Assessment of impaired consciousness using EEG-based connectivity features and convolutional neural networks
COGNITIVE NEURODYNAMICS(2023)
摘要
Growing electroencephalogram (EEG) studies have linked the abnormities of functional brain networks with disorders of consciousness (DOC). However, due to network data’s high-dimensional and non-Euclidean properties, it is difficult to exploit the brain connectivity information that can effectively detect the consciousness levels of DOC patients via deep learning. To take maximum advantage of network information in assessing impaired consciousness, we utilized the functional connectivity with convolutional neural network (CNN) and employed three rearrangement schemes to improve the evaluation performance of brain networks. In addition, the gradient-weighted class activation mapping (Grad-CAM) was adopted to visualize the classification contributions of connections among different areas. We demonstrated that the classification performance was significantly enhanced by applying network rearrangement techniques compared to those obtained by the original connectivity matrix (with an accuracy of 75.0%). The highest classification accuracy (87.2%) was achieved by rearranging the alpha network based on the anatomical regions. The inter-region connections (i.e., frontal-parietal and frontal-occipital connectivity) played dominant roles in the classification of patients with different consciousness states. The effectiveness of functional connectivity in revealing individual differences in brain activity was further validated by the correlation between behavioral performance and connections among specific regions. These findings suggest that our proposed assessment model could detect the residual consciousness of patients.
更多查看译文
关键词
Convolutional neural network (CNN),Disorders of consciousness (DOC),Electroencephalogram (EEG),Brain network
AI 理解论文
溯源树
样例
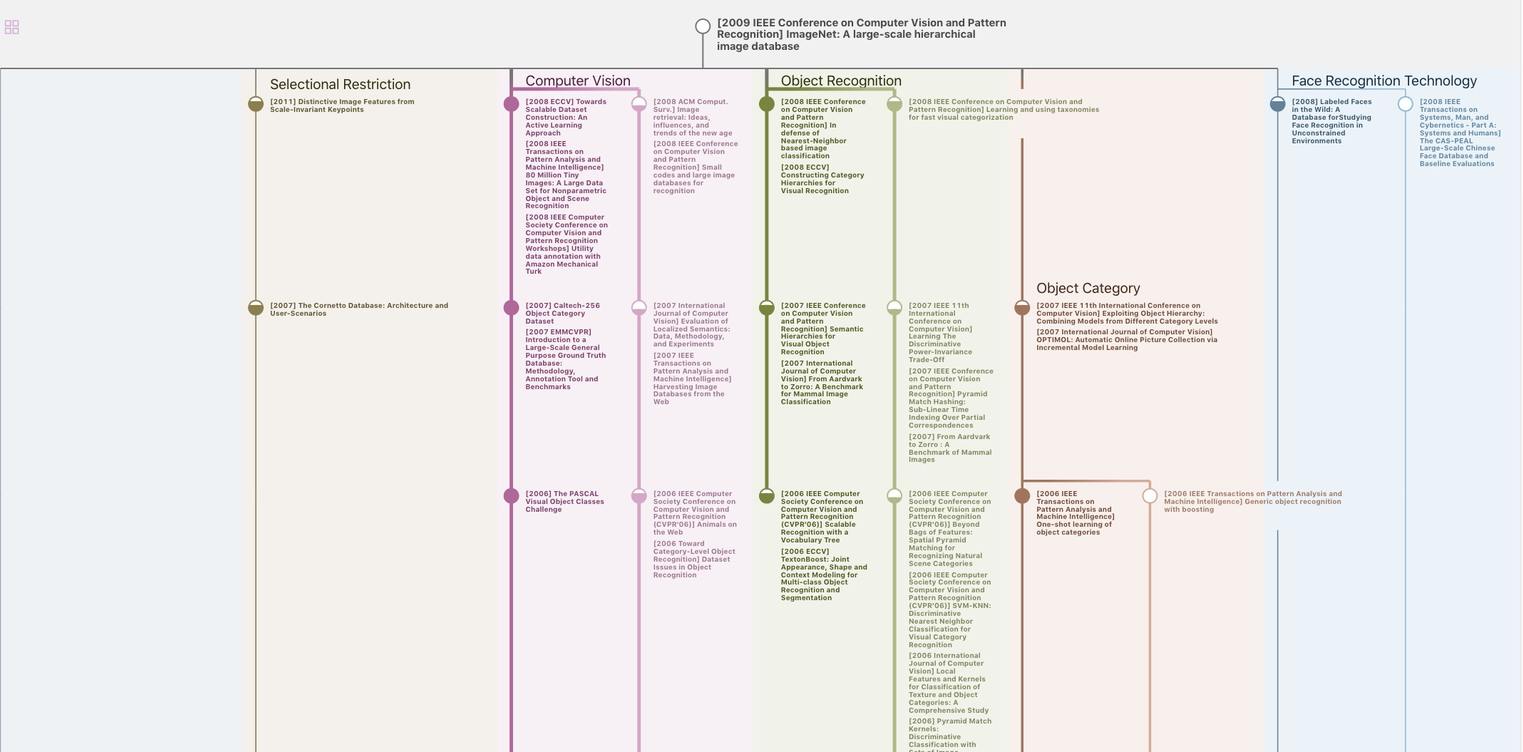
生成溯源树,研究论文发展脉络
Chat Paper
正在生成论文摘要