A Visual SLAM Approach to Deeply Explore the Spatial Constraints in Indoor Environment Based on the Manhattan Hypothesis
JOURNAL OF SENSORS(2023)
摘要
It is a major challenge for a visual SLAM system to maintain a highly precise and robust mapping and localization ability in an environment with low texture. In this paper, a bundle adjustment method for deeply exploring the spatial constraints based on the Manhattan hypothesis is proposed. First, an energy function for filtering the feature set and segmenting the point cloud is given, and a semantic graph is formed with the minimum energy. Then, a novel factor graph for back-end bundle adjustment is designed to include points, edges, planes, and spatial constraints to improve the stability and positioning accuracy of the system. After that, the corresponding error items of those spatial constraints for bundle adjustment are designed according to the Manhattan hypothesis. Finally, comparison tests are designed to evaluate the performance of the given approach using TUM RGB-D datasets in different scenes. Test results show that the proposed method provides better positioning ability, with the positioning accuracy improved by 18.07% on average compared with that of existing mainstream approaches.
更多查看译文
关键词
visual slam approach,spatial constraints,indoor environment
AI 理解论文
溯源树
样例
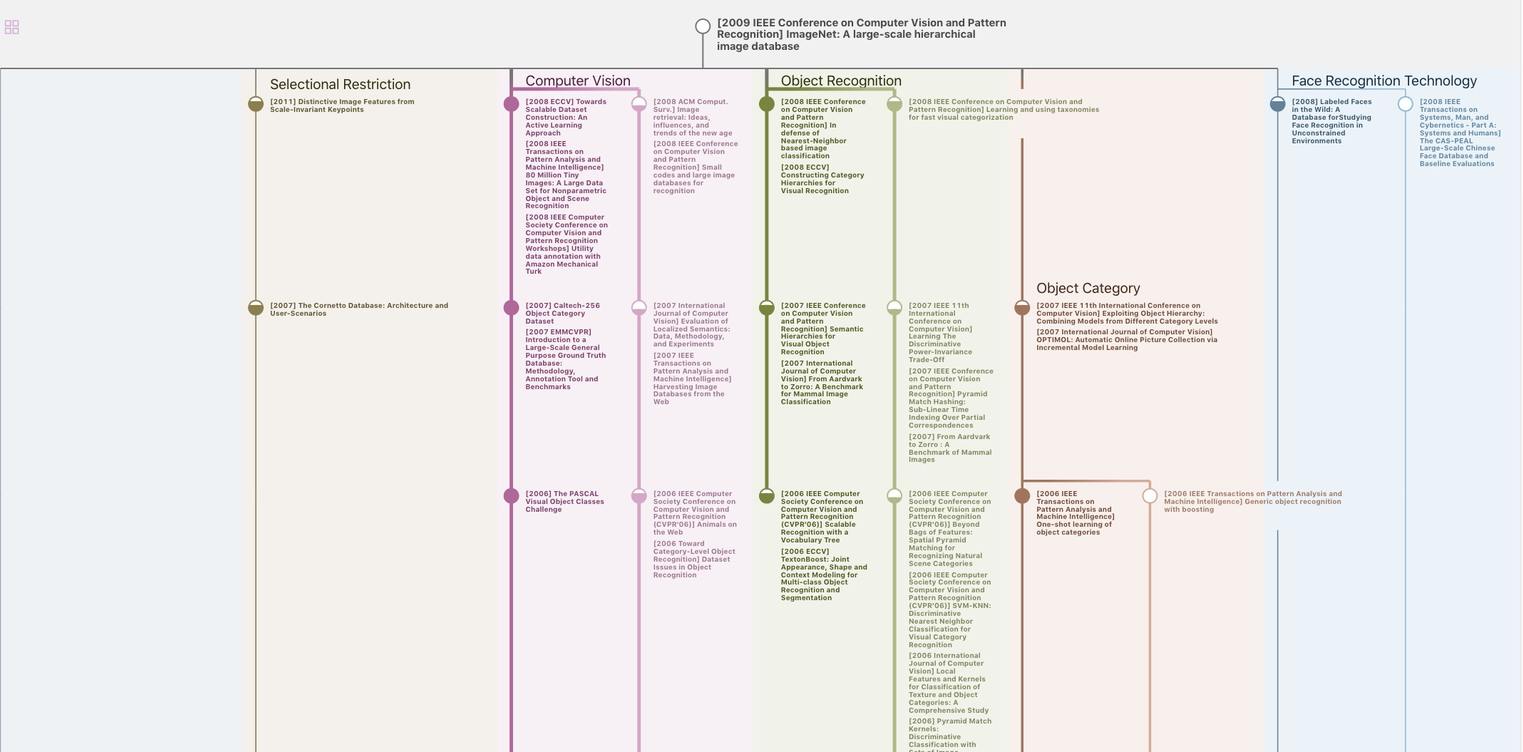
生成溯源树,研究论文发展脉络
Chat Paper
正在生成论文摘要