Fault detection method based on adversarial reinforcement learning
FRONTIERS IN COMPUTER SCIENCE(2023)
摘要
Fault detection is an essential task for large-scale industrial maintenance. However, in practical applications, due to the possible harm caused by the collection of fault data, the fault samples that lead to the labeling are usually very few. Most existing methods consider training unsupervised models with a large amount of unlabeled data while ignoring the rich knowledge that existed in a small amount of labeled data. To make full use of this prior knowledge, this article proposes a reinforcement learning model, namely, adversarial reinforcement learning in weakly supervised (WS-ARL), which performs significantly better by jointly learning small labeled anomaly data and large unlabeled data. We use an agent of the reinforcement learning model as a fault detector and add a new environment agent as a sample selector, by providing an opposite reward for two agents, and they learn in an adversarial environment. The feasibility and effectiveness of the model are verified by experimental analysis and compared the performance of the model with five state-of-the-art weakly/un-supervised methods in the hydraulic press fault detection task.
更多查看译文
关键词
fault detection,weakly supervised,reinforcement learning,neural network,agent,reward,strategy function,DQN
AI 理解论文
溯源树
样例
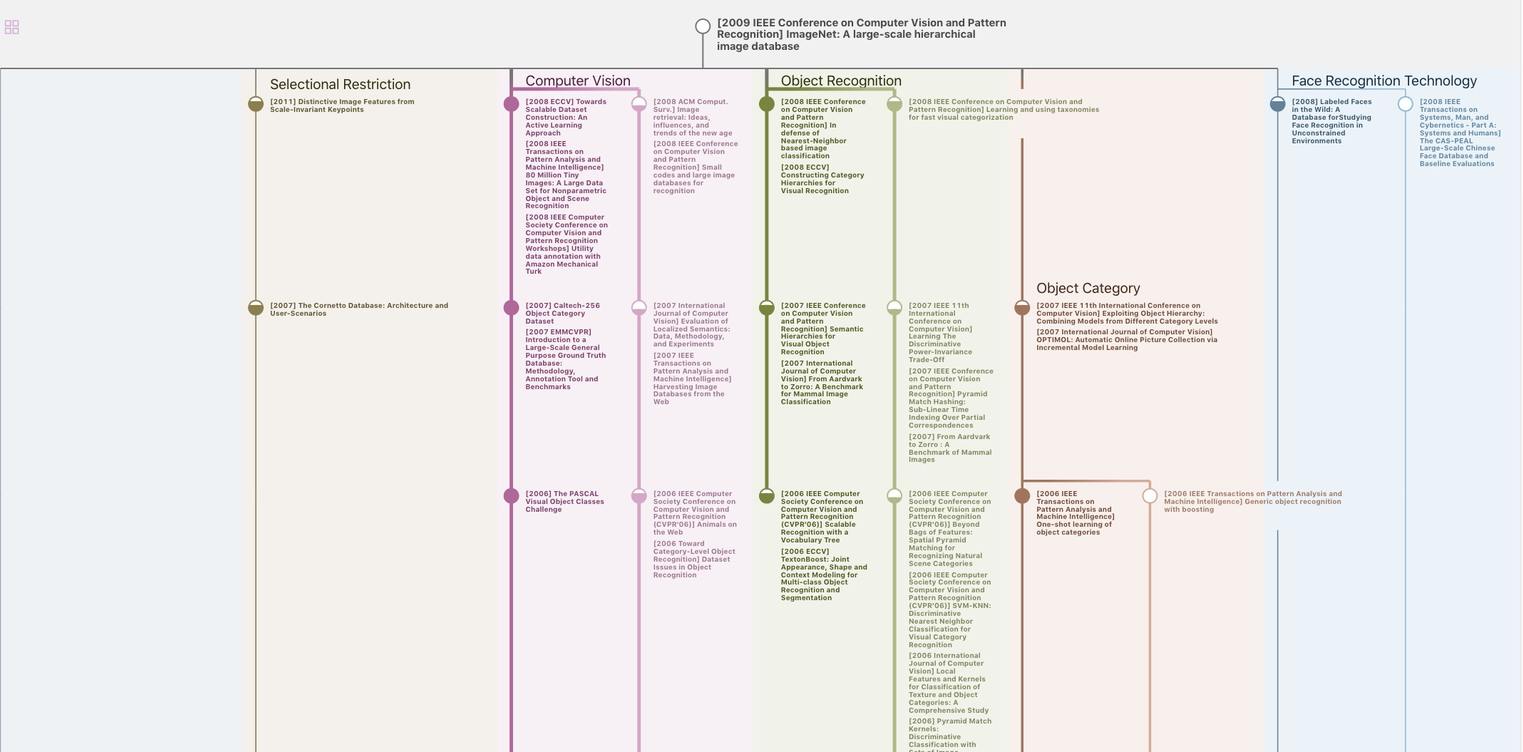
生成溯源树,研究论文发展脉络
Chat Paper
正在生成论文摘要