When Fairness Meets Consistency in AHP Pairwise Comparisons
MATHEMATICS(2023)
摘要
We propose introducing fairness constraints to one of the most famous multi-criteria decision-making methods, the analytic hierarchy process (AHP). We offer a solution that guarantees consistency while respecting legally binding fairness constraints in AHP pairwise comparison matrices. Through a synthetic experiment, we generate the comparison matrices of different sizes and ranges/levels of the initial parameters (i.e., consistency ratio and disparate impact). We optimize disparate impact for various combinations of these initial parameters and observed matrix sizes while respecting an acceptable level of consistency and minimizing deviations of pairwise comparison matrices (or their upper triangles) before and after the optimization. We use a metaheuristic genetic algorithm to set the dually motivating problem and operate a discrete optimization procedure (in connection with Saaty's 9-point scale). The results confirm the initial hypothesis (with 99.5% validity concerning 2800 optimization runs) that achieving fair ranking while respecting consistency in AHP pairwise comparison matrices (when comparing alternatives regarding given criterium) is possible, thus meeting two challenging goals simultaneously. This research contributes to the initiatives directed toward unbiased decision-making, either automated or algorithm-assisted (which is the case covered by this research).
更多查看译文
关键词
analytic hierarchy process (AHP),fairness,consistency,multi-criteria decision-making (MCDM),decision-making algorithms,discrete optimization,genetic algorithm (GA),fair ranking,pairwise comparison matrix (PCM)
AI 理解论文
溯源树
样例
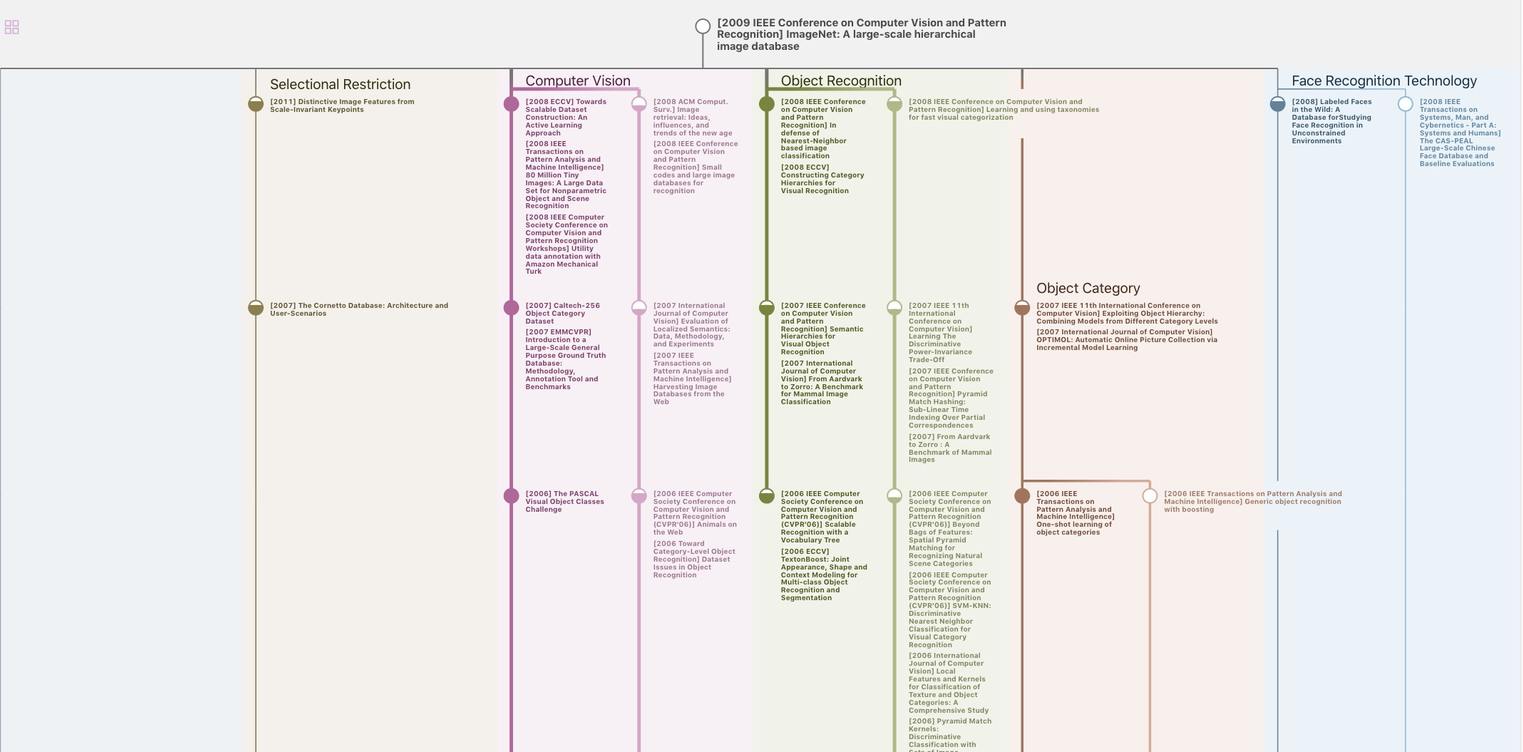
生成溯源树,研究论文发展脉络
Chat Paper
正在生成论文摘要