看译文
关键词
Quaternions,Mathematical models,Convergence,Numerical models,Computational modeling,Robustness,Neural networks,Fixed-time (FXT) convergence,robustness,time-variant quaternion matrix least-squares problem,zeroing neural network (ZNN)
AI 理解论文
溯源树
样例
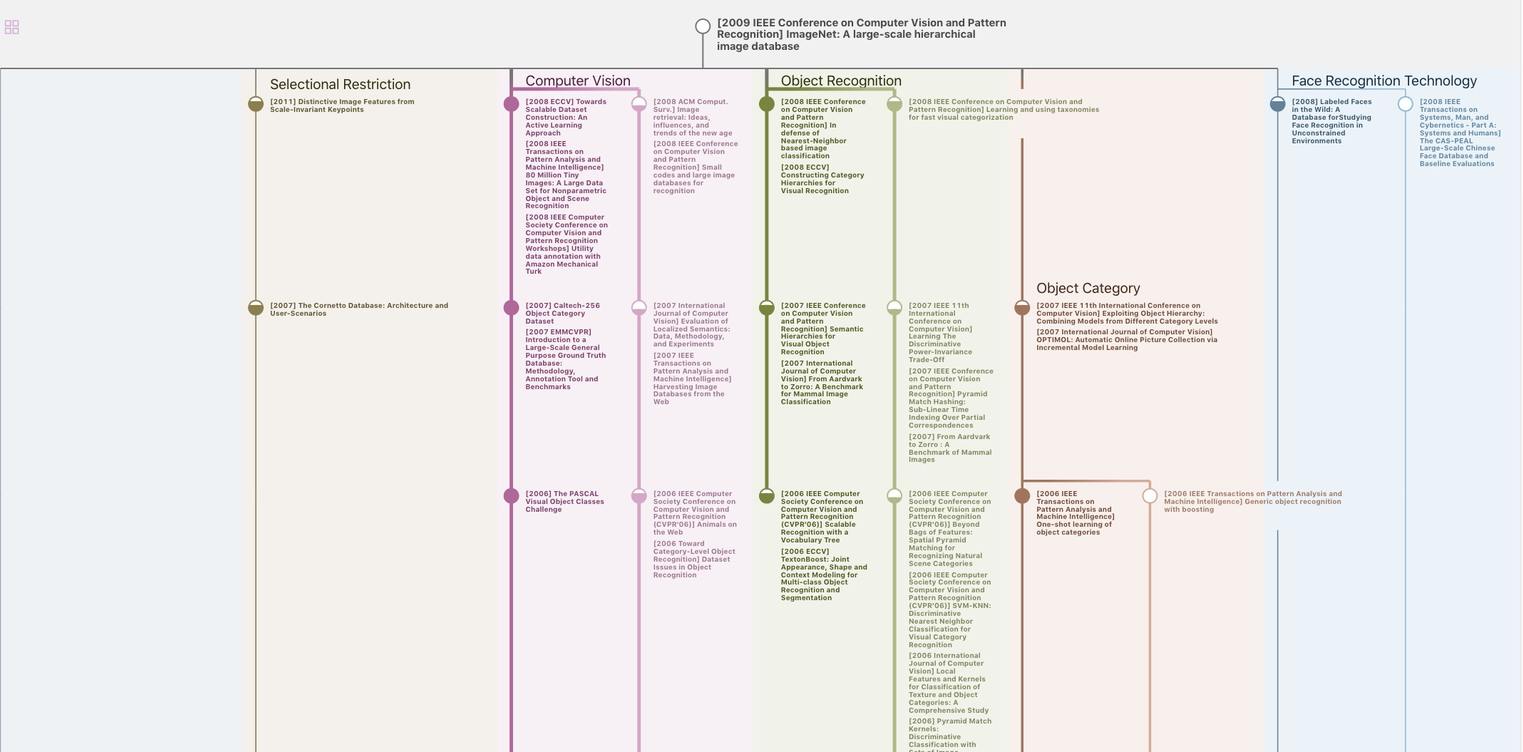
生成溯源树,研究论文发展脉络
Chat Paper
正在生成论文摘要