A Fast Kernel-Based Optimal Control Framework for Autonomous Driving
IEEE TRANSACTIONS ON CONTROL SYSTEMS TECHNOLOGY(2023)
摘要
A novel, promising strategy to improve path-tracking performance for autonomous driving is to combine a nominal model with an additive residual model. The residual part is identified by a kernel method Gaussian process (GP) regression from continuous data streaming. However, the high computational complexity of simultaneously using both numerical optimization and supervised learning is a critical challenge for applications with fast dynamics. This also limits the generalization capability in untrained circumstance. To address these issues, we design a feedforward compensator to handle the stochastic GP model. This allows an analytical solution to optimize the formulation, and therefore greatly lowers the computational burden. Our experimental results show that the computational time was reduced by 40 ms in comparison to the classical general-source solver. Consequently, the learning part can now track 19% more data points in one control step, saving more computation power to be allocated to prediction. In addition, we optimize the prediction dimension and apply the local technology to cluster intractable dictionary. Together, these improvements allow our fast kernel-based optimal control (FKB-OPT) framework to exhibit superior performance under inexperienced trajectories in the road tests.
更多查看译文
关键词
Computational modeling,Data models,Mathematical models,Optimization,Optimal control,Numerical models,Uncertainty,Learning-based control,model learning,path-tracking
AI 理解论文
溯源树
样例
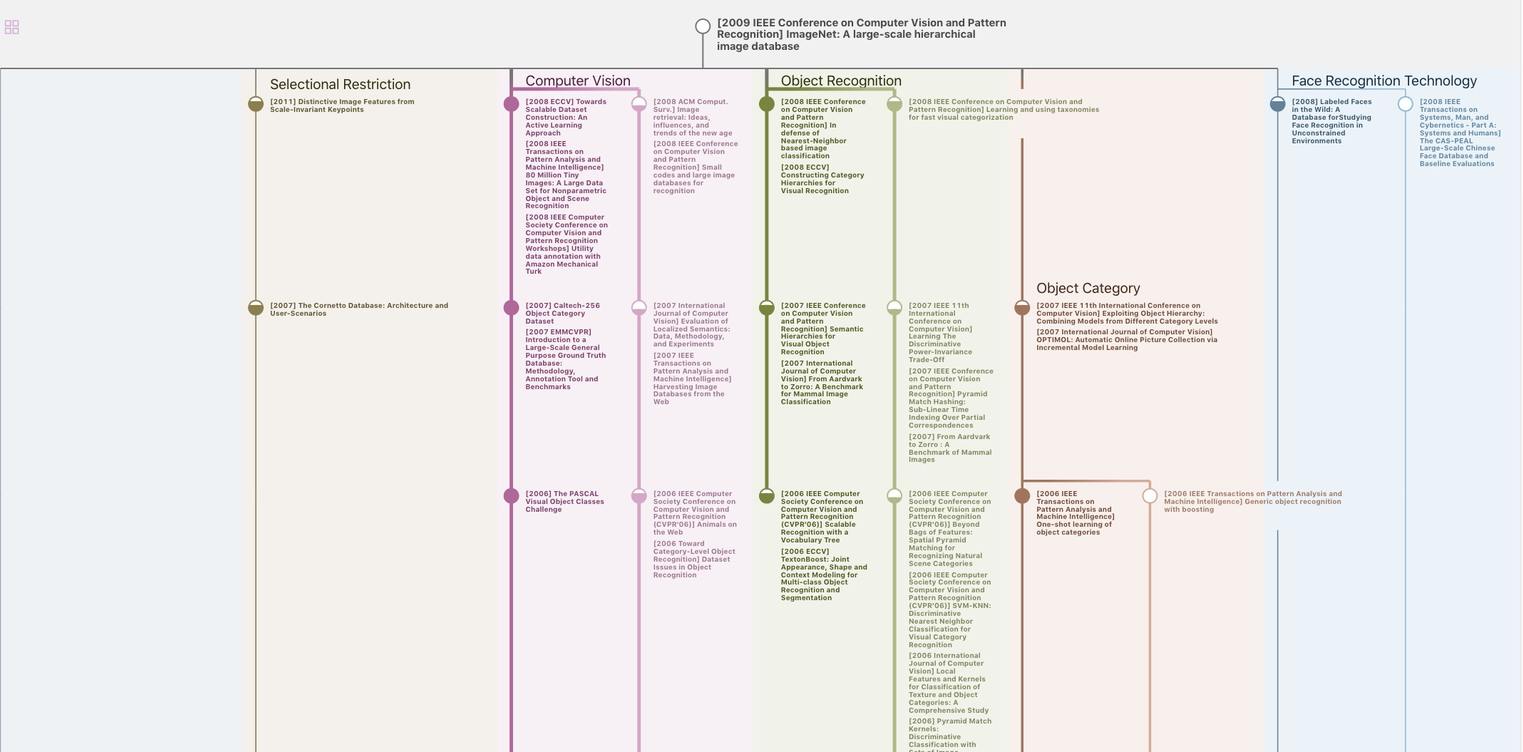
生成溯源树,研究论文发展脉络
Chat Paper
正在生成论文摘要