Simple deep-learning approach for alpha-decay half-life studies
PHYSICAL REVIEW C(2023)
摘要
To exploit the potential of deep learning (DL) in alpha-decay studies, we refocus on the essence of DL, which is a nonlinear input-output mapping with a hierarchical learning process. Here, instead of using the residual between experimental data and the calculation, the idea of training DL directly by experimental alpha-decay half-life is used. The Levenberg-Marquardt backpropagation algorithm is utilized for fixing free parameters of the mapping. A K-fold cross-validation method is introduced to avoid overfitting and improve the generalization performance of DL, as well as to determine hyperparameters effectively. We find that DL results with both three-dimensional {Z, A, Q(alpha)} and four-dimensional {Z, A, Q(alpha), l} input vectors achieve an impressive accuracy that matches or even exceeds the traditional models. Especially, DL realizes an efficient extraction of the pertinent shell and odd-even staggering effects of half-life solely from the characteristics of Q(alpha) values. On the other hand, the universal decay law (UDL) describes the classical relationship between {Z, A, Q(alpha)} and a-decay half-life, which is homologous with DL. Heuristically, we add the contributions of the angular momentum and blocking effect of unpaired nucleons in the UDL and develop an improved UDL formula.
更多查看译文
AI 理解论文
溯源树
样例
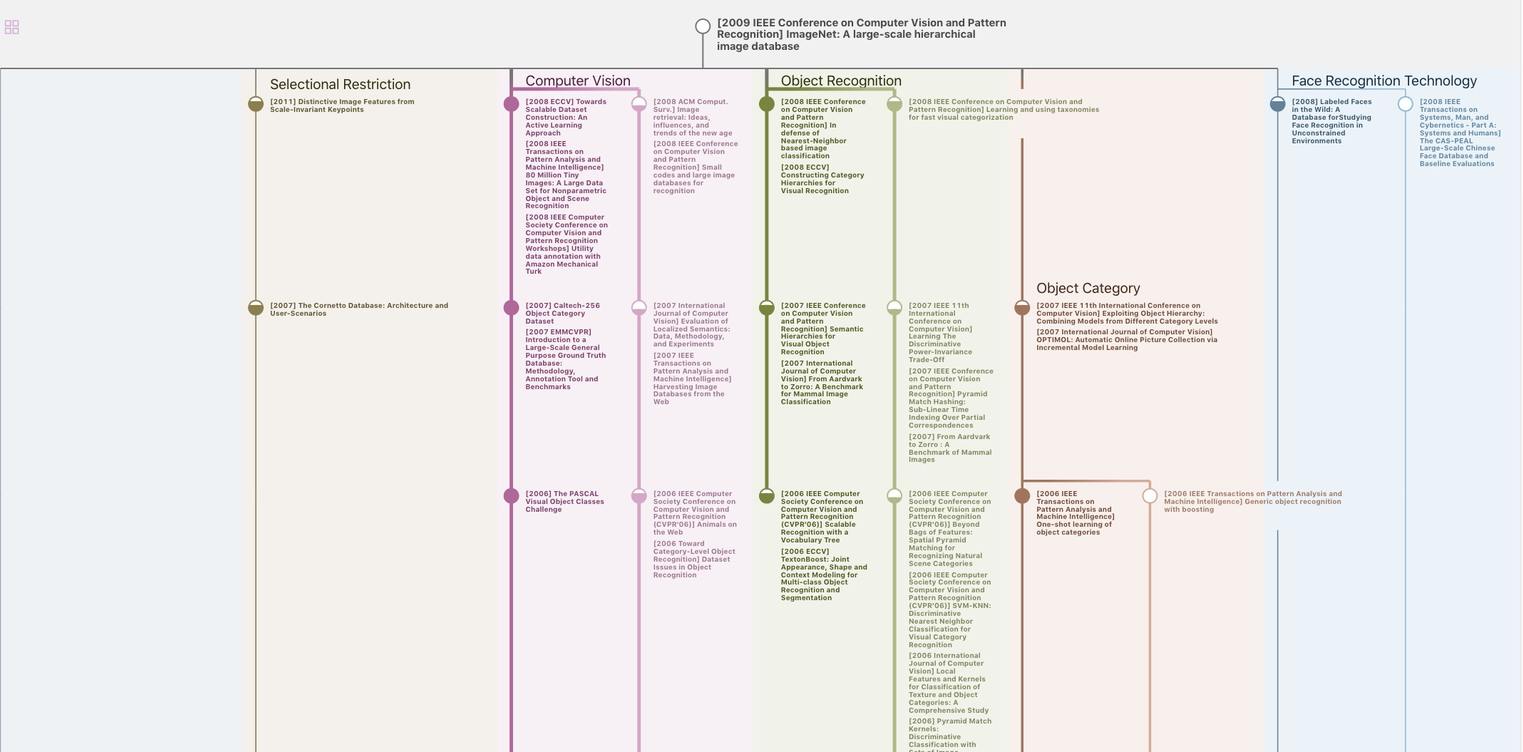
生成溯源树,研究论文发展脉络
Chat Paper
正在生成论文摘要