Crowd Emotion Prediction for Human-Vehicle Interaction Through Modified Transfer Learning and Fuzzy Logic Ranking
IEEE TRANSACTIONS ON INTELLIGENT TRANSPORTATION SYSTEMS(2023)
摘要
In metropolitan environments, unmanned aerial vehicles (UAVs) equipped with video surveillance equipment can monitor crowd behavior and maintain public safety. In high-traffic areas where humans are more likely to make mistakes, a smart city needs modern technology to forecast the behavior of its residents. In order to improve citywide traffic flow, urban transportation systems (UTSs) monitor and learn how people behave in crowds. Using UAVs for video surveillance in smart cities, our research describes a unique way to assess crowd condition, which expands the scope of human-vehicle interactions. Moreover, we use fuzzy logic ranking to improve the system's ability to detect anomalies in crowds. In order to improve decision-making, a novel deep transfer learning (DTL) technique is applied to the UAV's received frames. A 98.5% accuracy rate, satisfactory performance, and robustness to population behavior are all characteristics of the proposed integrated model. In UTSs and urban areas, our novel intelligent system analyzes human behavior based on vehicle-human interactions. In areas with low and high traffic congestion, the modified ResNet (mResNet) architecture predicts the crowd's condition based on fuzzy logic (FLA). Through decision-making based on accurate crowd conditions, best general paths can be selected using the algorithm.
更多查看译文
关键词
Behavioral sciences,Monitoring,Decision making,Feature extraction,Convolution,Transfer learning,Smart cities,Smart environment,urban transportation system,crowd emotion prediction,human-vehicle interaction,deep transfer learning,fuzzy logic approach
AI 理解论文
溯源树
样例
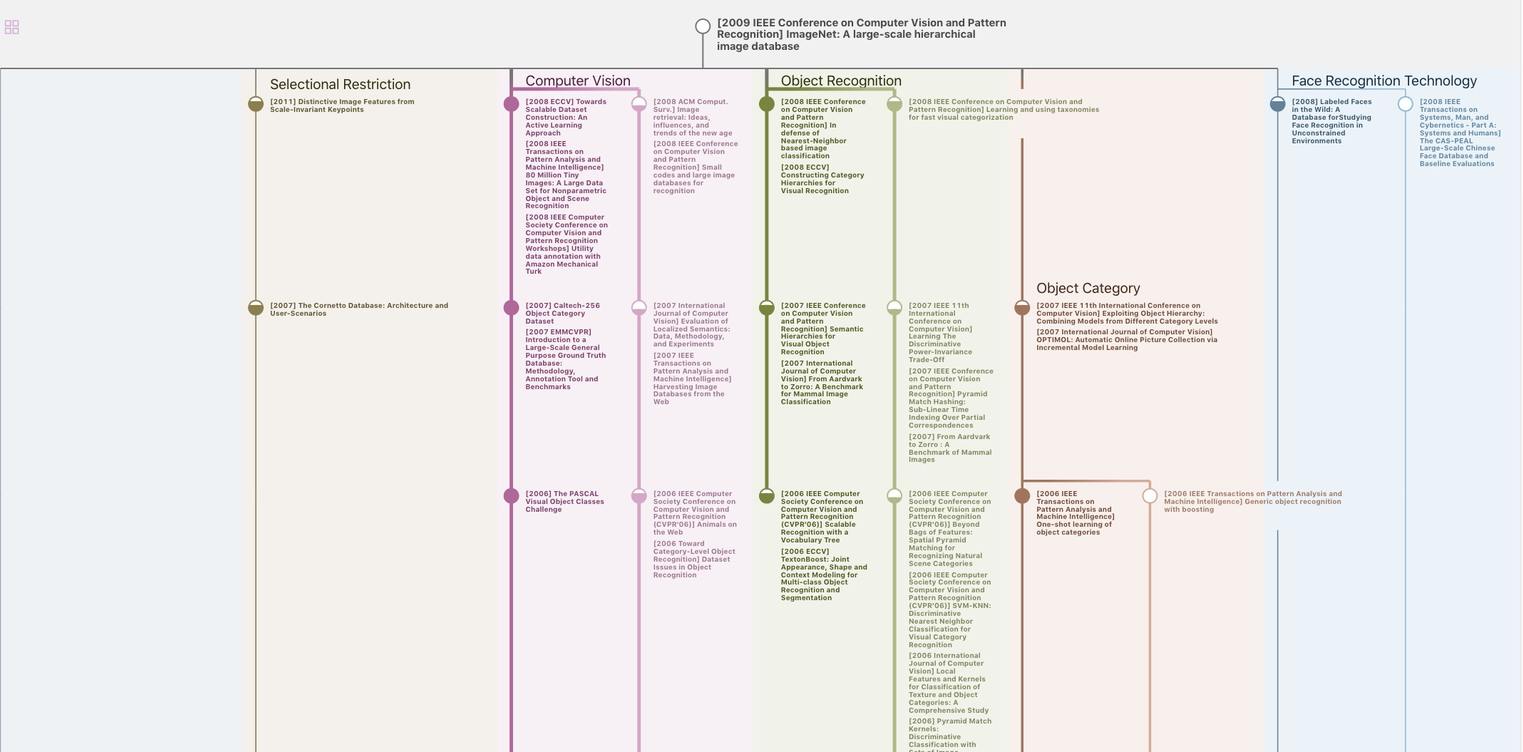
生成溯源树,研究论文发展脉络
Chat Paper
正在生成论文摘要