Physics-informed Transfer Learning for Out-of-sample Vapor Pressure Predictions
PROPELLANTS EXPLOSIVES PYROTECHNICS(2023)
摘要
Recent advances have enabled machine learning methodologies developed for large datasets to be applied to the small experimental datasets typically available for chemical systems. Such advances typically involve a data-based approach to transfer learning, where a portion of the experimental data for the property of interest is used to fine-tune a model that is pre-trained on computationally generated data. This transfer learning approach does not work for very small experimental datasets, where there are only enough data for model validation. Here, we develop a physics-informed transfer learning strategy to train a directed-message passing neural network (D-MPNN) model, enabling extrapolation outside of the training domain. We demonstrate this approach by training a D-MPNN model on interpolated vapor pressures and validate the model on an out-of-sample test set of energetic molecule vapor pressures, achieving accuracy comparable to those of experiments.
更多查看译文
关键词
Machine Learning,Transfer Learning,Energetic Materials
AI 理解论文
溯源树
样例
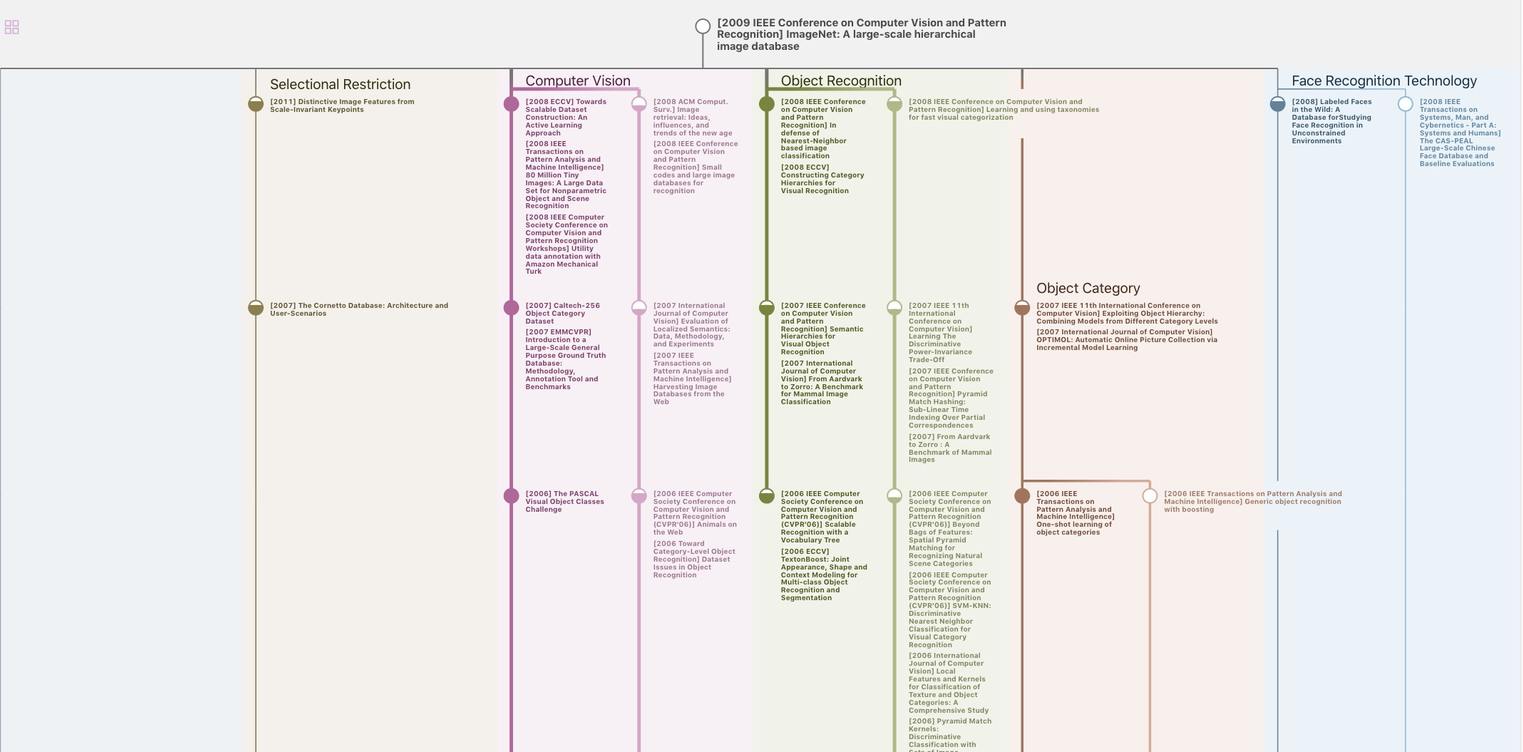
生成溯源树,研究论文发展脉络
Chat Paper
正在生成论文摘要