A Multi-Stage Deep Reinforcement Learning with Search-Based Optimization for Air-Ground Unmanned System Navigation
APPLIED SCIENCES-BASEL(2023)
摘要
An important challenge for air-ground unmanned systems achieving autonomy is navigation, which is essential for them to accomplish various tasks in unknown environments. This paper proposes an end-to-end framework for solving air-ground unmanned system navigation using deep reinforcement learning (DRL) while optimizing by using a priori information from search-based path planning methods, which we call search-based optimizing DRL (SO-DRL) for the air-ground unmanned system. SO-DRL enables agents, i.e., an unmanned aerial vehicle (UAV) or an unmanned ground vehicle (UGV) to move to a given target in a completely unknown environment using only Lidar, without additional mapping or global planning. Our framework is equipped with Deep Deterministic Policy Gradient (DDPG), an actor-critic-based reinforcement learning algorithm, to input the agents' state and laser scan measurements into the network and map them to continuous motion control. SO-DRL draws on current excellent search-based algorithms to demonstrate path planning and calculate rewards for its behavior. The demonstrated strategies are replayed in an experienced pool along with the autonomously trained strategies according to their priority. We use a multi-stage training approach based on course learning to train SO-DRL on the 3D simulator Gazebo and verify the robustness and success of the algorithm using new test environments for path planning in unknown environments. The experimental results show that SO-DRL can achieve faster algorithm convergence and a higher success rate. We piggybacked SO-DRL directly onto a real air-ground unmanned system, and SO-DRL can guide a UAV or UGV for navigation without adjusting any networks.
更多查看译文
关键词
air-ground unmanned system,deep reinforcement learning,deep deterministic policy gradient,navigation,path planning,obstacle avoidance
AI 理解论文
溯源树
样例
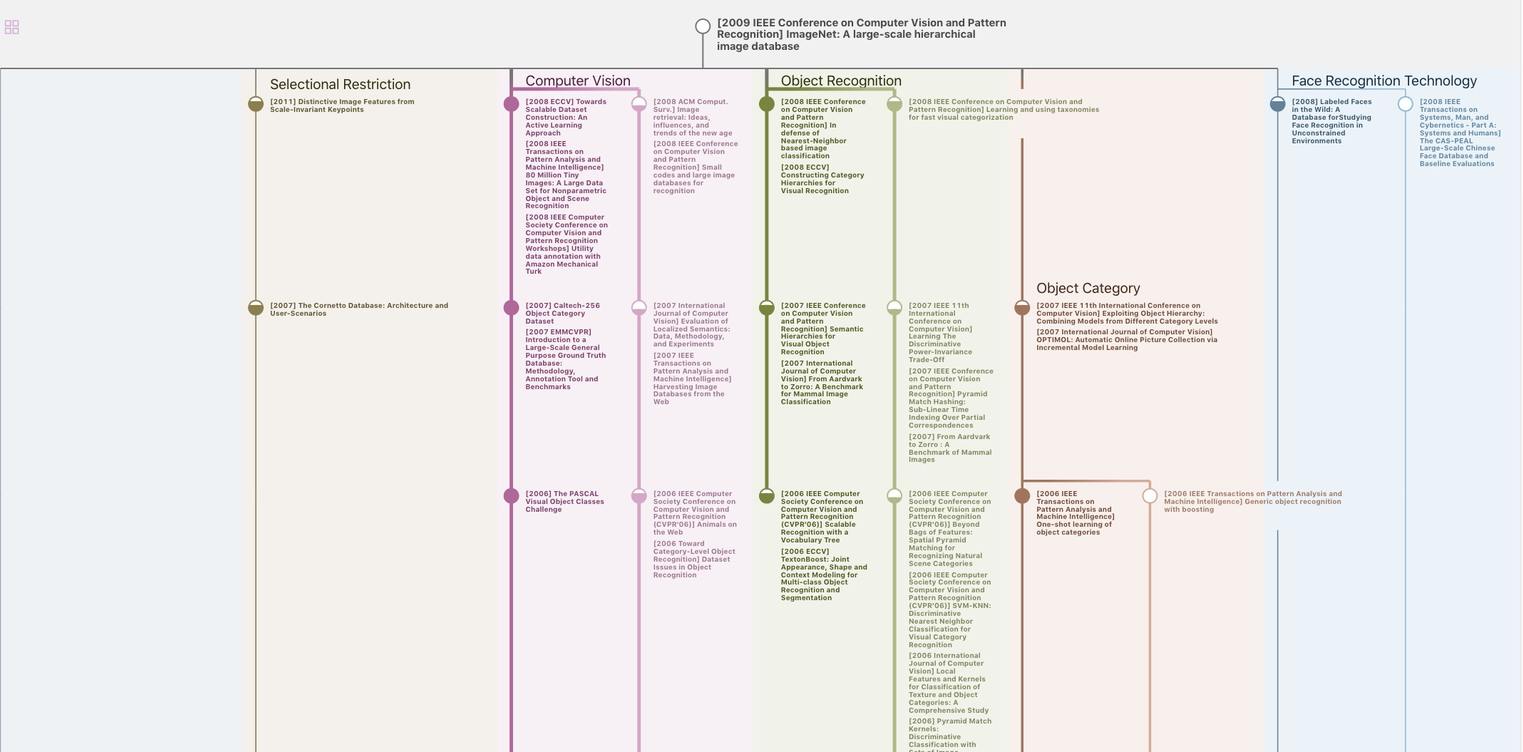
生成溯源树,研究论文发展脉络
Chat Paper
正在生成论文摘要