Asymmetric Supervised Fusion-Oriented Hashing for Cross-Modal Retrieval
IEEE TRANSACTIONS ON CYBERNETICS(2024)
摘要
Hashing technologies have been widely applied for large-scale multimodal retrieval tasks owing to their excellent performance in search and storage tasks. Although some effective hashing methods have been proposed, it is still difficult to handle the intrinsic linkages that exist among different heterogeneous modalities. Moreover, optimizing the discrete constraint problem through a relaxation-based strategy results in a large quantization error and leads to a suboptimal solution. In this article, we present a novel asymmetric supervised fusion-oriented hashing method, named (ASFOH), which investigates three novel schemes to remedy the above issues. Specifically, we first explicitly formulate the problem as matrix decomposition into a common latent representation and a transformation matrix, combined with an adaptive weight scheme and nuclear norm minimization to ensure the information completeness of multimodal data. Then, we associate the common latent representation with the semantic label matrix, thereby increasing the discriminative capability of the model by constructing an asymmetric hash learning framework, thus, making the generated hash codes more compact. Finally, an efficient discrete optimization iterative algorithm based on nuclear norm minimization is proposed to decompose the nonconvex multivariate optimization problem into several subproblems with analytical solutions. Comprehensive experiments on the MIRFlirck, NUS-WIDE, and IARP-TC12 datasets testify that ASFOH outperforms the compared state-of-the-art approaches.
更多查看译文
关键词
Semantics,Matrix decomposition,Binary codes,Optimization,Couplings,Task analysis,Minimization,Cross-modal retrieval,discrete optimization,hash
AI 理解论文
溯源树
样例
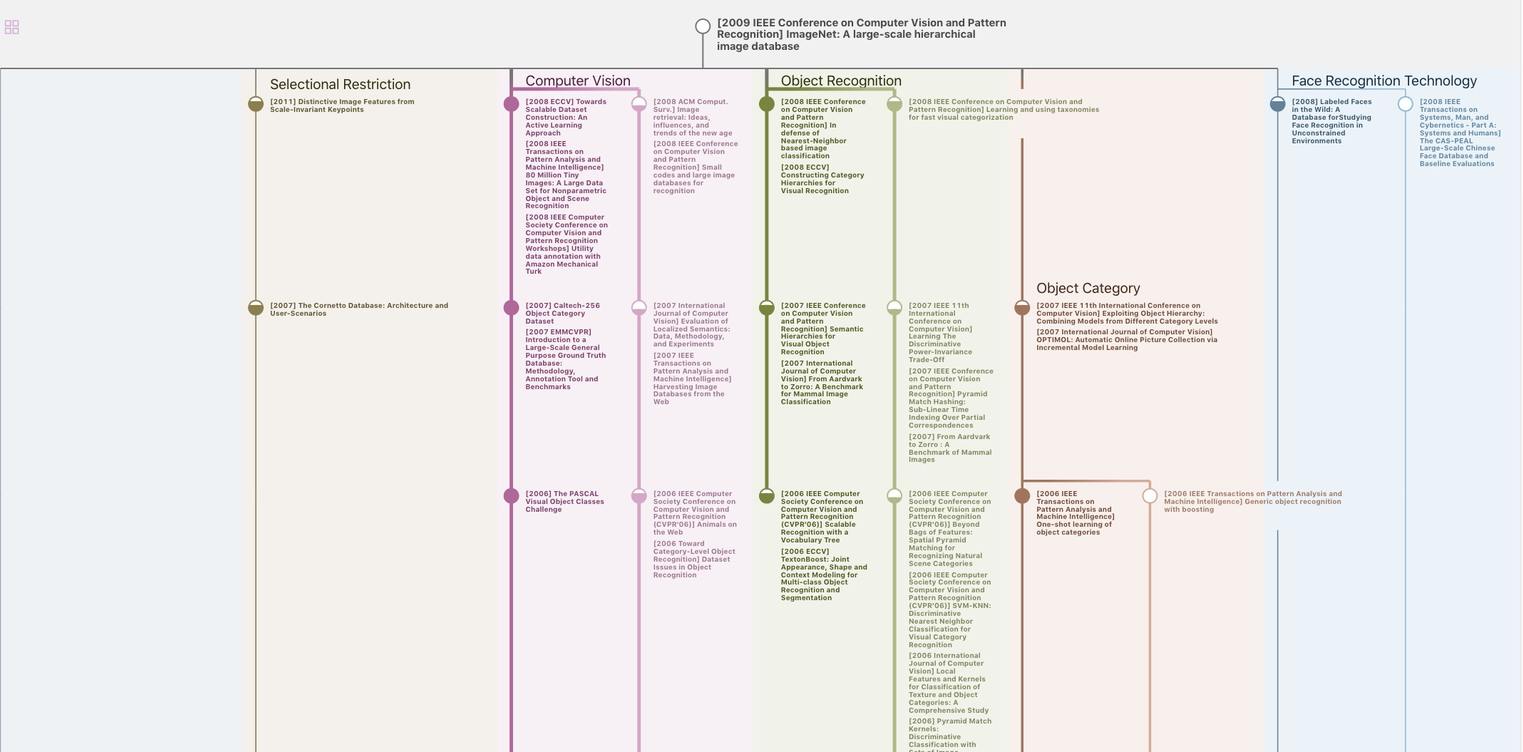
生成溯源树,研究论文发展脉络
Chat Paper
正在生成论文摘要