Predicting adherence to psychotherapy from smartphones using deep learning
AMERICAN JOURNAL OF GERIATRIC PSYCHIATRY(2023)
摘要
Introduction The effectiveness of psychotherapy strongly depends on patients’ adherence to homework (HW) which allows them to practice the skills needed for treatment. HW adherence also predicted decline in subjective and objective measures of depressive symptoms and correlates with short-term and long-term symptoms in anxiety disorders. HW completion rates are also correlated with clarity of instructions provided by the therapist and the time required to finish the homework. While the provider can give clear instructions and assign the patient a doable homework that could be done within a reasonable time frame, providers do not have the ability to consistently prompt clients to finish their homework in between treatment sessions. By comparison, mobile apps can offer continuing reminders and encouraging messages between sessions to encourage clients to complete their homework. However, too frequent prompts reminding patients to complete their HW can overwhelm or burden the patient, reduce the patient's engagement with the mHealth app and consequently be ineffective in improving HW adherence. The ability to predict when a patient is likely to complete HW is imperative to utilize mHealth technology in improving HW adherence. Methods Participants of three clinical trials conducted at the Weill Cornell ALACRITY Center undergoing psychotherapeutic interventions on middle-aged and older adults with depressive disorders were provided with a smartphone pre-installed with an mHealth app designed to collect daily active ratings, daily HW completion status and passively collect data recorded by the device sensors. This paper's goal is to build a predictive model of adherence to psychotherapy operationalized as provider assigned daily HW completion status. To this end, we include baseline demographics and clinical characteristics as predictors and construct predictors from active and passive data with a 7-day lookback period. We define mean, standard deviation, trends and number of peaks and valleys of predictors during this 7-day lookback period as our predictors. We then use various machine learning algorithms such as Random Forests, Gradient Boosting Machines, LASSO and Neural Networks to build prediction model of HW adherence using data on days participants respond to the HW. In addition, we implement a naïve semi-supervised deep learning algorithm that utilizes the predictors corresponding to non-response to HW prediction to estimate the predicted response and iteratively updates the prediction in a deep neural network. Finally, the prediction accuracy was estimated via a time-dependent cross-validation strategy that utilizes data from a participant up to a certain time-point in the study (say 3 weeks) to predict their outcomes in remaining duration of the study (from 3 to 9 weeks). Results There were 68 participants included in this study with a mean (SD) age of 71.4 (7.76) years, mean (SD) years of education of 14.9 (2.93) years, mean (SD) BIS/BAS total of 22.6 (3.36), mean (SD) MADRS total score of 20.4 (6.04) and a total of 3967 responses to the HW completion question combined over the period of the intervention. Four machine learning algorithms were used to predict HW adherence using baseline characteristics (b), daily active ratings (a) and passively collected data (p) and Random Forests produced the highest accuracy (83.2%) in the model which included all predictors (b+a+p). The prediction accuracy reduced to 80.9% when passive predictors were dropped from the model (b+a) but the accuracy was comparable in models without active predictors (b+p model; 82.8%), in the model without baseline predictors (p+a model; 82.5%) and in the model with passive predictors alone (p model; 82.3%). Including the data of predictors on days of non-response, (N=1935) to the HW completion question in our semi-supervised deep learning model, the prediction accuracy was 82% in the b+a+p model. This suggests that the prediction model is generalizable to predict HW completion status on days when a participant is likely not to respond. Conclusions This paper demonstrates the feasibility of predicting adherence to psychotherapy of depression in middle-aged and older adults using passively collected data by mHealth apps. Inclusion of such predictive models in the development of mHealth apps has the potential of increasing adherence to psychotherapy and thereby improving effectiveness of psychotherapies without increasing the notification burden of the user. This research was funded by NIMH P50MH113838 The effectiveness of psychotherapy strongly depends on patients’ adherence to homework (HW) which allows them to practice the skills needed for treatment. HW adherence also predicted decline in subjective and objective measures of depressive symptoms and correlates with short-term and long-term symptoms in anxiety disorders. HW completion rates are also correlated with clarity of instructions provided by the therapist and the time required to finish the homework. While the provider can give clear instructions and assign the patient a doable homework that could be done within a reasonable time frame, providers do not have the ability to consistently prompt clients to finish their homework in between treatment sessions. By comparison, mobile apps can offer continuing reminders and encouraging messages between sessions to encourage clients to complete their homework. However, too frequent prompts reminding patients to complete their HW can overwhelm or burden the patient, reduce the patient's engagement with the mHealth app and consequently be ineffective in improving HW adherence. The ability to predict when a patient is likely to complete HW is imperative to utilize mHealth technology in improving HW adherence. Participants of three clinical trials conducted at the Weill Cornell ALACRITY Center undergoing psychotherapeutic interventions on middle-aged and older adults with depressive disorders were provided with a smartphone pre-installed with an mHealth app designed to collect daily active ratings, daily HW completion status and passively collect data recorded by the device sensors. This paper's goal is to build a predictive model of adherence to psychotherapy operationalized as provider assigned daily HW completion status. To this end, we include baseline demographics and clinical characteristics as predictors and construct predictors from active and passive data with a 7-day lookback period. We define mean, standard deviation, trends and number of peaks and valleys of predictors during this 7-day lookback period as our predictors. We then use various machine learning algorithms such as Random Forests, Gradient Boosting Machines, LASSO and Neural Networks to build prediction model of HW adherence using data on days participants respond to the HW. In addition, we implement a naïve semi-supervised deep learning algorithm that utilizes the predictors corresponding to non-response to HW prediction to estimate the predicted response and iteratively updates the prediction in a deep neural network. Finally, the prediction accuracy was estimated via a time-dependent cross-validation strategy that utilizes data from a participant up to a certain time-point in the study (say 3 weeks) to predict their outcomes in remaining duration of the study (from 3 to 9 weeks). There were 68 participants included in this study with a mean (SD) age of 71.4 (7.76) years, mean (SD) years of education of 14.9 (2.93) years, mean (SD) BIS/BAS total of 22.6 (3.36), mean (SD) MADRS total score of 20.4 (6.04) and a total of 3967 responses to the HW completion question combined over the period of the intervention. Four machine learning algorithms were used to predict HW adherence using baseline characteristics (b), daily active ratings (a) and passively collected data (p) and Random Forests produced the highest accuracy (83.2%) in the model which included all predictors (b+a+p). The prediction accuracy reduced to 80.9% when passive predictors were dropped from the model (b+a) but the accuracy was comparable in models without active predictors (b+p model; 82.8%), in the model without baseline predictors (p+a model; 82.5%) and in the model with passive predictors alone (p model; 82.3%). Including the data of predictors on days of non-response, (N=1935) to the HW completion question in our semi-supervised deep learning model, the prediction accuracy was 82% in the b+a+p model. This suggests that the prediction model is generalizable to predict HW completion status on days when a participant is likely not to respond. This paper demonstrates the feasibility of predicting adherence to psychotherapy of depression in middle-aged and older adults using passively collected data by mHealth apps. Inclusion of such predictive models in the development of mHealth apps has the potential of increasing adherence to psychotherapy and thereby improving effectiveness of psychotherapies without increasing the notification burden of the user.
