Robust Near-optimal Control for Constrained Nonlinear System via Integral Reinforcement Learning
INTERNATIONAL JOURNAL OF CONTROL AUTOMATION AND SYSTEMS(2023)
摘要
This paper proposes a robust near-optimal control algorithm for uncertain nonlinear systems with state constraints and input saturation. By incorporating a barrier function and a non-quadratic term, the robust stabilization problem with constraints and uncertainties is converted into an unconstrained optimal control problem of the nominal system, which requires the solution of the Hamilton-Jacobi-Bellman (HJB) equation. The proposed integral reinforcement learning (IRL)-based method can obtain the approximate solution of the HJB equation without requiring any knowledge of system drift dynamics. An online gain-adjustable update law of the actor-critic architecture is developed to relax the persistence of excitation (PE) condition and ensure the closed-loop system stability throughout learning. The uniform ultimate boundedness of the closed-loop system is verified using Lyapunov’s direct method. Simulation results demonstrate the effectiveness and feasibility of the proposed method.
更多查看译文
关键词
Constrained nonlinear system,integral reinforcement learning,optimal control,robust control
AI 理解论文
溯源树
样例
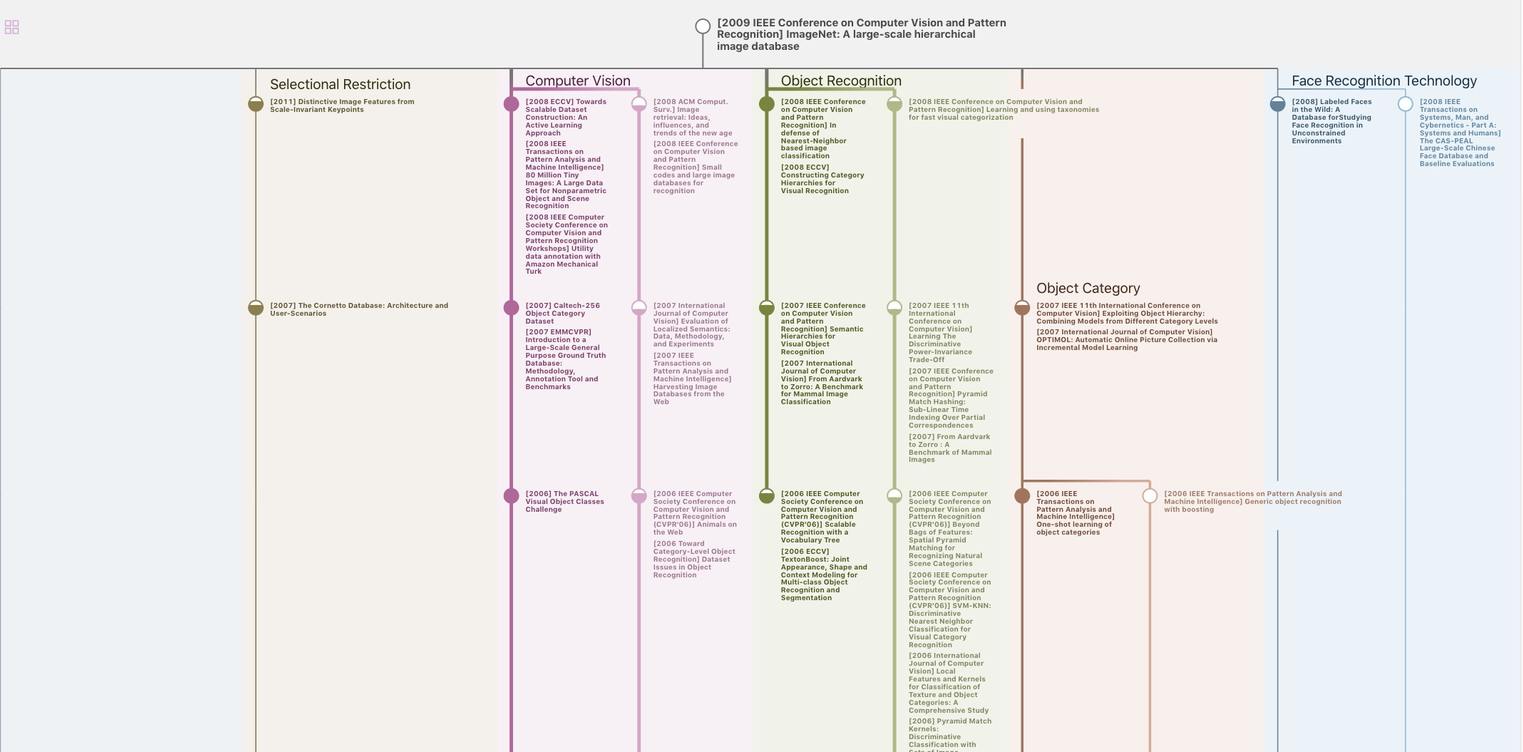
生成溯源树,研究论文发展脉络
Chat Paper
正在生成论文摘要