Uncertainty quantification and consideration in ML-aided traffic-driven service provisioning
COMPUTER COMMUNICATIONS(2023)
摘要
The network traffic prediction problem has been extensively studied in the literature through the application of statistical linear models and more recently through the application of machine learning (ML). In fact, ML has proven its capabilities on accurately modeling the non-linear nature of network traffic, outperforming conventional statistical linear models. Without doubt, model accuracy constitutes an important evaluation metric. In a network environment, however, where uncertainty may lead to erroneous service provisioning decisions (e.g., violations of the quality-of-service (QoS) requirements), it does not provide any information of how much the traffic predictions can be trusted. Hence, in this work the focus is on addressing traffic prediction uncertainty by leveraging the capabilities of Monte Carlo (MC) dropout inference. The proposed framework is compared with a margin-based technique, traditionally used to compensate for traffic prediction uncertainty, demonstrating that the MC dropout inference framework results in significant spectrum savings, as opposed to the baseline, myopic, margin-based scheme. Even though a small penalty is observed on the unpredictable traffic, this is successfully handled on-line with a reduced operational overhead compared to the case where prediction uncertainty is completely ignored. Importantly, it is shown that, unlike the margin-based framework, the MC dropout inference framework can be used for the provisioning of services with diverse QoS requirements.
更多查看译文
关键词
Traffic prediction,Uncertainty quantification,Margin reduction,Resource allocation,Optical networks
AI 理解论文
溯源树
样例
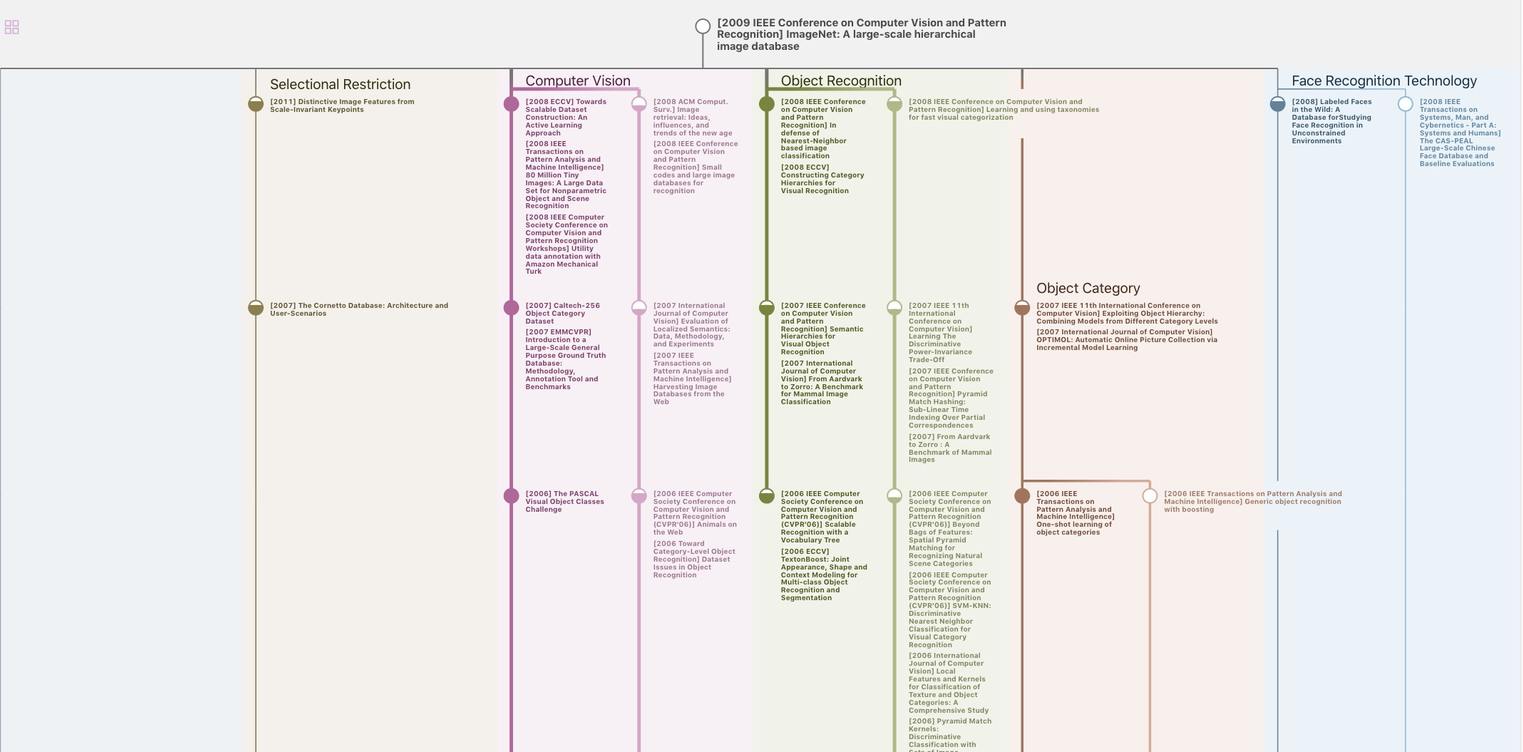
生成溯源树,研究论文发展脉络
Chat Paper
正在生成论文摘要