Data-Driven Virtual Reference Set-Point Learning of PD Control and Applications to Permanent Magnet Linear Motors
IEEE TRANSACTIONS ON SYSTEMS MAN CYBERNETICS-SYSTEMS(2023)
摘要
In this work, a data-driven virtual reference setting learning (DDVRSL) method is proposed to enhance the proportional-derivative (PD) feedback controller of the repetitive nonlinear system. First, an ideal nonlinear virtual reference setting learning law is presented in the outer loop of the control system to tune the reference setting. Such an ideal nonlinear learning law exists theoretically and is transferred to a linear parametric DDVRSL via iterative dynamic linearization (IDL). Next, an iterative adaptation law is proposed for the estimation of the parameters in the DDVRSL law subject to the nonlinear system which is also transferred into a linear form by using the IDL method. The iterative adaptation algorithm tunes the learning gains of DDVRSL law using input and output measurements, therefore improving the robust ability against uncertainties. The proposed DDVRSL-based PD control method does not require any exact mechanistic model knowledge. The convergence is proved via the contraction mapping principle, mathematical induction, and time-weighted norm. Further, the theoretical results are verified through simulations.
更多查看译文
关键词
Iterative methods,Control systems,Uncertainty,Process control,PD control,Feedback control,Convergence,Adaptive learning law,feedback controller,iterative learning control (ILC),reference setting learning,repetitive systems
AI 理解论文
溯源树
样例
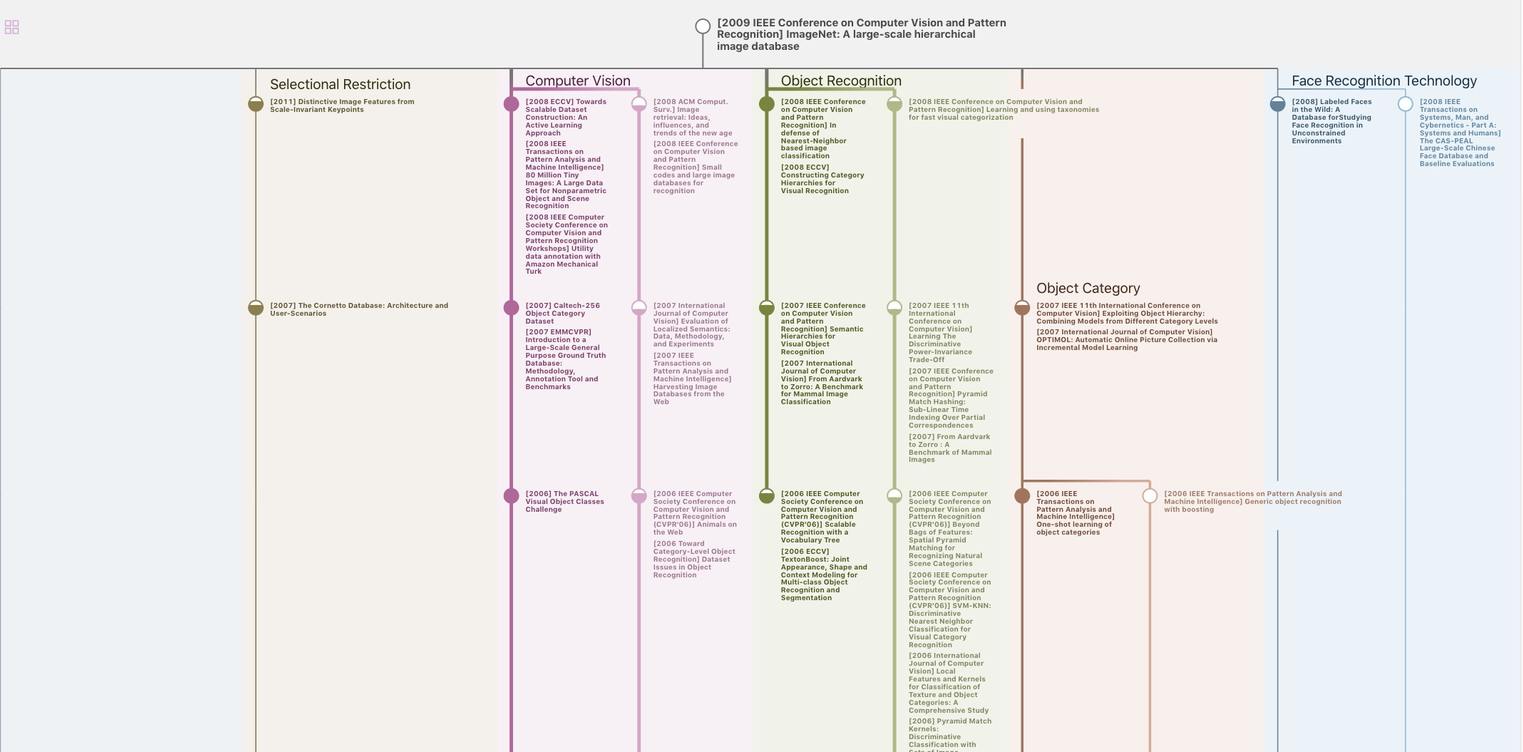
生成溯源树,研究论文发展脉络
Chat Paper
正在生成论文摘要