更多查看译文
关键词
adherence,psychotherapy,deep learning,smartphones
AI 理解论文
溯源树
样例
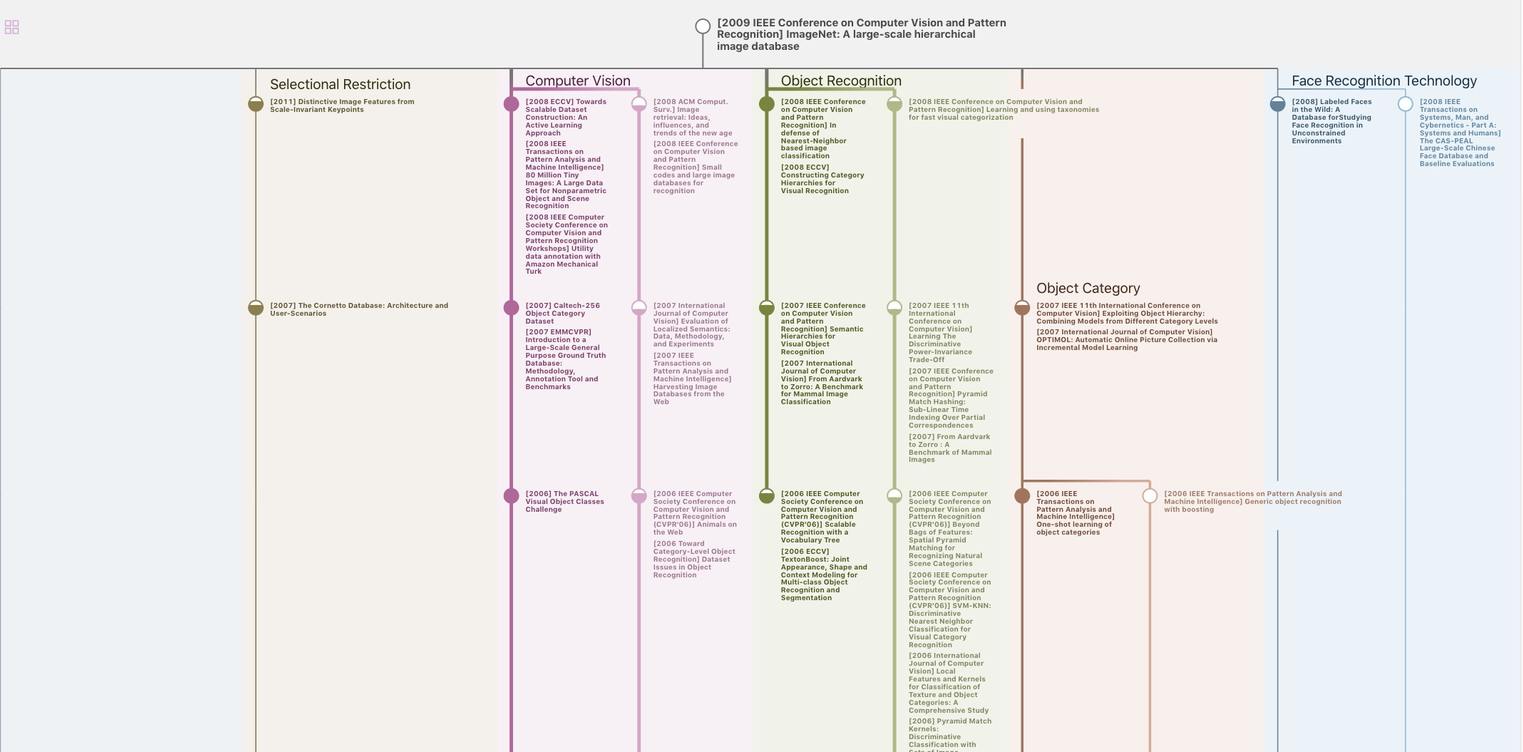
生成溯源树,研究论文发展脉络
Chat Paper
正在生成论文摘要