SSAE-KPLS: A quality-related process monitoring via integrating stacked sparse autoencoder with kernel partial least squares
CANADIAN JOURNAL OF CHEMICAL ENGINEERING(2023)
摘要
Kernel partial least squares (KPLS) is widely employed to address the issue of nonlinearity inherent in complex industrial processes. However, KPLS can only extract shallow features from process measurements. This paper proposes a new quality-related process monitoring method via integrating stacked sparse autoencoder (SSAE) with KPLS (SSAE-KPLS). First, an SSAE model is employed to exploit the nonlinearity within process variables. Through SSAE, hierarchical features are learned to extract latent representations of process variables from multiple sparse autoencoder (SAE) layers. Second, the learned hierarchical features from SSAE are used as input, and the final quality variables are used as output. A KPLS model is then built to exploit the nonlinear relationship between the hierarchical features extracted from process variables and the final product quality for process monitoring. Third, Hotelling's T-2 and Q statistics are employed to detect the quality-unrelated and quality-related faults, respectively. Finally, experiments on a numerical example and the commonly used industrial benchmark of the Tennessee Eastman process (TEP) are conducted to illustrate the efficacy and merits of the proposed SSAE-KPLS based quality-related process monitoring method by comparing it with other related methods.
更多查看译文
关键词
KPLS,process monitoring,quality-related,SSAE
AI 理解论文
溯源树
样例
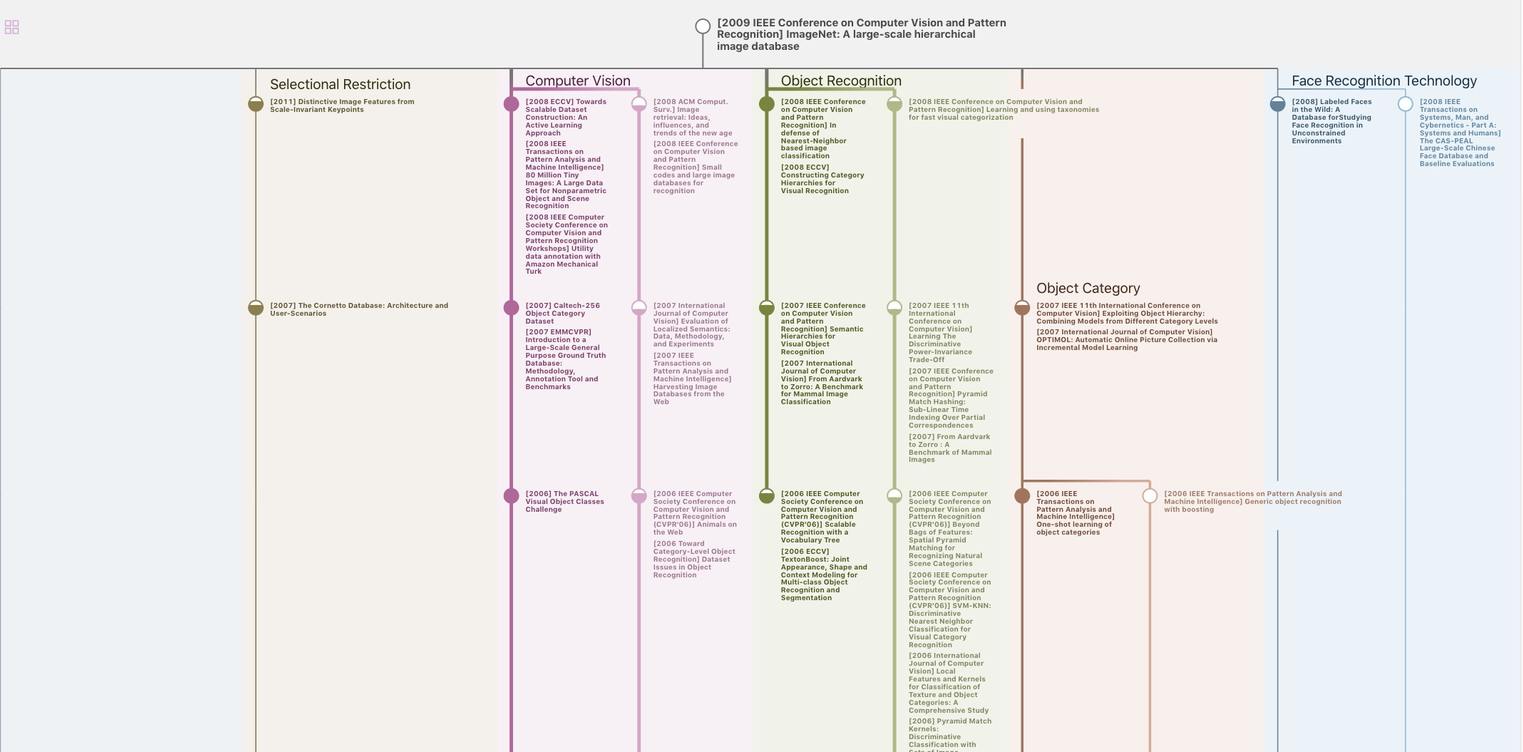
生成溯源树,研究论文发展脉络
Chat Paper
正在生成论文摘要