Augmenting Feature Representation with Gradient Penalty for Robust Text Categorization
INTERNATIONAL JOURNAL OF INTELLIGENT SYSTEMS(2023)
摘要
The capabilities of deep models are constantly mined for extraction and representation of features among text classification tasks. However, these models are sensitive to changes in input data, resulting in poor robustness. Meanwhile, the model lacks information interaction and weak representation ability. In this work, for feature extraction, a joint model that consists of a convolutional neural network, a bidirectional gated recurrent unit, and an attention mechanism is proposed. This new model can improve versatility and fully discover category information in text. For feature representation, a projector under the supervised contrastive learning method is introduced. The method can improve the representation of an encoder and realize aggregation of the same category. Considering the robustness of the PCRA, the gradient penalty is added to a contrastive loss function. Experiments are performed on four datasets to assess the proposed model (PCRA and PCRA-GP) using an accuracy metric. The experimental results show that our model is suitable for variable-length and bilingual texts. Compared with the baseline model, it remains competitive, and it reaches SOTA on the 20 Newsgroups dataset. Moreover, the performance of the model is evaluated under different hyperparameters to clarify its working mechanism.
更多查看译文
关键词
feature representation,gradient penalty,text
AI 理解论文
溯源树
样例
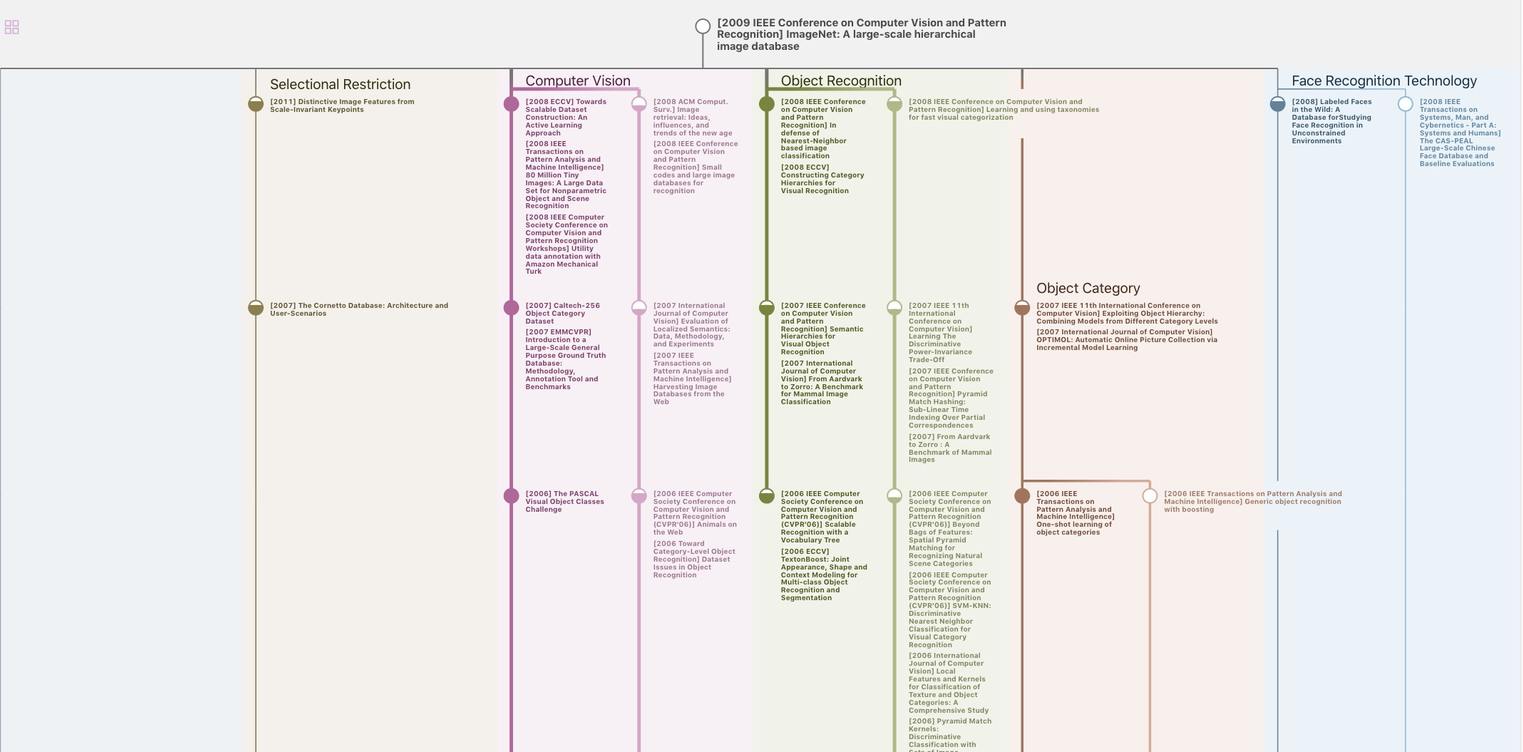
生成溯源树,研究论文发展脉络
Chat Paper
正在生成论文摘要