Research on Named Entity Recognition for Spoken Language Understanding Using Adversarial Transfer Learning
ELECTRONICS(2023)
摘要
In this paper, we propose an adversarial transfer learning method to solve the lack of data resources for named entity recognition (NER) tasks in spoken language understanding. In the framework, we use bi-directional long short-term memory with self-attention and conditional random field (BiLSTM-Attention-CRF) model which combines character and word information as the baseline model to train source domain and target domain corpus jointly. Shared features between domains are extracted by a shared feature extractor. This paper uses two different sharing patterns simultaneously: full sharing mode and private sharing mode. On this basis, an adversarial discriminator is added to the shared feature extractor to simulate generative adversarial networks (GAN) and eliminate domain-dependent features. This paper compares ordinary adversarial discriminator (OAD) and generalized resource-adversarial discriminator (GRAD) through experiments. The experimental results show that the transfer effect of GRAD is better than other methods. The F1 score reaches 92.99% at the highest, with a relative increase of 12.89%. It can effectively improve the performance of NER tasks in resource shortage fields and solve the problem of negative transfer.
更多查看译文
关键词
spoken language understanding,named entity recognition,transfer learning,adversarial discriminator
AI 理解论文
溯源树
样例
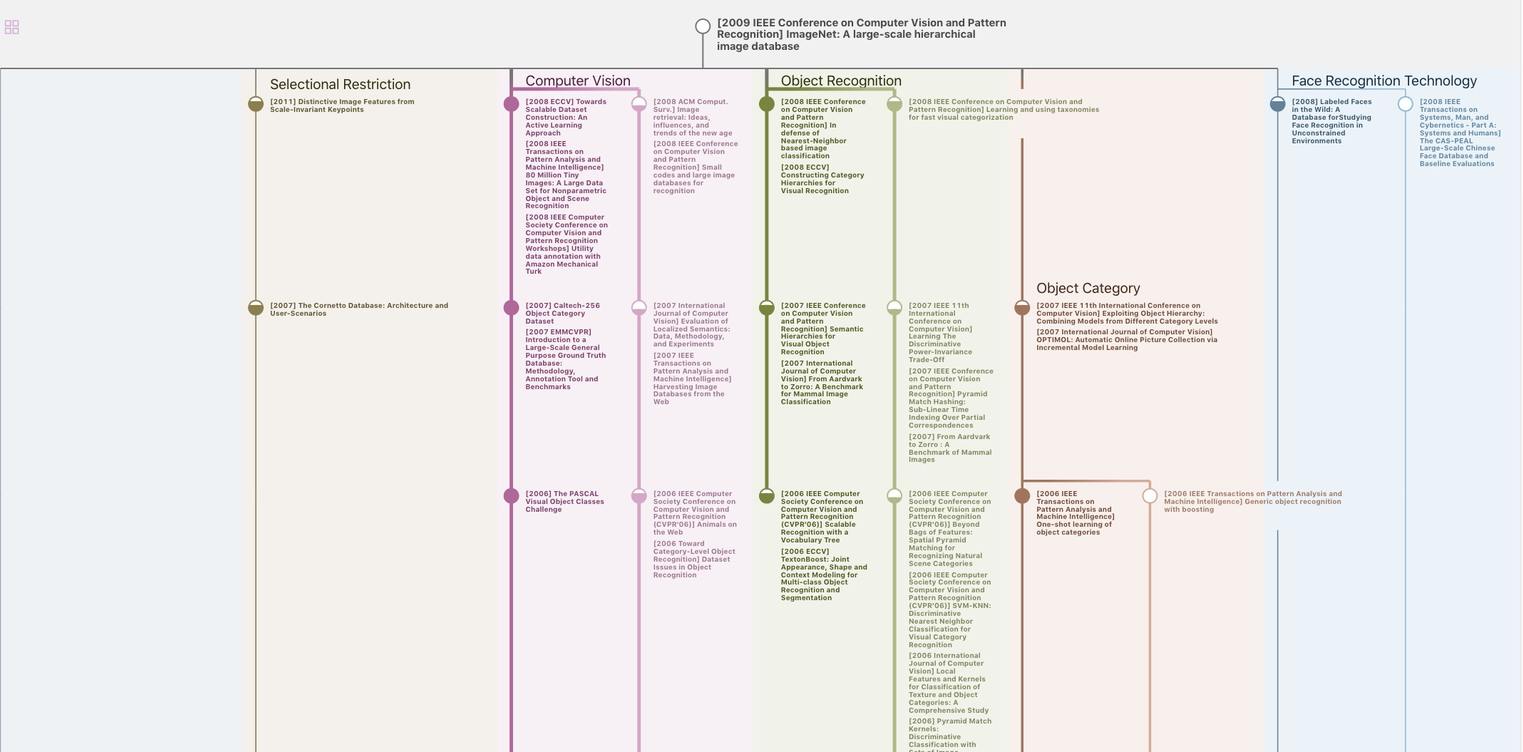
生成溯源树,研究论文发展脉络
Chat Paper
正在生成论文摘要